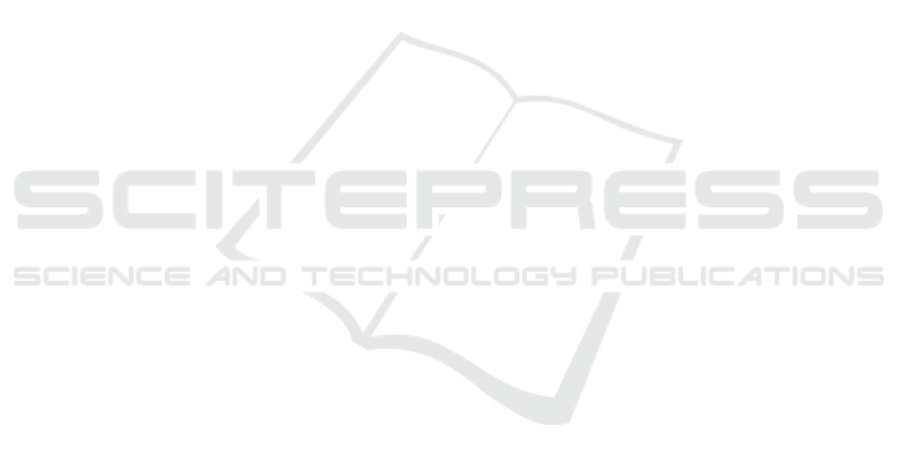
the number of strategy clusters identified by our pro-
posed method, it is evident that the individual util-
ity tends to increase when the number of clusters is
limited, with the highest utility achieved when there
are three clusters. In addition, negotiation simulation
experiments demonstrated that our approach yields
higher individual utility than those of previous stud-
ies.
Although this study provides valuable insights
into meta-strategy for automated negotiation, there re-
main several avenues for future studies. One potential
direction could be the development of new features
for agent clustering. In our proposed method, three
features from the existing opponent features are used
for clustering to group the opponent agents. How-
ever, these features focus on the distribution of of-
fers at the end of the negotiation or at specific time
points, thereby not sufficiently considering the transi-
tion of offers. Therefore, for precise classification of
the behavior of the opponent agent, the incorporation
of new features that can reflect the negotiation process
will be essential.
REFERENCES
Baarslag, T., Hindriks, K., and Jonker, C. (2011). Towards
a quantitative concession-based classification method
of negotiation strategies. In Kinny, D., Hsu, J. Y.-j.,
Governatori, G., and Ghose, A. K., editors, Agents in
Principle, Agents in Practice, pages 143–158, Berlin,
Heidelberg. Springer Berlin Heidelberg.
Baarslag, T., Hindriks, K., and Jonker, C. (2013). A tit
for tat negotiation strategy for real-time bilateral ne-
gotiations. Studies in Computational Intelligence,
435:229–233.
Bagga, P., Paoletti, N., Alrayes, B., and Stathis, K. (2021).
A deep reinforcement learning approach to concurrent
bilateral negotiation. In Proceedings of the Twenty-
Ninth International Joint Conference on Artificial In-
telligence, IJCAI’20.
Bezdek, J. C., Ehrlich, R., and Full, W. (1984). Fcm: The
fuzzy c-means clustering algorithm. Computers &
Geosciences, 10(2):191–203.
Brockman, G., Cheung, V., Pettersson, L., Schneider, J.,
Schulman, J., Tang, J., and Zaremba, W. (2016). Ope-
nAI Gym. arXiv e-prints arXiv:1606.01540.
Faratin, P., Sierra, C., and Jennings, N. R. (1998). Ne-
gotiation decision functions for autonomous agents.
Robotics and Autonomous Systems, 24(3):159–182.
Haarnoja, T., Zhou, A., Abbeel, P., and Levine, S.
(2018). Soft Actor-Critic: Off-Policy Maximum En-
tropy Deep Reinforcement Learning with a Stochastic
Actor. arXiv e-prints arXiv:1801.01290.
Ho, F., Geraldes, R., Gonc¸alves, A., Rigault, B., Sportich,
B., Kubo, D., Cavazza, M., and Prendinger, H. (2022).
Decentralized multi-agent path finding for uav traffic
management. IEEE Transactions on Intelligent Trans-
portation Systems, 23(2):997–1008.
Ilany, L. and Gal, Y. (2016). Algorithm selection in bilat-
eral negotiation. Autonomous Agents and Multi-Agent
Systems, 30(4):697–723.
Lin, R., Kraus, S., Baarslag, T., Tykhonov, D., Hindriks,
K., and Jonker, C. (2014). Genius: An integrated en-
vironment for supporting the design of generic auto-
mated negotiators. Computational Intelligence: an in-
ternational journal, 30(1):48–70. Online verschenen
september 2012; hard copy februari 2014.
Mohammad, Y., Nakadai, S., and Greenwald, A. (2021).
Negmas: A platform for situated negotiations. In
Aydo
˘
gan, R., Ito, T., Moustafa, A., Otsuka, T., and
Zhang, M., editors, Recent Advances in Agent-based
Negotiation, pages 57–75, Singapore. Springer Singa-
pore.
Razeghi, Y., Yavuz, C. O. B., and Aydo
˘
gan, R. (2020). Deep
reinforcement learning for acceptance strategy in bi-
lateral negotiations. Turkish J. Electr. Eng. Comput.
Sci., 28:1824–1840.
Renting, B. M., Hoos, H. H., and Jonker, C. M. (2020).
Automated configuration of negotiation strategies. In
Proceedings of the 19th International Conference on
Autonomous Agents and MultiAgent Systems, AA-
MAS ’20, page 1116–1124, Richland, SC. Interna-
tional Foundation for Autonomous Agents and Mul-
tiagent Systems.
Rubinstein, A. (1982). Perfect equilibrium in a bargaining
model. Econometrica, 50:97–109.
Sengupta, A., Mohammad, Y., and Nakadai, S. (2021).
An autonomous negotiating agent framework with re-
inforcement learning based strategies and adaptive
strategy switching mechanism. In Proceedings of
the 20th International Conference on Autonomous
Agents and MultiAgent Systems, AAMAS ’21, page
1163–1172, Richland, SC. International Foundation
for Autonomous Agents and Multiagent Systems.
van der Putten, S., Robu, V., La Poutr
´
e, H., Jorritsma, A.,
and Gal, M. (2006). Automating supply chain negotia-
tions using autonomous agents: A case study in trans-
portation logistics. In Proceedings of the Fifth Inter-
national Joint Conference on Autonomous Agents and
Multiagent Systems, AAMAS ’06, page 1506–1513,
New York, NY, USA. Association for Computing Ma-
chinery.
van Galen Last, N. (2012). Agent smith: Opponent model
estimation in bilateral multi-issue negotiation. In Ito,
T., Zhang, M., Robu, V., Fatima, S., and Matsuo, T.,
editors, New Trends in Agent-Based Complex Auto-
mated Negotiations, pages 167–174, Berlin, Heidel-
berg. Springer Berlin Heidelberg.
Clustering-Based Approach to Strategy Selection for Meta-Strategy in Automated Negotiation
263