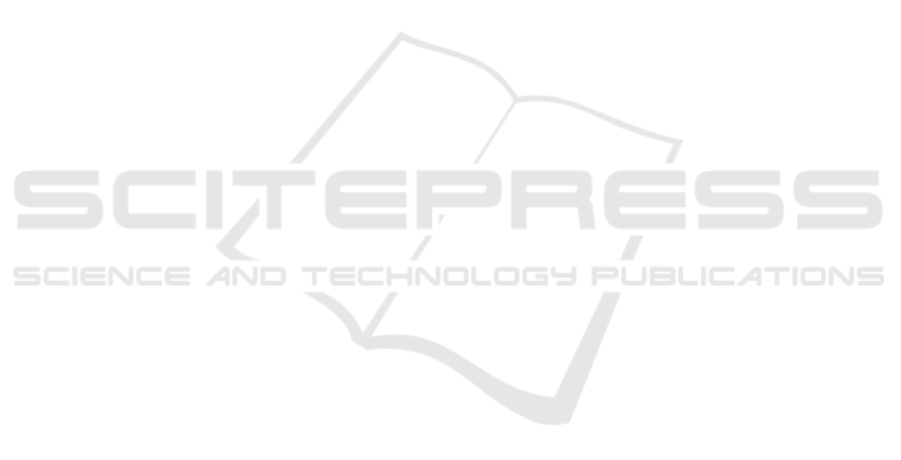
IEEE Transactions on Neural Networks, 18(6):1750–
1761.
Kamozawa, H., Muroga, S., and Tanaka, M. (2023). A
detection method of atrial fibrillation from 24-hour
Holter-ECG using CNN. IEEJ Transactions on Elec-
trical and Electronic Engineering.
Kingma, D. P. and Ba, J. (2014). Adam: A
method for stochastic optimization. arXiv preprint
arXiv:1412.6980.
Kiranyaz, S., Ince, T., and Gabbouj, M. (2017). Personal-
ized monitoring and advance warning system for car-
diac arrhythmias. Scientific reports, 7(1):1–8.
Kramer, O. and Kramer, O. (2016). Scikit-learn. Machine
learning for evolution strategies, pages 45–53.
Krizhevsky, A., Sutskever, I., and Hinton, G. E. (2012). Im-
agenet classification with deep convolutional neural
networks. Advances in neural information processing
systems, 25.
Kumar, V. (2022). Analysis of CNN features with multiple
machine learning classifiers in diagnosis of monkey-
pox from digital skin images. medRxiv, pages 2022–
09.
Li, J., Si, Y., Xu, T., and Jiang, S. (2018). Deep convolu-
tional neural network based ECG classification system
using information fusion and one-hot encoding tech-
niques. Mathematical problems in engineering, 2018.
Mark, R. and Moody, G. (1988). MIT-BIH arrhythmia
database directory. Cambridge: Massachusetts Insti-
tute of Technology.
Martis, R. J., Acharya, U. R., Adeli, H., Prasad, H., Tan,
J. H., Chua, K. C., Too, C. L., Yeo, S. W. J., and Tong,
L. (2014). Computer aided diagnosis of atrial arrhyth-
mia using dimensionality reduction methods on trans-
form domain representation. Biomedical signal pro-
cessing and control, 13:295–305.
Nair, V. and Hinton, G. E. (2010). Rectified linear units
improve restricted boltzmann machines. In Icml.
Ozaltin, O. and Yeniay, O. (2022). A novel proposed CNN–
SVM architecture for ECG scalograms classification.
Soft Computing, pages 1–20.
Sellami, A. and Hwang, H. (2019). A robust deep convo-
lutional neural network with batch-weighted loss for
heartbeat classification. Expert Systems with Applica-
tions, 122:75–84.
Shi, H., Wang, H., Zhang, F., Huang, Y., Zhao, L., and Liu,
C. (2019). Inter-patient heartbeat classification based
on region feature extraction and ensemble classifier.
Biomedical Signal Processing and Control, 51:97–
105.
Souza, I. and Dantas, D. (2023). Cardiac arrhythmia clas-
sification in electrocardiogram signals with convolu-
tional neural networks. In Proceedings of the 12th
International Conference on Pattern Recognition Ap-
plications and Methods. SCITEPRESS - Science and
Technology Publications.
Uchida, K., Tanaka, M., and Okutomi, M. (2018). Cou-
pled convolution layer for convolutional neural net-
work. Neural Networks, 105:197–205.
Wold Health Organization (2023). The top 10 causes of
death. Available at: https://www.who.int/news
-room/fact-sheets/detail/the-top-10-cause
s-of-death. Last accessed: 03 September.
Xiang, Y., Luo, J., Zhu, T., Wang, S., Xiang, X., and Meng,
J. (2018). ECG-based heartbeat classification using
two-level convolutional neural network and rr interval
difference. IEICE TRANSACTIONS on Information
and Systems, 101(4):1189–1198.
Xiong, Z., Stiles, M. K., and Zhao, J. (2017). Robust ECG
signal classification for detection of atrial fibrillation
using a novel neural network. In 2017 Computing in
Cardiology (CinC), pages 1–4. IEEE.
Xu, X. and Liu, H. (2020). ECG heartbeat classification
using convolutional neural networks. IEEE Access,
8:8614–8619.
Yong, P. C., Nordholm, S., Dam, H. H., and Low, S. Y.
(2011). On the optimization of sigmoid function for
speech enhancement. In 2011 19th European Signal
Processing Conference, pages 211–215.
Yu, Y., Si, X., Hu, C., and Zhang, J. (2019). A review of
recurrent neural networks: LSTM cells and network
architectures. Neural computation, 31(7):1235–1270.
Zhai, X. and Tin, C. (2018). Automated ECG classification
using dual heartbeat coupling based on convolutional
neural network. IEEE Access, 6:27465–27472.
ICPRAM 2024 - 13th International Conference on Pattern Recognition Applications and Methods
310