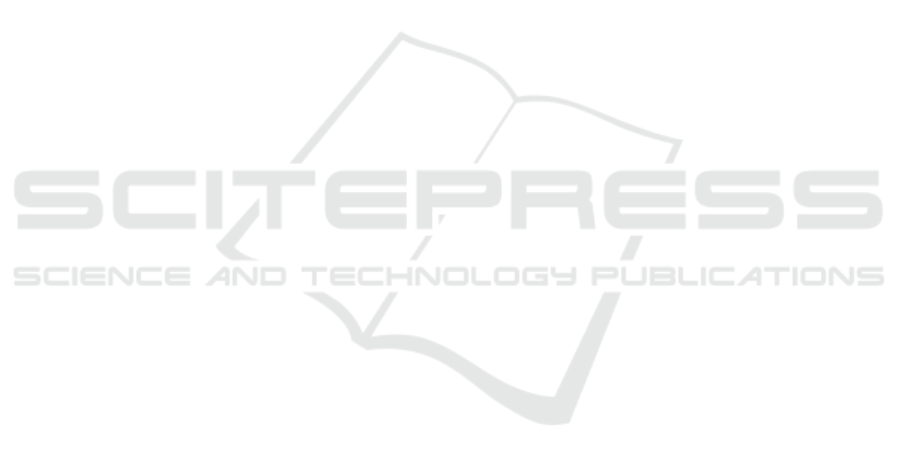
covery: a knowledge graph perspective. Briefings in
Bioinformatics, 23(6). bbac404.
Cizeau, P., Potters, M., and Bouchaud, J.-P. (2001). Corre-
lation structure of extreme stock returns. Quantitative
Finance, 1(2):217–222.
Gawehn, E., Hiss, J. A., and Schneider, G. (2016). Deep
learning in drug discovery. Molecular Informatics,
35(1):3–14.
Gomez, S., Hennart, J.-P., and Powell, M. J. D., editors
(1994). A Direct Search Optimization Method That
Models the Objective and Constraint Functions by
Linear Interpolation, pages 51–67. Springer Nether-
lands, Dordrecht.
Griewank, A. O. (1981). Generalized descent for global
optimization. J. Optim. Theory Appl., 34(1):11–39.
Huang, Y., Lei, H., and Li, X. (2020). An empirical study
of optimizers for quantum machine learning. In 2020
IEEE 6th International Conference on Computer and
Communications (ICCC), pages 1560–1566.
H
¨
ase, F., Aldeghi, M., Hickman, R. J., Roch, L. M., Chris-
tensen, M., Liles, E., Hein, J. E., and Aspuru-Guzik,
A. (2021). Olympus: a benchmarking framework for
noisy optimization and experiment planning. Machine
Learning: Science and Technology, 2(3):035021.
Joshi, N., Katyayan, P., and Ahmed, S. A. (2021). Eval-
uating the performance of some local optimizers for
variational quantum classifiers. Journal of Physics:
Conference Series, 1817(1):012015.
Khuri, A. I. and Mukhopadhyay, S. (2010). Response sur-
face methodology. WIREs Computational Statistics,
2(2):128–149.
Kingma, D. P. and Ba, J. (2014). Adam: A method for
stochastic optimization.
K
¨
olle., M., Giovagnoli., A., Stein., J., Mansky., M., Hager.,
J., and Linnhoff-Popien., C. (2023). Improving con-
vergence for quantum variational classifiers using
weight re-mapping. In Proceedings of the 15th In-
ternational Conference on Agents and Artificial In-
telligence - Volume 2: ICAART,, pages 251–258. IN-
STICC, SciTePress.
Lowe, A., Medvidovi
´
c, M., Hayes, A., O’Riordan, L. J.,
Bromley, T. R., Arrazola, J. M., and Killoran, N.
(2023). Fast quantum circuit cutting with randomized
measurements. Quantum, 7:934.
Ma, J., Sheridan, R. P., Liaw, A., Dahl, G. E., and Svet-
nik, V. (2015). Deep neural nets as a method for
quantitative structure–activity relationships. Journal
of Chemical Information and Modeling, 55(2):263–
274. PMID: 25635324.
Mantri, A., Demarie, T. F., and Fitzsimons, J. F. (2017).
Universality of quantum computation with cluster
states and (x, y)-plane measurements. Scientific Re-
ports, 7(1):42861.
McBride, K. and Sundmacher, K. (2019). Overview of
surrogate modeling in chemical process engineering.
Chemie Ingenieur Technik, 91(3):228–239.
Mitarai, K., Negoro, M., Kitagawa, M., and Fujii, K.
(2018). Quantum circuit learning. Physical Review
A, 98(3).
Ostaszewski, M., Grant, E., and Benedetti, M. (2021).
Structure optimization for parameterized quantum cir-
cuits. Quantum, 5:391.
P
´
erez-Salinas, A., Cervera-Lierta, A., Gil-Fuster, E., and
Latorre, J. I. (2020). Data re-uploading for a universal
quantum classifier. Quantum, 4:226.
Peters, E. and Schuld, M. (2023). Generalization de-
spite overfitting in quantum machine learning models.
Quantum, 7:1210.
Qin, F., Yan, Z., Yang, P., Tang, S., and Huang, H. (2022).
Deep-learning-based surrogate model for fast and ac-
curate simulation in pipeline transport. Frontiers in
Energy Research, 10.
Qiskit contributors (2023). Qiskit: An open-source frame-
work for quantum computing.
Queipo, N. V., Haftka, R. T., Shyy, W., Goel, T.,
Vaidyanathan, R., and Kevin Tucker, P. (2005).
Surrogate-based analysis and optimization. Progress
in Aerospace Sciences, 41(1):1–28.
Schuld, M. and Petruccione, F. (2021). Machine Learn-
ing with Quantum Computers. Quantum Science and
Technology. Springer International Publishing.
Schuld, M., Sweke, R., and Meyer, J. J. (2021a). Effect of
data encoding on the expressive power of variational
quantum-machine-learning models. Physical Review
A, 103(3).
Schuld, M., Sweke, R., and Meyer, J. J. (2021b). Effect
of data encoding on the expressive power of varia-
tional quantum-machine-learning models. Phys. Rev.
A, 103:032430.
Schwefel, H.-P. (1981). Numerical optimization of com-
puter models. John Wiley & Sons, Inc.
Sim, S., Johnson, P. D., and Aspuru-Guzik, A. (2019).
Expressibility and entangling capability of parame-
terized quantum circuits for hybrid quantum-classical
algorithms. Advanced Quantum Technologies,
2(12):1900070.
Stokes, J. M., Yang, K., Swanson, K., Jin, W., Cubillos-
Ruiz, A., Donghia, N. M., MacNair, C. R., French, S.,
Carfrae, L. A., Bloom-Ackermann, Z., Tran, V. M.,
Chiappino-Pepe, A., Badran, A. H., Andrews, I. W.,
Chory, E. J., Church, G. M., Brown, E. D., Jaakkola,
T. S., Barzilay, R., and Collins, J. J. (2020). A
deep learning approach to antibiotic discovery. Cell,
180(4):688–702.e13.
Styblinski, M. and Tang, T.-S. (1990). Experiments in non-
convex optimization: Stochastic approximation with
function smoothing and simulated annealing. Neural
Networks, 3(4):467–483.
Sun, G. and Wang, S. (2019). A review of the artificial neu-
ral network surrogate modeling in aerodynamic de-
sign. Proceedings of the Institution of Mechanical En-
gineers, Part G: Journal of Aerospace Engineering,
233(16):5863–5872.
Truax, B. (1980). Handbook for acoustic ecology.
Leonardo, 13:83.
Vazquez-Canteli, J., Demir, A. D., Brown, J., and Nagy, Z.
(2019). Deep neural networks as surrogate models for
urban energy simulations. Journal of Physics: Con-
ference Series, 1343(1):012002.
Weigold, M., Barzen, J., Leymann, F., and Salm, M. (2021).
Expanding data encoding patterns for quantum algo-
rithms. In 2021 IEEE 18th International Conference
on Software Architecture Companion (ICSA-C), pages
95–101.
Benchmarking Quantum Surrogate Models on Scarce and Noisy Data
359