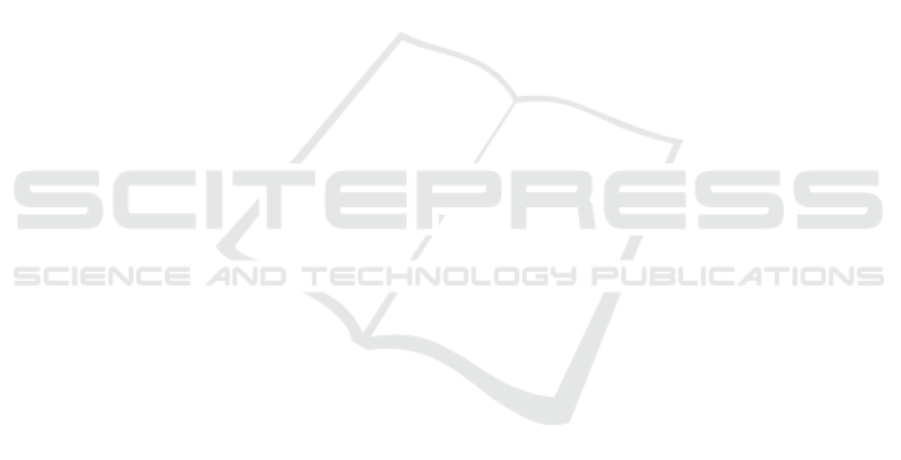
Gudivada, V., Apon, A., and Ding, J. (2017). Data quality
considerations for big data and machine learning: Go-
ing beyond data cleaning and transformations. Inter-
national Journal on Advances in Software, 10(1):1–
20.
Henkel, M., Horn, T., Leboutte, F., Trotsenko, P., Dugas,
S. G., Sutter, S. U., Ficht, G., Engesser, C., Matthias,
M., Stalder, A., Ebbing, J., Cornford, P., Seifert,
H., Stieltjes, B., and Wetterauer, C. (2022). Ini-
tial experience with AI Pathway Companion: Eval-
uation of dashboard-enhanced clinical decision mak-
ing in prostate cancer screening. PLOS ONE,
17(7):e0271183.
Herp, J., Braun, J.-M., Cantuaria, M. L., Tashk, A., Ped-
ersen, T. B., Poulsen, M. H. A., Krogh, M., Nadimi,
E. S., and Sheikh, S. P. (2023). Modeling of electronic
health records for time-variant event learning beyond
bio-markers—a case study in prostate cancer. IEEE
Access, 11:50295–50309.
Holmes, J. H., Beinlich, J., and Boland, M. R. (2021). Why
Is the Electronic Health Record So Challenging for
Research and Clinical Care? Methods of information
in medicine, 60(1-02):32–48.
Javaid, M., Haleem, A., Singh, R. P., Suman, R., and Rab,
S. (2022). Significance of machine learning in health-
care: Features, pillars and applications. International
Journal of Intelligent Networks, 3:58–73.
Knighton, A. J., Belnap, T., Brunisholz, K., Huynh, K., and
Bishoff, J. T. (2016). Using Electronic Health Record
Data to Identify Prostate Cancer Patients That May
Qualify for Active Surveillance. EGEMS (Washing-
ton, DC), 4(3):1220.
Kong, A., Liu, J. S., and Wong, W. H. (1994). Se-
quential imputations and bayesian missing data prob-
lems. Journal of the American Statistical Association,
89(425):278–288.
kuorttinen, E. (2023). HUS Acamedic - secure
operating environment. Available online at:
https://www.hus.fi/en/research-and-education/hus-
acamedic-secure-operating-environment. Accessed
2023-08-22.
Misukka, M. (2022). Standardizing electronic health
records in order to advance secondary use of hospital
data lakes - A case study on HUS data lake. Master’s
thesis, Aalto University. School of Science.
National Cancer Institute (2023). The natural his-
tory of prostate cancer. Available online at:
https://www.cancer.gov/types/prostate. Accessed
2023-08-03.
Park, J., Rho, M. J., Moon, H. W., Kim, J., Lee, C., Kim,
D., Kim, C.-S., Jeon, S. S., Kang, M., and Lee, J. Y.
(2021a). Dr. answer ai for prostate cancer: Predict-
ing biochemical recurrence following radical prostate-
ctomy. Technology in Cancer Research & Treatment,
20.
Park, J., Rho, M. J., Moon, H. W., Park, Y. H., Kim, C.-S.,
Jeon, S. S., Kang, M., and Lee, J. Y. (2021b). Prostate
cancer trajectory-map: clinical decision support sys-
tem for prognosis management of radical prostatec-
tomy. Prostate International, 9(1):25–30.
Pylk
¨
as, J. (2023). HUS facilitates clinical data ex-
ploitation through data lake. Available on-
line at: https://www.tietoevry.com/en/success-
stories/2019/hus-facilitates-clinical-data-exploitation-
through-an-integrated-hus-datalake-solution/. Ac-
cessed 2023-08-22.
Schafer, J. L. (1999). Multiple imputation: a primer. Statis-
tical Methods in Medical Research, 8(1):3–15. PMID:
10347857.
Seneviratne, M. G., Banda, J. M., Brooks, J. D., Shah,
N. H., and Hernandez-Boussard, T. M. (2018). Identi-
fying Cases of Metastatic Prostate Cancer Using Ma-
chine Learning on Electronic Health Records. AMIA
... Annual Symposium proceedings. AMIA Symposium,
2018:1498–1504.
Shen, S., Lepor, H., Yaffee, R., and Taneja, S. S.
(2005). ULTRASENSITIVE SERUM PROSTATE
SPECIFIC ANTIGEN NADIR ACCURATELY PRE-
DICTS THE RISK OF EARLY RELAPSE AFTER
RADICAL PROSTATECTOMY. Journal of Urology,
173(3):777–780.
Stephenson, A. J., Kattan, M. W., Eastham, J. A., Dotan,
Z. A., Bianco, F. J., Lilja, H., and Scardino, P. T.
(2006). Defining biochemical recurrence of prostate
cancer after radical prostatectomy: A proposal for a
standardized definition. Journal of Clinical Oncology,
24(24):3973–3978. PMID: 16921049.
The American Cancer Society medical and editorial con-
tent team (2019). Prostate cancer. Available
online at: https://www.cancer.org/cancer/prostate-
cancer/about/what-is-prostate-cancer.html. Accessed
2023-08-03.
Thirunavukarasu, A. J., Ting, D. S. J., Elangovan, K.,
Gutierrez, L., Tan, T. F., and Ting, D. S. W. (2023).
Large language models in medicine. Nature Medicine,
29(8):1930–1940.
Van Den Broeck, T., Van Den Bergh, R. C., Briers, E.,
Cornford, P., Cumberbatch, M., Tilki, D., De San-
tis, M., Fanti, S., Fossati, N., Gillessen, S., Grum-
met, J. P., Henry, A. M., Lardas, M., Liew, M., Ma-
son, M., Moris, L., Schoots, I. G., Van Der Kwast,
T., Van Der Poel, H., Wiegel, T., Willemse, P.-P. M.,
Rouvi
`
ere, O., Lam, T. B., and Mottet, N. (2020). Bio-
chemical Recurrence in Prostate Cancer: The Euro-
pean Association of Urology Prostate Cancer Guide-
lines Panel Recommendations. European Urology Fo-
cus, 6(2):231–234.
Wald, A. (1949). Note on the consistency of the maxi-
mum likelihood estimate. The Annals of Mathemat-
ical Statistics, 20(4):595–601.
Yadav, P., Steinbach, M., Kumar, V., and Simon, G. (2018).
Mining Electronic Health Records (EHRs): A Survey.
ACM Computing Surveys, 50(6):1–40.
Zhao, Y., Tao, Z., Li, L., Zheng, J., and Chen, X. (2022).
Predicting biochemical-recurrence-free survival using
a three-metabolic-gene risk score model in prostate
cancer patients. BMC Cancer, 22(1):239.
Synergizing Data Imputation and Electronic Health Records for Advancing Prostate Cancer Research: Challenges, and Practical
Applications
85