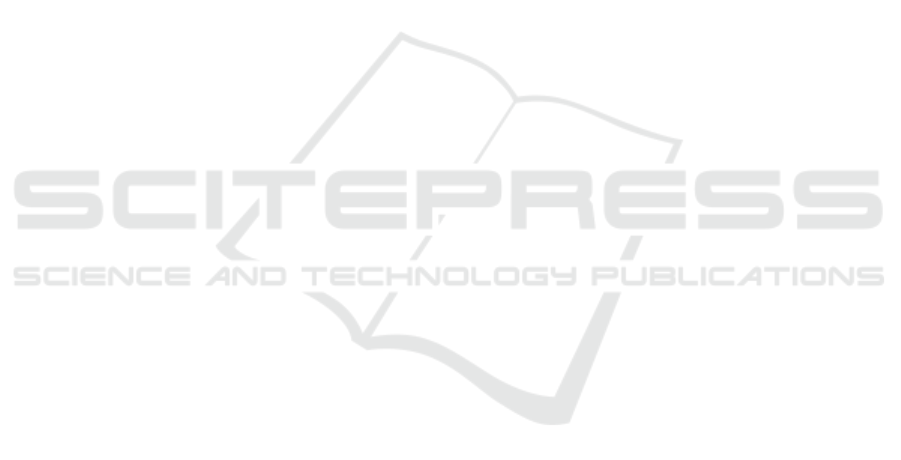
In response to these insights, we propose a do-
main gap reduction process for point clouds. This
process proves its effectiveness through clear quali-
tative enhancements and a substantial reduction in ac-
curacy gaps among various models when comparing
their performance on real and synthetic data. Notably,
this reduction is particularly prominent in the case of
point cloud accumulation, where the CenterPoint and
TransFusion models exhibit accuracy differences that
are reduced by 22.8% and 48.6%, respectively. This
approach can be applied to more reliably validate AD
functions using synthetic point clouds.
This paper, has not investigated the potential of
training models using solely synthetic data or in con-
junction with real data, and it does not assess the con-
tribution of these data in training, with or without pro-
cessing. The exploration of this task is deferred to fu-
ture work. Regarding the domain gap, although it has
been possible to reduce it, it still exists and has not yet
been completely reduced; continuing with the quan-
tification and reduction of the domain gap in point
clouds is still a pending and developing task.
ACKNOWLEDGEMENTS
This work has received funding from Basque Gov-
ernment under project AutoTrust of the program
ELKARTEK-2023. This work is partially supported
by the Ministerio de Ciencia, Innovaci
´
on y Universi-
dades, AEI, MCIN/AEI/10.13039/501100011033.
REFERENCES
Bai, X., Hu, Z., Zhu, X., Huang, Q., and et al. (2022). Trans-
fusion: Robust lidar-camera fusion for 3d object de-
tection with transformers.
Caesar, H., Bankiti, V., Lang, A. H., and et al. (2019).
nuscenes: A multimodal dataset for autonomous driv-
ing.
Contributors, M. (2020). MMDetection3D: OpenMM-
Lab next-generation platform for general 3D ob-
ject detection. https://github.com/open-mmlab/
mmdetection3d.
Dosovitskiy, A., Ros, G., Codevilla, F., Lopez, A., and
Koltun, V. (2017). Carla: An open urban driving sim-
ulator.
Dworak, D., Ciepiela, F., Derbisz, J., Izzat, I., and et al.
(2019). Performance of lidar object detection deep
learning architectures based on artificially generated
point cloud data from carla simulator. In 2019 24th
International Conference on MMAR, pages 600–605.
Huch, S., Scalerandi, L., Rivera, E., and Lienkamp, M.
(2023). Quantifying the lidar sim-to-real domain shift:
A detailed investigation using object detectors and an-
alyzing point clouds at target-level. IEEE Transac-
tions on Intelligent Vehicles, 8(4):2970–2982.
Inan, B. A., Rondao, D., and Aouf, N. (2023). Enhancing
lidar point cloud segmentation with synthetic data. In
2023 31st MED, pages 370–375.
Kalra, N. and Paddock, S. M. (2016). Driving to Safety:
How Many Miles of Driving Would It Take to Demon-
strate Autonomous Vehicle Reliability? RAND Cor-
poration, Santa Monica, CA.
Kloukiniotis, A., Papandreou, A., Anagnostopoulos, C.,
and et al. (2022). Carlascenes: A synthetic dataset
for odometry in autonomous driving. In CVPR Work-
shops, pages 4520–4528.
Lang, A. H., Vora, S., Caesar, H., and et al. (2018). Point-
pillars: Fast encoders for object detection from point
clouds.
Li, Y. and Ibanez-Guzman, J. (2020). Lidar for autonomous
driving: The principles, challenges, and trends for au-
tomotive lidar and perception systems. IEEE Signal
Processing Magazine, 37(4):50–61.
Li, Y., Ma, L., Zhong, Z., and et al. (2021a). Deep learn-
ing for lidar point clouds in autonomous driving: A
review. IEEE Transactions on Neural Networks and
Learning Systems, 32(8):3412–3432.
Li, Y., Ma, L., Zhong, Z., Liu, F., Chapman, M. A., and
et al. (2021b). Deep learning for lidar point clouds
in autonomous driving: A review. IEEE TNNLS,
32(8):3412–3432.
Liao, Y., Xie, J., and Geiger, A. (2021). Kitti-360: A novel
dataset and benchmarks for urban scene understand-
ing in 2d and 3d.
Mart
´
ınez-D
´
ıaz, M. and Soriguera, F. (2018). Autonomous
vehicles: theoretical and practical challenges. Trans-
portation Research Procedia, 33:275–282. CIT2018.
Qiao, D. and Zulkernine, F. (2023). Adaptive feature fusion
for cooperative perception using lidar point clouds. In
Proceedings of the IEEE/CVF WACV, pages 1186–
1195.
Rong, G., Shin, B. H., Tabatabaee, H., Lu, Q., Lemke,
S., and et al. (2020). LGSVL simulator: A high
fidelity simulator for autonomous driving. CoRR,
abs/2005.03778.
Sekkat, A. R., Dupuis, Y., Kumar, V. R., and et al.
(2022). SynWoodScape: Synthetic surround-view
fisheye camera dataset for autonomous driving. IEEE
Robotics and Automation Letters, 7(3):8502–8509.
Sun, P., Kretzschmar, H., and Dotiwalla, Xerxes, e. a.
(2019). Scalability in perception for autonomous driv-
ing: Waymo open dataset.
Wang, F., Zhuang, Y., Gu, H., and Hu, H. (2019). Automatic
generation of synthetic lidar point clouds for 3-d data
analysis. IEEE TIM, 68(7):2671–2673.
Xu, R., Xiang, H., Xia, X., Han, X., and et al. (2022).
Opv2v: An open benchmark dataset and fusion
pipeline for perception with vehicle-to-vehicle com-
munication.
Yin, T., Zhou, X., and Kr
¨
ahenb
¨
uhl, P. (2020). Center-based
3d object detection and tracking. CVPR.
Zhu, X., Ma, Y., Wang, T., Xu, Y., and et al. (2020). Ssn:
Shape signature networks for multi-class object detec-
tion from point clouds.
Analysis of Point Cloud Domain Gap Effects for 3D Object Detection Evaluation
285