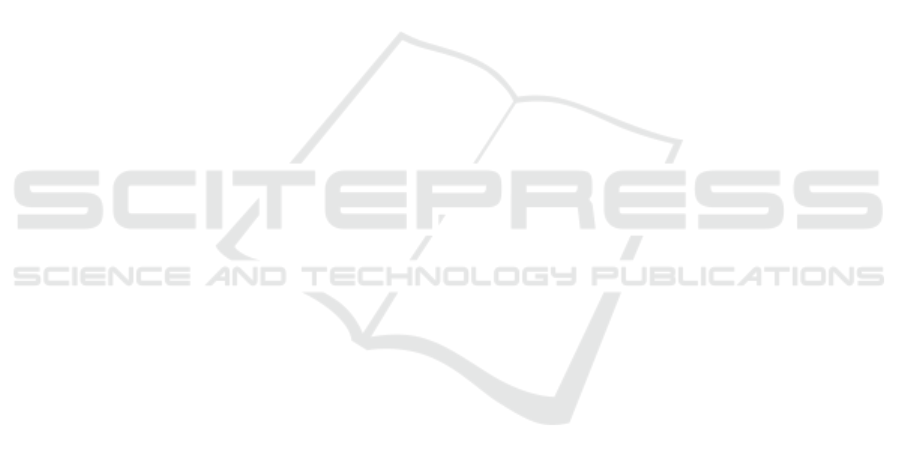
Gao, B. and Spratling, M. W. (2022). Robust template
matching via hierarchical convolutional features from
a shape biased cnn. In The International Confer-
ence on Image, Vision and Intelligent Systems (ICIVIS
2021), pages 333–344.
Guetter, C., Xu, C., Sauer, F., and Hornegger, J. (2005).
Learning based non-rigid multi-modal image registra-
tion using kullback-leibler divergence. In Medical Im-
age Computing and Computer-Assisted Intervention–
MICCAI 2005: 8th International Conference, Palm
Springs, CA, USA, October 26-29, 2005, Proceedings,
Part II 8, pages 255–262.
Haskins, G., Kruecker, J., Kruger, U., Xu, S., Pinto, P. A.,
Wood, B. J., and Yan, P. (2019). Learning deep simi-
larity metric for 3d mr–trus image registration. Inter-
national journal of computer assisted radiology and
surgery, 14:417–425.
Jiang, X., Ma, J., Xiao, G., Shao, Z., and Guo, X. (2021). A
review of multimodal image matching: Methods and
applications. Information Fusion, 73:22–71.
Lin, W., Li, X., Yang, Z., Manga, M., Fu, X., Xiong, S.,
Gong, A., Chen, G., Li, H., Pei, L., Li, S., Zhao,
X., and Wang, X. (2019). Multiscale digital porous
rock reconstruction using template matching. Water
Resources Research, 55(8):6911–6922.
Loeckx, D., Slagmolen, P., Maes, F., Vandermeulen, D., and
Suetens, P. (2009). Nonrigid image registration using
conditional mutual information. IEEE transactions on
medical imaging, 29(1):19–29.
Luo, Z., Shen, T., Zhou, L., Zhang, J., Yao, Y., Li, S., Fang,
T., and Quan, L. (2019). Contextdesc: Local descrip-
tor augmentation with cross-modality context. In Pro-
ceedings of the IEEE/CVF conference on computer vi-
sion and pattern recognition, pages 2527–2536.
Ma, W., Li, W., and Cao, P. (2019). Ranging method of
binocular stereo vision based on random ferns and ncc
template matching. In IOP Conference Series: Earth
and Environmental Science, page 022149.
Maes, F., Collignon, A., Vandermeulen, D., Marchal, G.,
and Suetens, P. (1997). Multimodality image regis-
tration by maximization of mutual information. IEEE
transactions on Medical Imaging, 16(2):187–198.
Marcu, L. (2012). Fluorescence lifetime techniques in med-
ical applications. Annals of biomedical engineering,
40:304–331.
Mench
´
on-Lara, R.-M., Simmross-Wattenberg, F.,
Rodr
´
ıguez-Cayetano, M., de-la Higuera, P. C.,
Mart
´
ın-Fern
´
andez, M.
´
A., and Alberola-L
´
opez, C.
(2023). Efficient convolution-based pairwise elastic
image registration on three multimodal similarity
metrics. Signal Processing, 202:108771.
Ono, Y., Trulls, E., Fua, P., and Yi, K. M. (2018). Lf-
net: Learning local features from images. Advances
in neural information processing systems, 31.
Simonovsky, M., Guti
´
errez-Becker, B., Mateus, D., Navab,
N., and Komodakis, N. (2016). A deep metric for mul-
timodal registration. In Medical Image Computing
and Computer-Assisted Intervention-MICCAI 2016:
19th International Conference, Athens, Greece, Octo-
ber 17-21, 2016, Proceedings, Part III 19, pages 10–
18.
Sonka, M., Hlavac, V., and Boyle, R. (2014). Image
processing, analysis, and machine vision. Cengage
Learning.
Stefano, L. D., Mattoccia, S., and Tombari, F. (2005). Zncc-
based template matching using bounded partial corre-
lation. Pattern recognition letters, 26(14):2129–2134.
Taimori, A., Mills, B., Gaughan, E., Ali, A., Dhaliwal, K.,
Williams, G., Finlayson, N., and Hopgood, J. (2023).
A novel fit-flexible fluorescence imager: Tri-sensing
of intensity, fall-time, and life profile. TechRxiv.
Wang, J., Zhou, F., Wen, S., Liu, X., and Lin, Y. (2017).
Deep metric learning with angular loss. In Proceed-
ings of the IEEE international conference on com-
puter vision, pages 2593–2601.
Wang, Q., Fernandes, S., Williams, G. O., Finlayson,
N., Akram, A. R., Dhaliwal, K., Hopgood, J. R.,
and Vallejo, M. (2022a). Deep learning-assisted co-
registration of full-spectral autofluorescence lifetime
microscopic images with h&e-stained histology im-
ages. Communications biology, 5(1):1119.
Wang, Q., Hopgood, J. R., Fernandes, S., Finlayson, N.,
Williams, G. O., Akram, A. R., Dhaliwal, K., and
Vallejo, M. (2022b). A layer-level multi-scale archi-
tecture for lung cancer classification with fluorescence
lifetime imaging endomicroscopy. Neural Computing
and Applications, 34(21):18881–18894.
Wu, G., Kim, M., Wang, Q., Gao, Y., Liao, S., and
Shen, D. (2013). Unsupervised deep feature learn-
ing for deformable registration of mr brain images.
In Medical Image Computing and Computer-Assisted
Intervention–MICCAI 2013: 16th International Con-
ference, Nagoya, Japan, September 22-26, 2013, Pro-
ceedings, Part II 16, pages 649–656.
Wu, G., Kim, M., Wang, Q., Munsell, B. C., and Shen, D.
(2015). Scalable high-performance image registration
framework by unsupervised deep feature representa-
tions learning. IEEE transactions on biomedical engi-
neering, 63(7):1505–1516.
Yang, K., Pan, A., Yang, Y., Zhang, S., Ong, S. H., and
Tang, H. (2017). Remote sensing image registra-
tion using multiple image features. Remote Sensing,
9(6):581.
Ye, Y., Bruzzone, L., Shan, J., Bovolo, F., and Zhu, Q.
(2019). Fast and robust matching for multimodal re-
mote sensing image registration. IEEE Transactions
on Geoscience and Remote Sensing, 57(11):9059–
9070.
Zhang, L. and Rusinkiewicz, S. (2018). Learning to detect
features in texture images. In Proceedings of the IEEE
conference on computer vision and pattern recogni-
tion, pages 6325–6333.
Zhang, X., Yu, F. X., Karaman, S., and Chang, S.-F. (2017).
Learning discriminative and transformation covariant
local feature detectors. In Proceedings of the IEEE
conference on computer vision and pattern recogni-
tion, pages 6818–6826.
Quantification of Matching Results for Autofluorescence Intensity Images and Histology Images
713