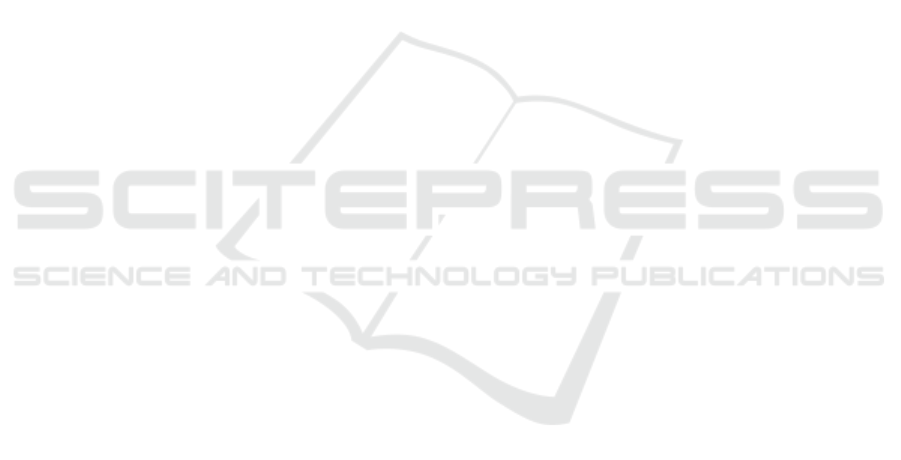
Kong, T., Sun, F., Liu, H., Jiang, Y., Li, L., and Shi,
J. (2020). Foveabox: Beyound anchor-based object
detection. IEEE Transactions on Image Processing,
29:7389–7398.
Kragh, M. F., Christiansen, P., Laursen, M. S., Larsen, M.,
Steen, K. A., Green, O., Karstoft, H., and Jørgensen,
R. N. (2017). Fieldsafe: dataset for obstacle detection
in agriculture. Sensors, 17(11):2579.
Lin, T.-Y., Doll
´
ar, P., Girshick, R., He, K., Hariharan, B.,
and Belongie, S. (2017a). Feature pyramid networks
for object detection. In Proceedings of the IEEE con-
ference on computer vision and pattern recognition,
pages 2117–2125.
Lin, T.-Y., Goyal, P., Girshick, R., He, K., and Doll
´
ar, P.
(2017b). Focal loss for dense object detection. In
Proceedings of the IEEE international conference on
computer vision, pages 2980–2988.
Lin, T.-Y., Maire, M., Belongie, S., Hays, J., Perona, P.,
Ramanan, D., Doll
´
ar, P., and Zitnick, C. L. (2014).
Microsoft coco: Common objects in context. In Euro-
pean conference on computer vision, pages 740–755.
Springer.
Liu, S., Zhou, H., Li, C., and Wang, S. (2020). Analy-
sis of anchor-based and anchor-free object detection
methods based on deep learning. In 2020 IEEE Inter-
national Conference on Mechatronics and Automation
(ICMA), pages 1058–1065. IEEE.
Maddern, W., Pascoe, G., Linegar, C., and Newman, P.
(2017). 1 year, 1000 km: The oxford robotcar
dataset. The International Journal of Robotics Re-
search, 36(1):3–15.
Neigel, P., Ameli, M., Katrolia, J., Feld, H., Wasenm
¨
uller,
O., and Stricker, D. (2020). Opedd: Off-road pedes-
trian detection dataset.
Neigel, P., Rambach, J. R., and Stricker, D. (2021). Offsed:
Off-road semantic segmentation dataset. In VISI-
GRAPP (4: VISAPP), pages 552–557.
Petsiuk, V., Jain, R., Manjunatha, V., Morariu, V. I., Mehra,
A., Ordonez, V., and Saenko, K. (2021). Black-box
explanation of object detectors via saliency maps. In
Proceedings of the IEEE/CVF Conference on Com-
puter Vision and Pattern Recognition, pages 11443–
11452.
Rezatofighi, H., Tsoi, N., Gwak, J., Sadeghian, A., Reid, I.,
and Savarese, S. (2019). Generalized intersection over
union: A metric and a loss for bounding box regres-
sion. In Proceedings of the IEEE/CVF Conference on
Computer Vision and Pattern Recognition, pages 658–
666.
Tian, Z., Shen, C., Chen, H., and He, T. (2019). Fcos:
Fully convolutional one-stage object detection. In
Proceedings of the IEEE/CVF international confer-
ence on computer vision, pages 9627–9636.
Tong, K., Wu, Y., and Zhou, F. (2020). Recent advances
in small object detection based on deep learning: A
review. Image and Vision Computing, 97:103910.
Woo, S., Park, J., Lee, J.-Y., and Kweon, I. S. (2018). Cbam:
Convolutional block attention module. In Proceed-
ings of the European conference on computer vision
(ECCV), pages 3–19.
Xiang, Y., Wang, H., Su, T., Li, R., Brach, C., Mao, S. S.,
and Geimer, M. (2020). Kit moma: A mobile ma-
chines dataset. arXiv preprint arXiv:2007.04198.
Xie, S., Girshick, R., Doll
´
ar, P., Tu, Z., and He, K. (2017).
Aggregated residual transformations for deep neural
networks. In Proceedings of the IEEE conference on
computer vision and pattern recognition, pages 1492–
1500.
Yu, J., Jiang, Y., Wang, Z., Cao, Z., and Huang, T. (2016).
Unitbox: An advanced object detection network. In
Proceedings of the 24th ACM international confer-
ence on Multimedia, pages 516–520.
Zheng, Z., Wang, P., Liu, W., Li, J., Ye, R., and Ren, D.
(2020). Distance-iou loss: Faster and better learn-
ing for bounding box regression. In Proceedings of
the AAAI Conference on Artificial Intelligence, vol-
ume 34, pages 12993–13000.
Zhu, C., He, Y., and Savvides, M. (2019a). Feature selec-
tive anchor-free module for single-shot object detec-
tion. In Proceedings of the IEEE/CVF Conference on
Computer Vision and Pattern Recognition, pages 840–
849.
Zhu, X., Cheng, D., Zhang, Z., Lin, S., and Dai, J. (2019b).
An empirical study of spatial attention mechanisms
in deep networks. In Proceedings of the IEEE/CVF
International Conference on Computer Vision, pages
6688–6697.
APPENDIX
Further results from training the anchor-free dense
model over the OPEDD dataset and the COCO bench-
mark dataset are presented in this Appendix. These
results show more information about using different
IoU loss functions without the plugin spatial attention
as well as with the integration of such an attention
mechanism.
Appendix A
Results of training different backbones of FSAF and
FCOS models over the COCO dataset are mentioned.
Table 6 shows the results of the training FSAF model
with different IoU loss without an attention mecha-
nism in part (a) as well as with an attention mecha-
nism in part (b). Table 7 shows the same but for the
FCOS model.
Appendix B
FSAF and FCOS models based on different back-
bones are investigated with and without spatial atten-
tion mechanism. Those models are trained over a spe-
cific OPEDD dataset. Table 8 and Table 9 show de-
tailed results, which express that using another regres-
sion loss rather than IoU improves the accuracy of the
models.
Investigation of the Performance of Different Loss Function Types Within Deep Neural Anchor-Free Object Detectors
409