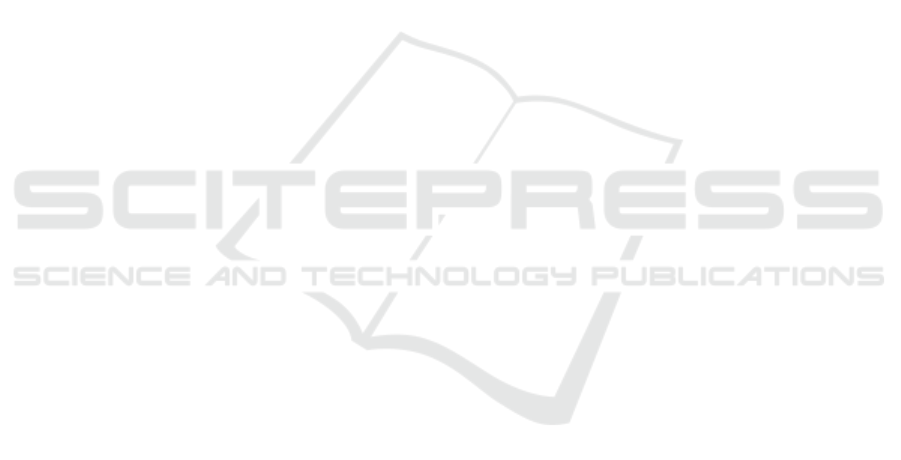
a low average absolute difference between predicted
and actual SpO2 values. Additionally, the RMSE
value, which is derived from the MSE and equals
1.23, provides a comprehensible metric in the orig-
inal SpO2 scale, signifying that the model’s predic-
tions align well with the true values. There was no
difference observed in the data between the three sub-
jects.
Moreover, the R
2
value of 0.68 demonstrates the
model’s ability to explain roughly 68% of the variance
in SpO2, indicating a good fit to the underlying data
patterns.
4 CONCLUSION
This paper investigates the application of a wearable
laser-induced graphene respiration sensor for SpO2
estimation as a substitute for PPG-based oximeters,
foremost used as a tool in a triage process in mass
casualty events. The LIG sensor is placed in 7 differ-
ent positions on the individual’s chest to facilitate the
real-time monitoring of respiratory signals.
The obtained promising results for estimating
SpO2 with LIG signals processed by HeartPy have
shown another possible utilization of the wearable
mechanical deflection sensors as a part of integrated
patch-like sensors. The neural network’s performance
shows potential, as indicated by regression metrics,
including a mean squared error (MSE) of 0.152, a
mean absolute error (MAE) of 1.13, a root mean
square error (RMSE) of 1.23, and an R
2
score of 0.68.
By combining PPG and respiratory signals from
graphene, we offer an idea for developing 2D sensors
for emergency situations, leading to better monitor-
ing and management of various medical conditions.
However, further research is needed to explore the
potential correlations between these signals and their
clinical applications, as well as realistic performance
under application in the field. For example, motion
artefacts may appear in signals, and additional filter-
ing may need to be applied to remedy it.
The study limitation includes the small number of
instances where SpO2 was measured below 90%, as
we faced challenges in obtaining access to individuals
with respiratory difficulties.
Ethical Considerations
The signals were recorded and conducted with ap-
proval from the Ethics Committee.
ACKNOWLEDGEMENTS
This work was supported in part by the NATO Sci-
ence for Peace and Security Program under project
SP4LIFE, number G5825. We also acknowledge sup-
port by the Serbian Ministry of Science, Technolog-
ical Development, and Innovations, contract num-
ber 451-03-47/2023–01/200026. This work was sup-
ported in part by the Faculty of Computer Science
and Engineering in Skopje, North Macedonia under
project BIOX.
REFERENCES
Benson, M., Koenig, K. L., and Schultz, C. H. (1996). Dis-
aster triage: Start, then save—a new method of dy-
namic triage for victims of a catastrophic earthquake.
Prehospital and disaster medicine, 11(2):117–124.
Castaneda, D., Esparza, A., Ghamari, M., and Soltanpur,
C. (2018). Hji jo b. Nazeran, and bioelectronics,”
A review on wearable photoplethysmography sensors
and their potential future applications in health care,
4(4):195.
Chan, M., Ganti, V. G., Heller, J. A., Abdallah, C. A.,
Etemadi, M., and Inan, O. T. (2021). Enabling contin-
uous wearable reflectance pulse oximetry at the ster-
num. Biosensors, 11(12):521.
Chen, H., Bao, S., Ma, J., Wang, P., Lu, H., Oetomo,
S. B., and Chen, W. (2019). A wearable daily respi-
ration monitoring system using pdms-graphene com-
pound tensile sensor for adult. In 2019 41st Annual
International Conference of the IEEE Engineering in
Medicine and Biology Society (EMBC), pages 1269–
1273. IEEE.
Dias, D. and Paulo Silva Cunha, J. (2018). Wearable health
devices—vital sign monitoring, systems and technolo-
gies. Sensors, 18(8):2414.
Kleine B
¨
uning, M., Kern, P., and Sinz, C. (2020). Verifying
equivalence properties of neural networks with relu
activation functions. In International Conference on
Principles and Practice of Constraint Programming,
pages 868–884. Springer.
Kolbæk, M., Tan, Z.-H., Jensen, S. H., and Jensen, J.
(2020). On loss functions for supervised monaural
time-domain speech enhancement. IEEE/ACM Trans-
actions on Audio, Speech, and Language Processing,
28:825–838.
Koteska, B., Bodanova, A. M., Mitrova, H., Sidorenko, M.,
and Lehocki, F. (2022). A deep learning approach
to estimate spo2 from ppg signals. In Proceedings
of the 9th International Conference on Bioinformatics
Research and Applications, pages 142–148.
Majumder, S., Mondal, T., and Deen, M. J. (2017). Wear-
able sensors for remote health monitoring. Sensors,
17(1):130.
Na, S. J., Ko, R.-E., Ko, M. G., and Jeon, K. (2021). Auto-
mated alert and activation of medical emergency team
Prediction of Oxygen Saturation from Graphene Respiratory Signals with PPG Trained DNN
745