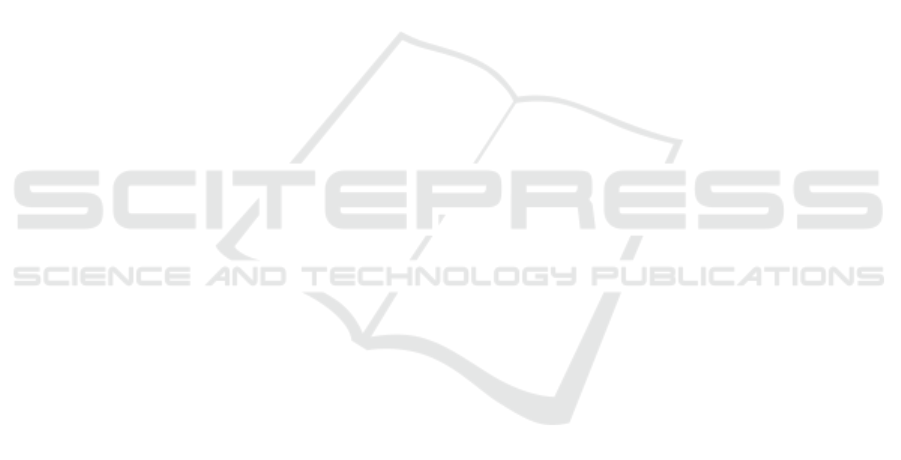
therapy, NIV and invasive mechanical ventilation, de-
pending on severity (Wang et al., 2020) (Spadari and
Gardenghi, 2020).
(Gu
´
erin et al., 2020) the prone positioning tech-
nique, known as the prone position, which has been
used for years, is now recommended for sedated,
mechanically ventilated patients taking neuromus-
cular blockers, especially those suffering from se-
vere to moderate acute respiratory distress syndrome
(ARDS).
In the context of the COVID-19 pandemic, a study
carried out in China with a sample of 1,009 patients
revealed that 41% of all patients required hospitaliza-
tion, with more than 70% of cases considered serious
requiring the administration of supplementary oxygen
(Siemieniuk et al., 2018).
For Elharrar (Elharrar et al., 2020), only 63% of
the total of 24 patients with hypoxemic respiratory
failure with COVID19 were able to endure more than
three hours in the prone position and oxygenation im-
proved in only 25% of this sample.
Patients who remained in the prone position for
three hours experienced an improvement in oxygena-
tion, while those who did so for just one hour had
unfavorable outcomes, including intubation (Elharrar
et al., 2020).
Some studies explore the application of computa-
tional methods for monitoring and predictions based
on hospital data. As an example, Meneses’ study
(Meneses, 2021) explores the use of machine learn-
ing algorithms, such as Random Forest and Gradient
Boost, to predict ICU patient admission based on data
from the first 24, 48 and 72 hours of hospitalization.
Using data from a real hospital during the COVID-
19 pandemic, Gradient Boost models showed the best
performance metrics. For example, for data from
the first 24 hours, Gradient Boost achieved AUROC
of 92.7%, Accuracy of 61%, Sensitivity of 81.6%,
Specificity of 86.1%, Accuracy of 85.1% and F1-
Score of 69.9%. The study suggests that this approach
can be an effective tool in predicting ICU admissions,
helping with hospital management.
(Fabrizzio et al., 2023) proposes the development
of a Web App using a decision tree model to estimate
the risk of ICU admission for patients with COVID-
19. Streamlit, created in Python, stratifies patients
based on variables associated with Precision Nursing,
assisting healthcare professionals in making clinical
decisions. Despite the possible impact of vaccination
on data, the Web App proved to be viable for pre-
senting research results in an understandable way and
supporting clinical decision-making.
Given the difficulties of treating respiratory failure
in COVID-19 patients and their current high mortality
rate, it is essential to develop software based on arti-
ficial intelligence to optimize this type of treatment.
The system would be based on AI to apply scales and
care flows objectively, learning from previous data to
improve the effectiveness of procedures and thus im-
prove care while reducing hospital costs.
This study presents a quantum computing al-
gorithm for predictive analysis in the screening of
COVID patients. This algorithm is based on the Vari-
ational Quantum Classifier (VQC). For comparison
purposes, a widely recognized deep neural network
(DNN) model was used.
The use of quantum computing is driven by im-
proved artificial intelligence, faster processing of ma-
trices and vectors and the joint properties of qubits.
Achieving the research objectives will allow pa-
tients to receive more effective treatment in cases of
readmission for similar reasons, as well as benefiting
new patients with similar profiles.
The article is carried out without any commercial
or financial relationship that could be interpreted as
a potential conflict of interest. It is divided into four
sections: section 2 deals with the computational tech-
nique used in the work, while section 3 describes the
results obtained and, finally, the conclusion.
2 METHODOLOGY
The representation of the process adopted can be seen
in the flowchart 1. First there is the data input, then
there are two architectures that can be used, flow 1 or
flow 2. Flow 1 is characterised by the use of the DNN
algorithm and flow 2 by the use of PCA followed by
the VQC algorithm. Finally, the classification result
is obtained.
2.1 Dataset
This project is based on the (Barros et al., 2022)
dataset, which was developed from an observational,
longitudinal and retrospective study of patients who
were exposed to respiratory failure treatment at a
reference hospital for the treatment of COVID-19
in Teresina-PI. Biometric data and monitoring signs
were taken into account.
The dataset shows only patients with respiratory
failure diagnosed with COVID-19 by the new SARS-
CoV-2 coronavirus defined by molecular tests (PCR-
RT).
The input elements that were part of the pro-
gramme and data collection were: oxygenation con-
trol indices such as PaO2/FiO2, age, gender, address,
pathological history, symptoms, monitoring data such
BIODEVICES 2024 - 17th International Conference on Biomedical Electronics and Devices
112