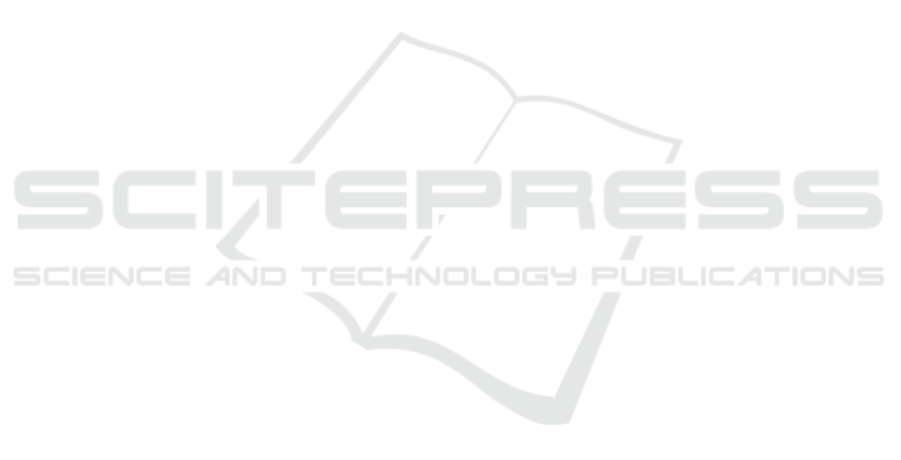
the number of labels available for each configuration
(25%, 50%, 80%).
Resutls of the semi-supervised experiementation
are reported in Figure 2, interestingly, MMFusion
outperforms sensor and touch modality even on a
very limited portion of annotated train set of 25%
(Figure 2.(a)) by showing the best trade-off between
sensitivity and specificity. Moreover, we observe
consistent improvement with more annotated data
available, in the case of 50% and 80% of train la-
bels (Figure 2.(b) and Figure 2.(c)). Regarding Hu-
mIdb database, MMFusion gives the best trade-off
between sensitivity and specificity when it is fine-
tuned on 25% labels of train data (Figure 2.(d)), and
improvement is consistent with more available la-
bels (Figure2.(e) and Figure2.(f)). Unlike sensor and
touch modality, MMFusion shows the best trade-off
in semi-supervised evaluation, both on HMOG and
HumIdb databses, which makes it a feasible solution
when limited annotated data is used for fine-tuning a
self-supervised model.
4 CONCLUSIONS
In this paper a powerful multimodal fusion is pro-
posed within the context of active biometric verifica-
tion on mobile devices. It relies on self-supervised
learning, and combines touch screen and hand move-
ment data collected from mobile users while perform-
ing natural interactions. MMFusion builds strong fea-
ture representations at the contrastive learning level
by leveraging the complementary information of sen-
sor and touch data.
Extensive experiments on two benchmark
databases show that the proposed model outperforms
contrastive learning with SimCLR when applied on
hand movement and touch modality separately. In
addition, during semi-supervised evaluation where
labeled data is very limited, MMFusion gives the
best trade-off compared to hand movement and touch
models. In a future work, we aim to evaluate the
vulnerability of self-supervised models on active
biometric verification and suggest a method to defend
against adversarial attacks.
REFERENCES
Acien, A., Morales, A., Fierrez, J., Vera-Rodriguez, R., and
Delgado-Mohatar, O. (2021). Becaptcha: Behavioral
bot detection using touchscreen and mobile sensors
benchmarked on humidb. Engineering Applications
of Artificial Intelligence, 98:104058.
Chen, T., Kornblith, S., Norouzi, M., and Hinton, G. (2020).
A simple framework for contrastive learning of visual
representations. Vienna, AUSTRIA.
De Marsico, M., Galdi, C., Nappi, M., and Riccio, D.
(2014). Firme: Face and iris recognition for mo-
bile engagement. Image and Vision Computing,
32(12):1161–1172.
Delgado-Santos, P., Tolosana, R., Guest, R., Vera-
Rodriguez, R., Deravi, F., and Morales, A. (2022).
Gaitprivacyon: Privacy-preserving mobile gait bio-
metrics using unsupervised learning. Pattern Recog-
nition Letters, 161:30–37.
Fathy, M. E., Patel, V. M., and Chellappa, R. (2015). Face-
based active authentication on mobile devices. In
2015 IEEE International Conference on Acoustics,
Speech and Signal Processing (ICASSP), pages 1687–
1691.
Giorgi, G., Saracino, A., and Martinelli, F. (2021). Using
recurrent neural networks for continuous authentica-
tion through gait analysis. Pattern Recognition Let-
ters, 147:157–163.
Jing, L. and Tian, Y. (2021). Self-supervised visual feature
learning with deep neural networks: A survey. IEEE
Transactions on Pattern Analysis and Machine Intel-
ligence, 43(11):4037–4058.
Sitov
´
a, Z.,
ˇ
Sed
ˇ
enka, J., Yang, Q., Peng, G., Zhou, G., Gasti,
P., and Balagani, K. S. (2016). Hmog: New behav-
ioral biometric features for continuous authentication
of smartphone users. IEEE Transactions on Informa-
tion Forensics and Security, 11(5):877–892.
Stragapede, G., Vera-Rodriguez, R., Tolosana, R., and
Morales, A. (2023). Behavepassdb: Public database
for mobile behavioral biometrics and benchmark eval-
uation. Pattern Recognition, 134:109089.
Stragapede, G., Vera-Rodriguez, R., Tolosana, R., Morales,
A., Acien, A., and Le Lan, G. (2022). Mobile be-
havioral biometrics for passive authentication. Pattern
Recognition Letters, 157:35–41.
Stylios, I., Kokolakis, S., Thanou, O., and Chatzis, S.
(2021). Behavioral biometrics & continuous user au-
thentication on mobile devices: A survey. Information
Fusion, 66:76–99.
Tolosana, R. and Vera-Rodriguez, R. (2022). Svc-ongoing:
Signature verification competition. Pattern Recogni-
tion, 127:108609.
Tolosana, R., Vera-Rodriguez, R., Fierrez, J., and Ortega-
Garcia, J. (2018). Exploring recurrent neural networks
for on-line handwritten signature biometrics. IEEE
Access, 6:5128–5138.
Zou, Q., Wang, Y., Wang, Q., Zhao, Y., and Li, Q. (2020).
Deep learning-based gait recognition using smart-
phones in the wild. IEEE Transactions on Information
Forensics and Security, 15:3197–3212.
ICPRAM 2024 - 13th International Conference on Pattern Recognition Applications and Methods
744