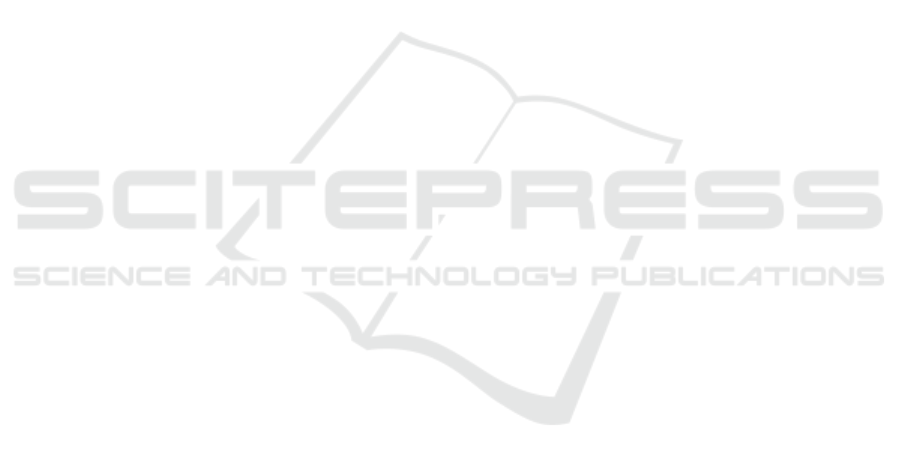
observation space due to angle embedding. We pro-
posed a new encoding block architecture - stacked
VQC - which allows the utilization of additional
qubits, resulting in improved training performance.
It has been previously shown that an increase of the
number of layers improves training performance only
until a threshold (Skolik et al., 2022). We reveal a
similar trend: an increase of the number of qubits
substantially improves training performance, but also
only until a certain limit. Our work indicates that
current VQC architectures therefore are limited both
in the number of layers, as well as in the amount of
qubits, and thus dictate both the depth and the width
of the circuit, respectively. While we investigated and
enhanced current variational quantum circuit design
choices, future work should aim to further improve
upon these results as well as explore novel circuit ar-
chitectures in order to bridge the performance gap be-
tween QRL and RL.
ACKNOWLEDGEMENTS
The research is part of the Munich Quantum Valley,
which is supported by the Bavarian state government
with funds from the Hightech Agenda Bayern Plus.
REFERENCES
Abbas, A., Sutter, D., Zoufal, C., Lucchi, A., Figalli, A.,
and Woerner, S. (2021). The power of quantum neural
networks. Nature Computational Science, 1(6):403–
409.
Acuto, A., Barilla, P., Bozzolo, L., Conterno, M., Pavese,
M., and Policicchio, A. (2022). Variational quantum
soft actor-critic for robotic arm control. arXiv preprint
arXiv:2212.11681.
Banchi, L., Pereira, J., and Pirandola, S. (2021). Gener-
alization in quantum machine learning: A quantum
information standpoint. PRX Quantum, 2(4):040321.
Benedetti, M., Garcia-Pintos, D., Perdomo, O., Leyton-
Ortega, V., Nam, Y., and Perdomo-Ortiz, A. (2019). A
generative modeling approach for benchmarking and
training shallow quantum circuits. npj Quantum In-
formation, 5(1).
Caro, M. C., Huang, H.-Y., Cerezo, M., Sharma, K., Sorn-
borger, A., Cincio, L., and Coles, P. J. (2022). Gener-
alization in quantum machine learning from few train-
ing data. Nature Communications, 13(1).
Dalzell, A. M., McArdle, S., Berta, M., Bienias, P., Chen,
C.-F., Gily
´
en, A., Hann, C. T., Kastoryano, M. J.,
Khabiboulline, E. T., Kubica, A., Salton, G., Wang, S.,
and Brand
˜
ao, F. G. S. L. (2023). Quantum algorithms:
A survey of applications and end-to-end complexities.
Dr
˘
agan, T.-A., Monnet, M., Mendl, C. B., and Lorenz, J. M.
(2022). Quantum reinforcement learning for solv-
ing a stochastic frozen lake environment and the im-
pact of quantum architecture choices. arXiv preprint
arXiv:2212.07932.
Du, Y., Hsieh, M.-H., Liu, T., and Tao, D. (2020). Expres-
sive power of parametrized quantum circuits. Phys.
Rev. Res., 2:033125.
Jerbi, S., Gyurik, C., Marshall, S., Briegel, H., and Dunjko,
V. (2021). Parametrized quantum policies for rein-
forcement learning. Advances in Neural Information
Processing Systems, 34:28362–28375.
McClean, J. R., Boixo, S., Smelyanskiy, V. N., Babbush,
R., and Neven, H. (2018). Barren plateaus in quantum
neural network training landscapes. Nature Commu-
nications, 9(1).
Meyer, N., Scherer, D., Plinge, A., Mutschler, C., and Hart-
mann, M. (2023). Quantum policy gradient algorithm
with optimized action decoding. In International Con-
ference on Machine Learning, pages 24592–24613.
PMLR.
Meyer, N., Ufrecht, C., Periyasamy, M., Scherer, D. D.,
Plinge, A., and Mutschler, C. (2022). A survey on
quantum reinforcement learning.
Park, S., Kim, J. P., Park, C., Jung, S., and Kim, J. (2023).
Quantum multi-agent reinforcement learning for au-
tonomous mobility cooperation. IEEE Communica-
tions Magazine.
P
´
erez-Salinas, A., Cervera-Lierta, A., Gil-Fuster, E., and
Latorre, J. I. (2020). Data re-uploading for a universal
quantum classifier. Quantum, 4:226.
Qian, Y., Wang, X., Du, Y., Wu, X., and Tao, D. (2022). The
dilemma of quantum neural networks. IEEE Transac-
tions on Neural Networks and Learning Systems.
Schuld, M., Sweke, R., and Meyer, J. J. (2021). Effect of
data encoding on the expressive power of variational
quantum-machine-learning models. Physical Review
A, 103(3):032430.
Skolik, A., Jerbi, S., and Dunjko, V. (2022). Quantum
agents in the gym: a variational quantum algorithm
for deep q-learning. Quantum, 6:720.
Wu, S., Jin, S., Wen, D., and Wang, X. (2020). Quantum
reinforcement learning in continuous action space.
arXiv preprint arXiv:2012.10711.
ICAART 2024 - 16th International Conference on Agents and Artificial Intelligence
400