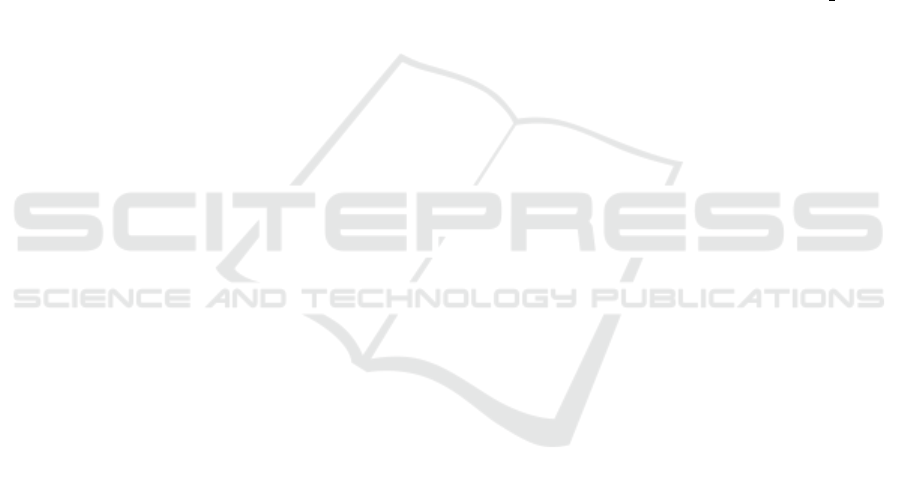
ology consisted of augmenting the dataset and fine-
tuning YOLOv5 and YOLOv8 models using the aug-
mented data. The experimental results showed that
both models effectively detected the olive fruit fly,
but YOLOv8 obtained superior results, in terms of the
evaluation metrics.
In the future, the fine-tuned models, which include
domain knowledge to help improve the methodolo-
gies, are going to be integrated on a web-based infor-
mation and management system, which will receive
the images collected from the field, by a robotic smart
trap developed by our team, and return the image
with the detection results, to aid farmers in making
informed decisions.
ACKNOWLEDGEMENTS
This work is co-financed by Component 5 - Capital-
ization and Business Innovation of core funding for
Technology and Innovation Centres (CTI), integrated
in the Resilience Dimension of the Recovery and Re-
silience Plan within the scope of the Recovery and
Resilience Mechanism (MRR) of the European Union
(EU), framed in the Next Generation EU, for the pe-
riod 2021 - 2026
REFERENCES
Bjerge, K., Mann, H. M., and Høye, T. T. (2022). Real-time
insect tracking and monitoring with computer vision
and deep learning. Remote Sensing in Ecology and
Conservation, 8:315–327.
Caselli, A. and Petacchi, R. (2021). Climate change and
major pests of mediterranean olive orchards: Are we
ready to face the global heating? Insects, 12(9).
Diller, Y., Shamsian, A., Shaked, B., Altman, Y., Danziger,
B. C., Manrakhan, A., Serfontein, L., Bali, E., Wer-
nicke, M., Egartner, A., Colacci, M., Sciarretta, A.,
Chechik, G., Alchanatis, V., Papadopoulos, N. T., and
Nestel, D. (2022). A real-time remote surveillance
system for fruit flies of economic importance: sen-
sitivity and image analysis. Journal of Pest Science,
1:1–12.
Eurostat, E. (2019). Statistics explained. (Accessed on
2/02/2023).
He, K., Zhang, X., Ren, S., and Sun, J. (2014). Spatial
pyramid pooling in deep convolutional networks for
visual recognition. In Fleet, D., Pajdla, T., Schiele, B.,
and Tuytelaars, T., editors, Computer Vision – ECCV
2014, pages 346–361, Cham. Springer International
Publishing.
Hong, S.-J., Kim, S.-Y., Kim, E., Lee, C.-H., Lee, J.-S.,
Lee, D.-S., Bang, J., and Kim, G. (2020). Moth detec-
tion from pheromone trap images using deep learning
object detectors. Agriculture, 10(5):170.
Jocher, G. (2020). YOLOv5 by ultralytics. Version 7.0.
Jocher, G., Chaurasia, A., and Qiu, J. (2023). YOLO by
Ultralytics.
Kalamatianos, R., Karydis, I., Doukakis, D., and Avlonitis,
M. (2018). Dirt: The dacus image recognition toolkit.
Journal of Imaging 2018, Vol. 4, Page 129, 4:129.
Le, A. D., Pham, D. A., Pham, D. T., and Vo, H. B. (2021).
Alerttrap: A study on object detection in remote in-
sects trap monitoring system using on-the-edge deep
learning platform.
Mamdouh, N. and Khattab, A. (2021). Yolo-based deep
learning framework for olive fruit fly detection and
counting. IEEE Access, 9.
Nazir, N., Bilal, S., Bhat, K., Shah, T., Badri, Z., Bhat,
F., Wani, T., Mugal, M., Parveen, S., and Dorjey, S.
(2018). Effect of climate change on plant diseases. In-
ternational Journal of Current Microbiology and Ap-
plied Sciences, 7:250–256.
Pereira, J. A. (2023). Yellow sticky traps dataset
olive fly
(Bactrocera Oleae).
Redmon, J., Divvala, S., Girshick, R., and Farhadi, A.
(2016). You only look once: Unified, real-time ob-
ject detection.
Redmon, J. and Farhadi, A. (2018). Yolov3: An incremental
improvement.
Remboski, T. B., de Souza, W. D., de Aguiar, M. S., and
Ferreira, P. R. (2018). Identification of fruit fly in in-
telligent traps using techniques of digital image pro-
cessing and machine learning. In Proceedings of the
33rd Annual ACM Symposium on Applied Computing,
SAC ’18, page 260–267, New York, NY, USA. Asso-
ciation for Computing Machinery.
Shaked, B., Amore, A., Ioannou, C., Vald
´
es, F., Alorda,
B., Papanastasiou, S., Goldshtein, E., Shenderey, C.,
Leza, M., Pontikakos, C., and et al. (2017). Electronic
traps for detection and population monitoring of adult
fruit flies (diptera: Tephritidae). Journal of Applied
Entomology, 142(1-2):43–51.
Tetila, E. C., MacHado, B. B., Menezes, G. V., Belete, N.
A. D. S., Astolfi, G., and Pistori, H. (2020). A deep-
learning approach for automatic counting of soybean
insect pests. IEEE Geoscience and Remote Sensing
Letters, 17:1837–1841.
Tzutalin (2015). Labelimg.
Uzun, Y., Tolun, M. R., Eyyuboglu, H. T., and Sari, F.
(2022). An intelligent system for detecting mediter-
ranean fruit fly [medfly; ceratitis capitata (wiede-
mann)]. Journal of Agricultural Engineering, 53.
Wang, C.-Y., Liao, H.-Y. M., Yeh, I.-H., Wu, Y.-H., Chen,
P.-Y., and Hsieh, J.-W. (2019). Cspnet: A new back-
bone that can enhance learning capability of cnn.
Wang, K., Liew, J. H., Zou, Y., Zhou, D., and Feng, J.
(2020). Panet: Few-shot image semantic segmenta-
tion with prototype alignment.
Comparative Study Between Object Detection Models, for Olive Fruit Fly Identification
465