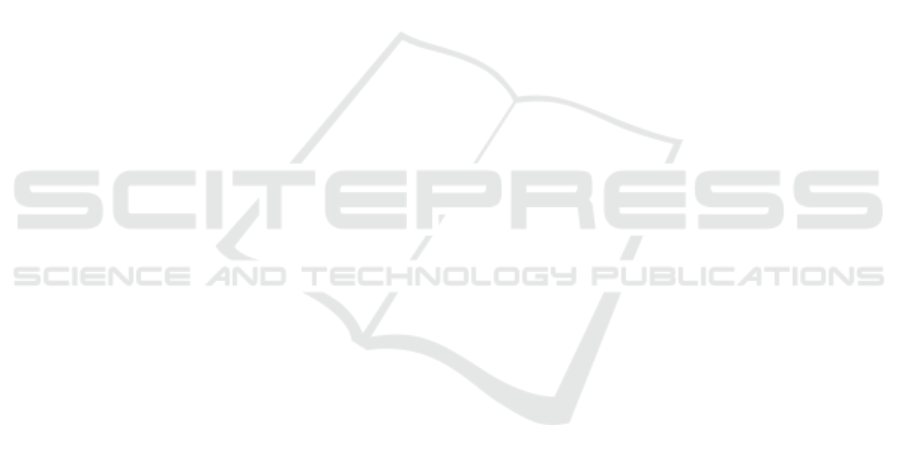
Chiu, D. (2012). Book review: ”pattern classification”, r.
o. duda, p. e. hart and d. g. stork, second edition. In-
ternational Journal of Computational Intelligence and
Applications, 01.
Cruz-Roa, A., Caicedo, J. C., and Gonz
´
alez, F. A. (2011).
Visual pattern mining in histology image collec-
tions using bag of features. Artificial intelligence in
medicine, 52(2):91–106.
do Nascimento, M. Z., Martins, A. S., Azevedo Tosta, T. A.,
and Neves, L. A. (2018). Lymphoma images analysis
using morphological and non-morphological descrip-
tors for classification. Computer Methods and Pro-
grams in Biomedicine, 163:65–77.
Doshi-Velez, F. and Kim, B. (2017). Towards a rigorous
science of interpretable machine learning.
Drelie Gelasca, E., Byun, J., Obara, B., and Manjunath, B.
(2008). Evaluation and benchmark for biological im-
age segmentation. In 2008 15th IEEE International
Conference on Image Processing, pages 1816–1819.
Frank, E., Hall, M. A., and Witten, I. H. (2016). The weka
workbench. online appendix for ”data mining: Practi-
cal machine learning tools and techniques”. Morgan
Kaufmann, Fourth Edition.
He, K., Zhang, X., Ren, S., and Sun, J. (2016). Deep resid-
ual learning for image recognition. In 2016 IEEE Con-
ference on Computer Vision and Pattern Recognition
(CVPR), pages 770–778.
Hsu, H., Hsieh, C. W., and Lu, M. (2011). Hybrid feature
selection by combining filters and wrappers. Expert
Systems with Applications, 38(7):8144–8150.
Iam Palatnik de Sousa, Marley Maria Bernardes Re-
buzzi Vellasco, E. C. d. S. (2019). Local inter-
pretable model-agnostic explanations for classifica-
tion of lymph node metastases. Sensors (Basel,
Switzerland), 19, no. 13.
Ivanovici, M., Richard, N., and Decean, H. (2009a). Fractal
dimension and lacunarity of psoriatic lesions-a colour
approach. medicine, 6(4):7.
Ivanovici, M., Richard, N., and Decean, H. (2009b). Frac-
tal dimension and lacunarity of psoriatic lesions—a
colour approach—. Proceedings of the 2nd WSEAS
International Conference on Biomedical Electronics
and Biomedical Informatics, BEBI ’09, 6.
LeCun, Y., Bengio, Y., and Hinton, G. (2015). Deep learn-
ing. Nature, 521:436–44.
M. Sahini, M. S. (2014). Applications of percolation theory.
CRC Press.
Mahendran, A. and Vedaldi, A. (2016). Visualizing deep
convolutional neural networks using natural preim-
ages. International Journal of Computer Vision.
Mengdi, L., Liancheng, X., Jing, Y., and Jie, H. (2018).
A feature gene selection method based on relieff
and pso. In 2018 10th International Conference on
Measuring Technology and Mechatronics Automation
(ICMTMA), pages 298–301. IEEE.
o. A. AGEMAP, N. I. (2020). The atlas of gene expres-
sion in mouse aging project (agemap). Acesso em:
04/05/2020.
Rajaraman, S., Candemir, S., Kim, I., Thoma, G., and An-
tani, S. (2018). Visualization and interpretation of
convolutional neural network predictions in detecting
pneumonia in pediatric chest radiographs. Applied
Sciences, 8:1715.
Reyes, M., Meier, R., Pereira, S., Silva, C., Dahlweid,
M., Tengg-Kobligk, H., Summers, R., and Wiest, R.
(2020). On the interpretability of artificial intelligence
in radiology: Challenges and opportunities. Radiol-
ogy: Artificial Intelligence, 2:e190043.
Ribeiro, M. G., Neves, L. A., Nascimento, M. Z. d.,
Roberto, G. F., Martins, A. M., and Tosta, T. A. A.
(2019). Classification of colorectal cancer based on
the association of multidimensional and multireso-
lution features. Expert Systems With Applications,
120:262––278.
Robnik-Sikonja, M. and Kononenko, I. (2003). Theoretical
and empirical analysis of relieff and rrelieff. Machine
Learning, 53:23–69.
Sahini, M. and Sahimi, M. (2014). Applications of percola-
tion theory. CRC Press.
Silva, A. B., Martins, A. S., Tosta, T. A. A., Neves, L. A.,
Servato, J. P. S., de Ara
´
ujo, M. S., de Faria, P. R., and
do Nascimento, M. Z. (2022). Computational analysis
of histological images from hematoxylin and eosin-
stained oral epithelial dysplasia tissue sections. Expert
Systems with Applications, 193:116456.
Sirinukunwattana, K., Pluim, J. P., Chen, H., Qi, X., Heng,
P.-A., Guo, Y. B., Wang, L. Y., Matuszewski, B. J.,
Bruni, E., Sanchez, U., et al. (2017). Gland segmen-
tation in colon histology images: The glas challenge
contest. Medical image analysis, 35:489–502.
Suman, R., Mall, R., Sukumaran, S., and Satpathy, M.
(2010). Extracting state models for blackbox software
components. Journal of Object Technology - JOT,
9:79–103.
Szegedy, C., Liu, W., Jia, Y., Sermanet, P., Reed, S.,
Anguelov, D., Erhan, D., Vanhoucke, V., and Rabi-
novich, A. (2015). Going deeper with convolutions.
In Proceedings of the IEEE conference on computer
vision and pattern recognition, pages 1–9.
Yosinski, J., Clune, J., Nguyen, A., Fuchs, T., and Lipson,
H. (2015). Understanding neural networks through
deep visualization.
Zerdoumi, S., Sabri, A. Q. M., Kamsin, A., Hashem, I.
A. T., Gani, A., Hakak, S., Al-Garadi, M. A., and
Chang, V. (2018). Image pattern recognition in big
data: taxonomy and open challenges: survey. Multi-
media Tools and Applications, 77(8):10091–10121.
Association of Grad-CAM, LIME and Multidimensional Fractal Techniques for the Classification of H&E Images
447