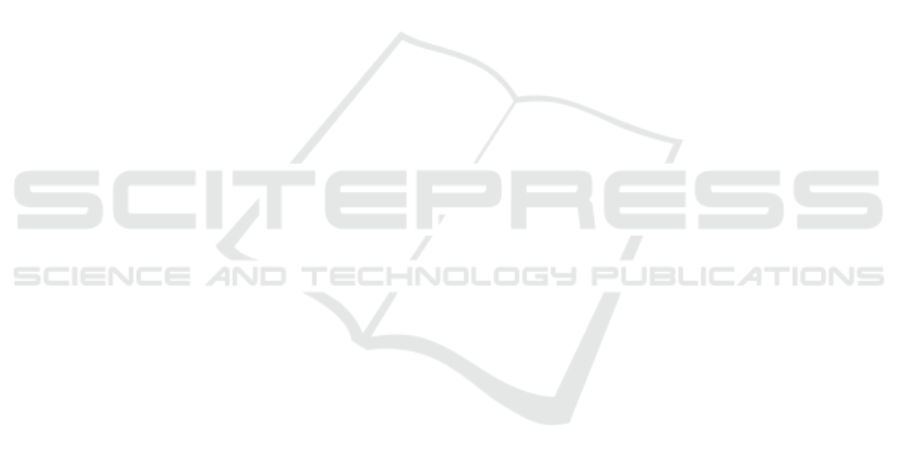
Hong, H., Tsangaratos, P., Ilia, I., Liu, J., Zhu, A.-X., and
Xu, C. (2018). Applying genetic algorithms to set
the optimal combination of forest fire related variables
and model forest fire susceptibility based on data min-
ing models. the case of dayu county, china. Science of
the Total Environment, 630:1044–1056.
Kaur, P., Naik, K., Purcell, R., Sampalli, S., Lung, C.-H.,
Zaman, M., and Mutakabbir, A. (2023). Data inte-
gration framework with multi-source big data for en-
hanced forest fire prediction. Manuscript under re-
view.
Kosovi
´
c, B., Jimenez, P., McCandless, T., Petzke, B.,
Massie, S., Siems-Anderson, A., DeCastro, A.,
Mu
˜
noz-Esparza, D., and Haupt, S. E. (2020). Es-
timation of fuel moisture content by integrating sur-
face and satellite observations using machine learn-
ing. In IGARSS 2020-2020 IEEE International Geo-
science and Remote Sensing Symposium, pages 3626–
3628. IEEE.
Laube, R. and Hamilton, H. J. (2021). Wildfire occurrence
prediction using time series classification: A compar-
ative study. In 2021 IEEE International Conference
on Big Data (Big Data), pages 4178–4182. IEEE.
Lawson, B. D. and Armitage, O. (2008). Weather guide for
the canadian forest fire danger rating system.
Lema
ˆ
ıtre, G., Nogueira, F., and Aridas, C. K. (2017).
Imbalanced-learn: A python toolbox to tackle the
curse of imbalanced datasets in machine learning.
Journal of Machine Learning Research, 18(17):1–5.
Li, Y., Feng, Z., Chen, S., Zhao, Z., and Wang, F. (2020).
Application of the artificial neural network and sup-
port vector machines in forest fire prediction in the
guangxi autonomous region, china. Discrete Dynam-
ics in Nature and Society, 2020:1–14.
Mutakabbir, A., Lung, C.-H., Ajila, S. A., Zaman, M., Naik,
K., Purcell, R., and Sampalli, S. (2023a). Forest fire
prediction using multi-source deep learning. In EAI
BDTA 2023 - 13th EAI International Conference on
Big Data Technologies and Applications (BDTA).
Mutakabbir, A., Lung, C.-H., Ajila, S. A., Zaman, M., Naik,
K., Purcell, R., and Sampalli, S. (2023b). Spatio-
temporal agnostic deep learning modeling of forest
fire prediction using weather data. In 2023 IEEE 47th
Annual Computers, Software, and Applications Con-
ference (COMPSAC), pages 346–351. IEEE.
Natekar, S., Patil, S., Nair, A., and Roychowdhury, S.
(2021). Forest fire prediction using lstm. In 2021
2nd International Conference for Emerging Technol-
ogy (INCET), pages 1–5. IEEE.
Omar, N., Al-zebari, A., and Sengur, A. (2021). Deep learn-
ing approach to predict forest fires using meteorolog-
ical measurements. In 2021 2nd international infor-
matics and software engineering conference (IISEC),
pages 1–4. IEEE.
Preeti, T., Kanakaraddi, S., Beelagi, A., Malagi, S., and
Sudi, A. (2021). Forest fire prediction using machine
learning techniques. In 2021 International Confer-
ence on Intelligent Technologies (CONIT), pages 1–6.
IEEE.
Purcell, R., Naik, K., Sampalli, S., Lung, C.-H., Zaman, M.,
Mutakabbir, A., Kaur, P., and Tavakoli, F. (2023). A
framework for creating forest fire ignition prediction
datasets. Manuscript under review.
Rodriguez-Galiano, V. F., Ghimire, B., Rogan, J., Chica-
Olmo, M., and Rigol-Sanchez, J. P. (2012). An as-
sessment of the effectiveness of a random forest clas-
sifier for land-cover classification. ISPRS journal of
photogrammetry and remote sensing, 67:93–104.
Safi, Y. and Bouroumi, A. (2013). Prediction of forest fires
using artificial neural networks. Applied Mathemati-
cal Sciences, 7(6):271–286.
Saskatchewan Government (2022). Hydrography map
service. Available at https://gis.saskatchewan.ca/
arcgis/rest/services/Hydrography/MapServer. Ac-
cessed: 2022-09-26.
Service, C. F. (2022). Canadian wildland fire information
system (cwfis) datamart. Licensed under the Open
Government Licence - Canada. Available at http://
open.canada.ca/en/open-government-licence-canada.
Singh, B., Kumar, N., and Tiwari, P. (2019). Extreme learn-
ing machine approach for prediction of forest fires us-
ing topographical and metrological data of vietnam.
In 2019 Women Institute of Technology Conference
on Electrical and Computer Engineering (WITCON
ECE), pages 104–112. IEEE.
Statistics Canada (2021). 2021 standard geographical
classification (sgc) - boundaries. Available at
https://www12.statcan.gc.ca/census-recensement/
2021/geo/sip-pis/boundary-limites/index2021-eng.
cfm?year=21. Accessed: 2022-09-26.
Sudhakar, S., Vijayakumar, V., Kumar, C. S., Priya, V.,
Ravi, L., and Subramaniyaswamy, V. (2020). Un-
manned aerial vehicle (uav) based forest fire detection
and monitoring for reducing false alarms in forest-
fires. Computer Communications, 149:1–16.
Suklabaidya, S. and Das, I. (2023). Processing iot sen-
sor fire dataset using machine learning techniques. In
2023 International Conference on Intelligent Systems,
Advanced Computing and Communication (ISACC),
pages 1–7. IEEE.
Tayal, D. K., Agarwal, N., Jha, A., Abrol, V., et al. (2022).
To predict the fire outbreak in australia using histori-
cal database. In 2022 10th International Conference
on Reliability, Infocom Technologies and Optimiza-
tion (Trends and Future Directions)(ICRITO), pages
1–7. IEEE.
Tyukavina, A., Potapov, P., Hansen, M. C., Pickens, A. H.,
Stehman, S. V., Turubanova, S., Parker, D., Zalles, V.,
Lima, A., Kommareddy, I., et al. (2022). Global trends
of forest loss due to fire from 2001 to 2019. Frontiers
in Remote Sensing, 3:825190.
Zope, V., Dadlani, T., Matai, A., Tembhurnikar, P., and
Kalani, R. (2020). Iot sensor and deep neural network
based wildfire prediction system. In 2020 4th Interna-
tional Conference on Intelligent Computing and Con-
trol Systems (ICICCS), pages 205–208. IEEE.
Big Data Synthesis and Class Imbalance Rectification for Enhanced Forest Fire Classification Modeling
275