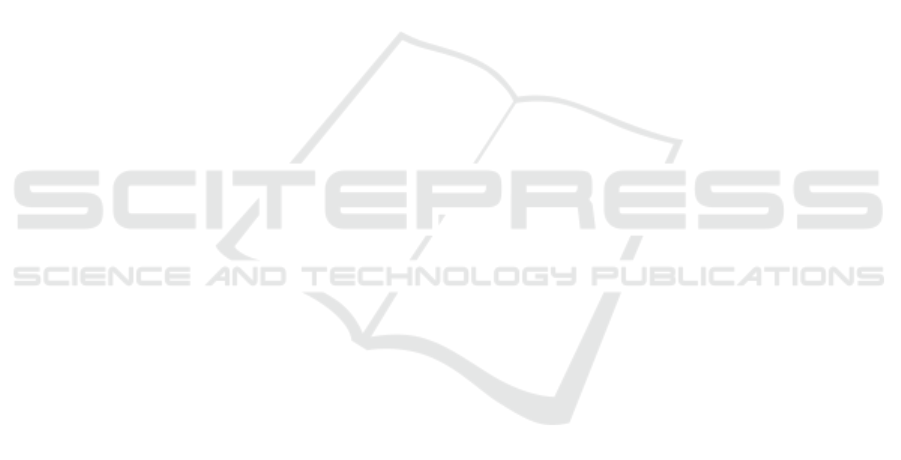
Lee, S. (2023). Look around for anomalies: Weakly-
supervised anomaly detection via context-motion re-
lational learning. In Proceedings of the IEEE/CVF
Conference on Computer Vision and Pattern Recog-
nition, pages 12137–12146.
Georgescu, M.-I., Barbalau, A., Ionescu, R. T., Khan, F. S.,
Popescu, M., and Shah, M. (2021a). Anomaly detec-
tion in video via self-supervised and multi-task learn-
ing. In Proceedings of the IEEE/CVF conference
on computer vision and pattern recognition, pages
12742–12752.
Georgescu, M. I., Ionescu, R. T., Khan, F. S., Popescu, M.,
and Shah, M. (2021b). A background-agnostic frame-
work with adversarial training for abnormal event de-
tection in video. IEEE transactions on pattern analy-
sis and machine intelligence, 44(9):4505–4523.
Guzhov, A., Raue, F., Hees, J., and Dengel, A. (2021). Au-
dioclip: Extending clip to image, text and audio.
Hasan, M., Choi, J., Neumann, J., Roy-Chowdhury, A. K.,
and Davis, L. S. (2016). Learning temporal regularity
in video sequences. In Proceedings of the IEEE con-
ference on computer vision and pattern recognition,
pages 733–742.
Ionescu, R. T., Khan, F. S., Georgescu, M.-I., and Shao,
L. (2019a). Object-centric auto-encoders and dummy
anomalies for abnormal event detection in video. In
Proceedings of the IEEE/CVF Conference on Com-
puter Vision and Pattern Recognition, pages 7842–
7851.
Ionescu, R. T., Smeureanu, S., Popescu, M., and Alexe,
B. (2019b). Detecting abnormal events in video us-
ing narrowed normality clusters. In 2019 IEEE win-
ter conference on applications of computer vision
(WACV), pages 1951–1960. IEEE.
Joo, H. K., Vo, K., Yamazaki, K., and Le, N. (2022). Clip-
tsa: Clip-assisted temporal self-attention for weakly-
supervised video anomaly detection. arXiv preprint
arXiv:2212.05136.
Kingma, D. P. and Ba, J. (2014). Adam: A
method for stochastic optimization. arXiv preprint
arXiv:1412.6980.
Li, M., Xu, R., Wang, S., Zhou, L., Lin, X., Zhu, C., Zeng,
M., Ji, H., and Chang, S.-F. (2022a). Clip-event: Con-
necting text and images with event structures. 2022
IEEE/CVF Conference on Computer Vision and Pat-
tern Recognition (CVPR), pages 16399–16408.
Li, N., Chang, F., and Liu, C. (2022b). A self-trained spatial
graph convolutional network for unsupervised human-
related anomalous event detection in complex scenes.
IEEE Transactions on Cognitive and Developmental
Systems.
Li, N., Wu, X., Guo, H., Xu, D., Ou, Y., and Chen,
Y.-L. (2015). Anomaly detection in video surveil-
lance via gaussian process. International Journal
of Pattern Recognition and Artificial Intelligence,
29(06):1555011.
Liu, K. and Ma, H. (2019). Exploring background-bias
for anomaly detection in surveillance videos. In Pro-
ceedings of the 27th ACM International Conference
on Multimedia, pages 1490–1499.
Liu, W., Luo, W., Lian, D., and Gao, S. (2018a). Fu-
ture frame prediction for anomaly detection–a new
baseline. In Proceedings of the IEEE conference on
computer vision and pattern recognition, pages 6536–
6545.
Liu, W., W. Luo, D. L., and Gao, S. (2018b). Future frame
prediction for anomaly detection – a new baseline. In
2018 IEEE Conference on Computer Vision and Pat-
tern Recognition (CVPR).
Lv, H., Chen, C., Cui, Z., Xu, C., Li, Y., and Yang, J. (2021).
Learning normal dynamics in videos with meta proto-
type network. In Proceedings of the IEEE/CVF con-
ference on computer vision and pattern recognition,
pages 15425–15434.
Medioni, G., Cohen, I., Br
´
emond, F., Hongeng, S., and
Nevatia, R. (2001). Event detection and analysis from
video streams. IEEE Transactions on pattern analysis
and machine intelligence, 23(8):873–889.
Park, H., Noh, J., and Ham, B. (2020). Learning memory-
guided normality for anomaly detection. In Proceed-
ings of the IEEE/CVF conference on computer vision
and pattern recognition, pages 14372–14381.
Piciarelli, C., Micheloni, C., and Foresti, G. L. (2008).
Trajectory-based anomalous event detection. IEEE
Transactions on Circuits and Systems for video Tech-
nology, 18(11):1544–1554.
Purwanto, D., Chen, Y.-T., and Fang, W.-H. (2021). Dance
with self-attention: A new look of conditional random
fields on anomaly detection in videos. In Proceedings
of the IEEE/CVF International Conference on Com-
puter Vision, pages 173–183.
Radford, A., Kim, J. W., Hallacy, C., Ramesh, A., Goh, G.,
Agarwal, S., Sastry, G., Askell, A., Mishkin, P., Clark,
J., et al. (2021). Learning transferable visual models
from natural language supervision. In International
conference on machine learning, pages 8748–8763.
PMLR.
Ravanbakhsh, M., Nabi, M., Sangineto, E., Marcenaro, L.,
Regazzoni, C., and Sebe, N. (2017). Abnormal event
detection in videos using generative adversarial nets.
In 2017 IEEE international conference on image pro-
cessing (ICIP), pages 1577–1581. IEEE.
Sabokrou, M., Fayyaz, M., Fathy, M., and Klette, R. (2017).
Deep-cascade: Cascading 3d deep neural networks for
fast anomaly detection and localization in crowded
scenes. IEEE Transactions on Image Processing,
26(4):1992–2004.
Sch
¨
olkopf, B., Williamson, R. C., Smola, A., Shawe-Taylor,
J., and Platt, J. (1999). Support vector method for nov-
elty detection. Advances in neural information pro-
cessing systems, 12.
Sultani, W., Chen, C., and Shah, M. (2018). Real-world
anomaly detection in surveillance videos. In Proceed-
ings of the IEEE conference on computer vision and
pattern recognition, pages 6479–6488.
Sun, S. and Gong, X. (2023). Hierarchical semantic contrast
for scene-aware video anomaly detection. In Proceed-
ings of the IEEE/CVF Conference on Computer Vision
and Pattern Recognition, pages 22846–22856.
ICPRAM 2024 - 13th International Conference on Pattern Recognition Applications and Methods
532