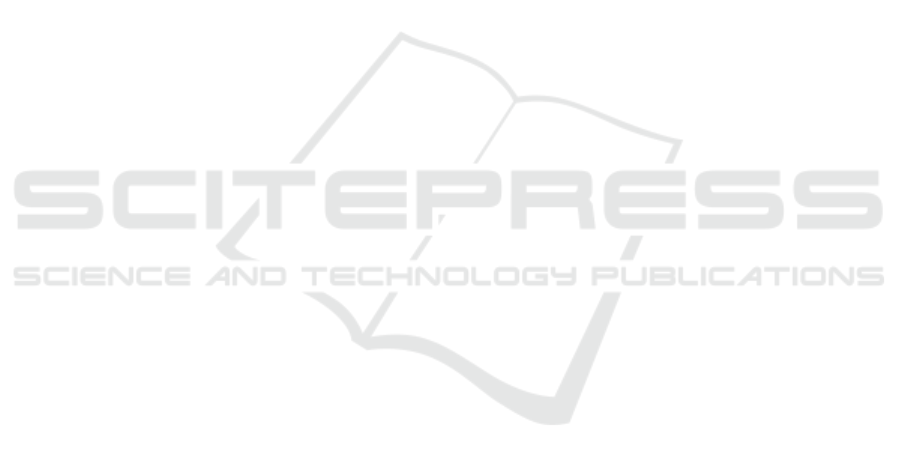
able CNN for joint description and detection of local
features. In CVPR.
Edstedt, J., Athanasiadis, I., Wadenb
¨
ack, M., and Felsberg,
M. (2023). Dkm: Dense kernelized feature match-
ing for geometry estimation. In Proceedings of the
IEEE/CVF Conference on Computer Vision and Pat-
tern Recognition, pages 17765–17775.
Germain, H., Bourmaud, G., and Lepetit, V. (2020).
S2DNet: learning image features for accurate sparse-
to-dense matching. In ECCV.
Germain, H., Lepetit, V., and Bourmaud, G. (2021). Neural
reprojection error: Merging feature learning and cam-
era pose estimation. In CVPR.
Germain, H., Lepetit, V., and Bourmaud, G. (2022). Visual
correspondence hallucination. In ICLR.
Giang, K. T., Song, S., and Jo, S. (2023). TopicFM: Robust
and interpretable feature matching with topic-assisted.
In AAAI.
Heinly, J., Sch
¨
onberger, J. L., Dunn, E., and Frahm, J.-M.
(2015). Reconstructing the World* in Six Days *(as
Captured by the Yahoo 100 Million Image Dataset).
In CVPR.
Jaegle, A., Borgeaud, S., Alayrac, J.-B., Doersch, C.,
Ionescu, C., Ding, D., Koppula, S., Zoran, D., Brock,
A., Shelhamer, E., Henaff, O. J., Botvinick, M., Zis-
serman, A., Vinyals, O., and Carreira, J. (2022). Per-
ceiver IO: A general architecture for structured inputs
& outputs. In ICLR.
Jaegle, A., Gimeno, F., Brock, A., Vinyals, O., Zisserman,
A., and Carreira, J. (2021). Perceiver: General per-
ception with iterative attention. In ICML.
Jin, Y., Mishkin, D., Mishchuk, A., Matas, J., Fua, P., Yi,
K. M., and Trulls, E. (2020). Image Matching across
Wide Baselines: From Paper to Practice. IJCV.
Katharopoulos, A., Vyas, A., Pappas, N., and Fleuret, F.
(2020). Transformers are RNNs: Fast autoregressive
transformers with linear attention. In ICML.
Li, X., Han, K., Li, S., and Prisacariu, V. (2020). Dual-
resolution correspondence networks. NeurIPS.
Li, Z. and Snavely, N. (2018). Megadepth: Learning single-
view depth prediction from internet photos. In CVPR.
Lin, T.-Y., Doll
´
ar, P., Girshick, R., He, K., Hariharan, B.,
and Belongie, S. (2017). Feature pyramid networks
for object detection. In CVPR.
Lowe, D. G. (1999). Object recognition from local scale-
invariant features. In ICCV.
Mao, R., Bai, C., An, Y., Zhu, F., and Lu, C. (2022). 3DG-
STFM: 3D geometric guided student-teacher feature
matching. In ECCV.
Ni, J., Li, Y., Huang, Z., Li, H., Bao, H., Cui, Z., and Zhang,
G. (2023). PATS: Patch area transportation with sub-
division for local feature matching. In Proceedings
of the IEEE/CVF Conference on Computer Vision and
Pattern Recognition, pages 17776–17786.
Ono, Y., Trulls, E., Fua, P., and Yi, K. M. (2018). LF-Net:
learning local features from images. NeurIPS.
Revaud, J., De Souza, C., Humenberger, M., and Weinza-
epfel, P. (2019). R2D2: reliable and repeatable detec-
tor and descriptor. NeurIPS.
Rocco, I., Arandjelovi
´
c, R., and Sivic, J. (2020a). Efficient
neighbourhood consensus networks via submanifold
sparse convolutions. In ECCV.
Rocco, I., Cimpoi, M., Arandjelovi
´
c, R., Torii, A., Pajdla,
T., and Sivic, J. (2018). Neighbourhood consensus
networks. NeurIPS.
Rocco, I., Cimpoi, M., Arandjelovi
´
c, R., Torii, A., Pajdla,
T., and Sivic, J. (2020b). NCNet: neighbourhood
consensus networks for estimating image correspon-
dences. PAMI.
Sarlin, P.-E., DeTone, D., Malisiewicz, T., and Rabinovich,
A. (2020). Superglue: Learning feature matching with
graph neural networks. In CVPR.
Sarlin, P.-E., Dusmanu, M., Sch
¨
onberger, J. L., Speciale,
P., Gruber, L., Larsson, V., Miksik, O., and Pollefeys,
M. (2022). LaMAR: Benchmarking Localization and
Mapping for Augmented Reality. In ECCV.
Sattler, T., Torii, A., Sivic, J., Pollefeys, M., Taira, H., Oku-
tomi, M., and Pajdla, T. (2017). Are large-scale 3D
models really necessary for accurate visual localiza-
tion? In CVPR.
Sch
¨
onberger, J. L. and Frahm, J.-M. (2016). Structure-
from-motion revisited. In CVPR.
Sch
¨
ops, T., Sattler, T., and Pollefeys, M. (2019). BAD
SLAM: Bundle adjusted direct RGB-D SLAM. In
CVPR.
Strasdat, H., Davison, A. J., Montiel, J. M., and Konolige,
K. (2011). Double window optimisation for constant
time visual slam. In ICCV.
Sun, J., Shen, Z., Wang, Y., Bao, H., and Zhou, X.
(2021). LoFTR: detector-free local feature matching
with transformers. In CVPR.
Sv
¨
arm, L., Enqvist, O., Kahl, F., and Oskarsson, M. (2017).
City-scale localization for cameras with known verti-
cal direction. PAMI.
Taira, H., Okutomi, M., Sattler, T., Cimpoi, M., Pollefeys,
M., Sivic, J., Pajdla, T., and Torii, A. (2018). In-
Loc: Indoor visual localization with dense matching
and view synthesis. In CVPR.
Tang, S., Zhang, J., Zhu, S., and Tan, P. (2022). Quadtree
attention for vision transformers. In ICLR.
Truong, P., Danelljan, M., and Timofte, R. (2020). Glu-
net: Global-local universal network for dense flow and
correspondences. In CVPR.
Truong, P., Danelljan, M., Van Gool, L., and Timofte, R.
(2021). Learning accurate dense correspondences and
when to trust them. In CVPR.
Vaswani, A., Shazeer, N., Parmar, N., Uszkoreit, J., Jones,
L., Gomez, A. N., Kaiser, Ł., and Polosukhin, I.
(2017). Attention is all you need. NeurIPS.
Wang, Q., Zhang, J., Yang, K., Peng, K., and Stiefelha-
gen, R. (2022). Matchformer: Interleaving attention
in transformers for feature matching. In ACCV.
Wang, W., Xie, E., Li, X., Fan, D.-P., Song, K., Liang, D.,
Lu, T., Luo, P., and Shao, L. (2021). Pyramid vision
transformer: A versatile backbone for dense predic-
tion without convolutions. In ICCV.
Xie, E., Wang, W., Yu, Z., Anandkumar, A., Alvarez, J. M.,
and Luo, P. (2021). Segformer: Simple and efficient
Are Semi-Dense Detector-Free Methods Good at Matching Local Features?
45