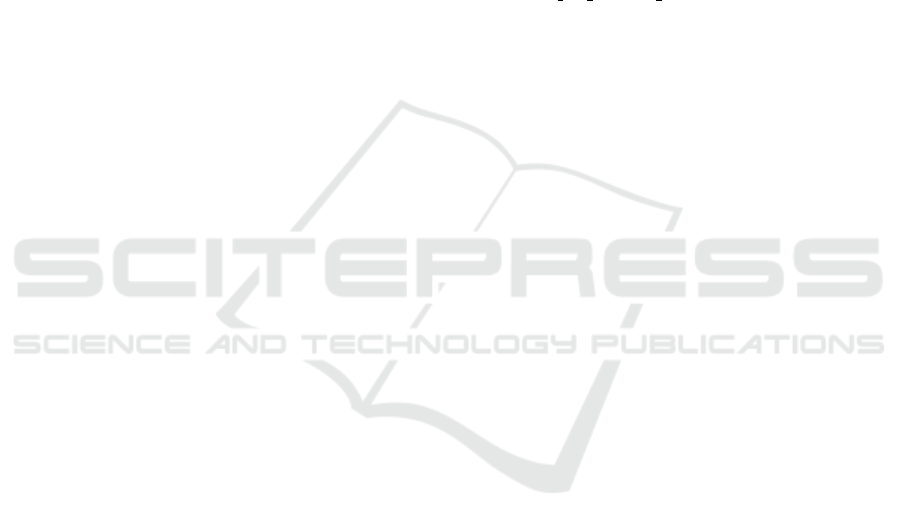
be used in the pre-training, enabling the network to
accurately learn the physical underlying link. Then
the fine-tuning will adapt this learning to the noise of
the real-world data. One of the most obvious candi-
dates to serve as well as a target or input variable is
the sea’s Chlorophyll, which is a passive tracer of the
oceanic currents (Chelton et al., 2011).
Realism of the OSSE. We show that the multivariate
OSSE performed in this study was realistic, as well
for the SSH noise than for the SST noise. However,
given an appropriate transfer strategy, the networks
trained on the noised version of the SST and networks
trained on the ground truth SST achieve similar re-
sults once retrained. This leads us to reconsider the
necessity of computing a very realistic noise on con-
textual information, as the fine-tuning process will get
rid of the learned features that do not appear in real-
world data.
Toward a Global Gridded Image. The experiment
that we performed in this work was focusing on a sin-
gle geographic area. Training a method able to es-
timate SSH on a global scale would require further
work. For instance, as the physical relationship be-
tween SSH and SST depends on latitude, we are cu-
rious to know if a global model would be competitive
compared to several local models.
REFERENCES
Amores, A., Jord
`
a, G., Arsouze, T., and Le Sommer, J.
(2018). Up to what extent can we characterize ocean
eddies using present-day gridded altimetric products?
Journal of Geophysical Research: Oceans, 123:7220–
7236.
Archambault., T., Filoche., A., Charantonis., A., and
B
´
er
´
eziat., D. (2023). Multimodal unsupervised spatio-
temporal interpolation of satellite ocean altimetry
maps. In Proceedings of the 18th International Joint
Conference on Computer Vision, Imaging and Com-
puter Graphics Theory and Applications (VISIGRAPP
2023) - Volume 4: VISAPP, pages 159–167. IN-
STICC, SciTePress.
Archambault, T., Filoche, A., Charantonis, A., B
´
er
´
eziat, D.,
and Thiria, S. (2023). Unsupervised learning of sea
surface height interpolation from multi-variate simu-
lated satellite observations. Submitted to Journal of
Advances of Modeling Earth Systems (JAMES).
Ardhuin, F., Ubelmann, C., Dibarboure, G., Gaultier, L.,
Ponte, A., Ballarotta, M., and Faug
`
ere, Y. (2020). Re-
constructing ocean surface current combining altime-
try and future spaceborne doppler data. Earth and
Space Science Open Archive.
Ballarotta, M., Ubelmann, C., Rog
´
e, M., Fournier, F.,
Faug
`
ere, Y., Dibarboure, G., Morrow, R., and Picot,
N. (2020). Dynamic mapping of along-track ocean al-
timetry: Performance from real observations. Journal
of Atmospheric and Oceanic Technology, 37:1593–
1601.
Bretherton, F., Davis, R., and Fandry, C. (1976). A tech-
nique for objective analysis and design of oceano-
graphic experiments applied to MODE-73. Deep-Sea
Research and Oceanographic Abstracts, 23:559–582.
Che, H., Niu, D., Zang, Z., Cao, Y., and Chen, X. (2022).
Ed-drap: Encoder–decoder deep residual attention
prediction network for radar echoes. IEEE Geoscience
and Remote Sensing Letters, 19.
Chelton, D. B., Gaube, P., Schlax, M. G., Early, J. J., and
Samelson, R. M. (2011). The influence of nonlinear
mesoscale eddies on near-surface oceanic chlorophyll.
Science, 334:328–332.
CLS/MEOM (2020). Swot data challenge natl60 [dataset].
CLS/MEOM (2021). Data challenge ose -
2021a ssh mapping ose [dataset].
CMEMS (2020). Global ocean physics reanalysis [dataset].
CMEMS (2021). Global ocean along-track l3 sea surface
heights reprocessed (1993-ongoing) tailored for data
assimilation [dataset].
Fablet, R., Amar, M., Febvre, Q., Beauchamp, M., and
Chapron, B. (2021). End-to-end physics-informed
representation learning for satellite ocean remote
sensing data: Applications to satellite altimetry and
sea surface currents. ISPRS Annals of the Photogram-
metry, Remote Sensing and Spatial Information Sci-
ences, 5:295–302.
Fablet, R., Febvre, Q., and Chapron, B. (2023). Multimodal
4dvarnets for the reconstruction of sea surface dynam-
ics from sst-ssh synergies. IEEE Transactions on Geo-
science and Remote Sensing, 61.
Filoche, A., Archambault, T., Charantonis, A., and
B
´
er
´
eziat, D. (2022). Statistics-free interpolation of
ocean observations with deep spatio-temporal prior.
In ECML/PKDD Workshop on Machine Learning for
Earth Observation and Prediction (MACLEAN).
Gaultier, L., Ubelmann, C., and Fu, L. (2016). The chal-
lenge of using future SWOT data for oceanic field
reconstruction. Journal of Atmospheric and Oceanic
Technology, 33:119–126.
Guo, M.-H., Xu, T.-X., Liu, J.-J., Liu, Z.-N., Jiang, P.-T.,
Mu, T.-J., Zhang, S.-H., Martin, R. R., Cheng, M.-M.,
and Hu, S.-M. (2021). Attention mechanisms in com-
puter vision: A survey. Computational Visual Media,
8:331–368.
Hinton, G. and Dean, J. (2015). Distilling the knowledge in
a neural network. In NIPS Deep Learning and Repre-
sentation Learning Workshop.
Jam, J., Kendrick, C., Walker, K., Drouard, V., Hsu, J., and
Yap, M. (2021). A comprehensive review of past and
present image inpainting methods. Computer Vision
and Image Understanding, 203.
Kingma, D. P. and Ba, J. (2017). Adam: A method for
stochastic optimization.
Le Guillou, F., Metref, S., Cosme, E., Ubelmann, C., Bal-
larotta, M., Verron, J., and Le Sommer, J. (2020).
Mapping altimetry in the forthcoming SWOT era by
back-and-forth nudging a one-layer quasi-geostrophic
model. Earth and Space Science Open Archive.
VISAPP 2024 - 19th International Conference on Computer Vision Theory and Applications
108