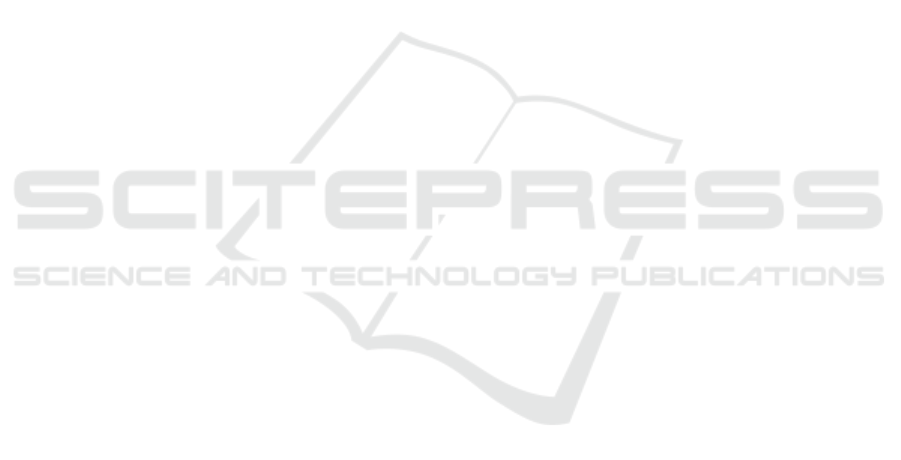
layer MLP accentuated by the Mish activation func-
tion. This deliberate inclusion reduces the parameters
and amplifies pose estimation accuracy—a result we
link to the non-linear attributes of the MLP and the
gradient-rich dynamics of the Mish function. More-
over, the EBA infusion within ResNet34 enhances
our model’s feature extraction capabilities, granting
it deeper contextual insights. By emphasizing pixel-
level discretization, we curtail quantization irregular-
ities and boost joint localization precision. Experi-
mental results produce EBA-PRNetCC superior per-
formance on the COCO dataset, attributed to its re-
fined feature mapping, optimal activation function,
and sophisticated optimization techniques. In the fu-
ture, we aim to adopt increasingly efficient architec-
tures and expand training over varied datasets to en-
hance model generalization.
REFERENCES
Andriluka, M., Pishchulin, L., Gehler, P., and Schiele, B.
(2014). 2d human pose estimation: New bench-
mark and state of the art analysis. In Proceedings of
the IEEE conference on computer vision and pattern
recognition (CVPR).
Cai, Y., Wang, Z., Luo, Z., Yin, B., Du, A., Wang, H.,
Zhang, X., Zhou, X., Zhou, E., and Sun, J. (2020).
Learning delicate local representations for multi-
person pose estimation. In Computer Vision–ECCV
2020: 16th European Conference, Glasgow, UK, Au-
gust 23–28, 2020, Proceedings, Part III, pages 455–
472. Springer.
Cao, Z., Simon, T., Wei, S.-E., and Sheikh, Y. (2017). Real-
time multi-person 2d pose estimation using part affin-
ity fields. Proceedings of the IEEE conference on
computer vision and pattern recognition, pages 7291–
7299.
Chen, H., Feng, R., Wu, S., Xu, H., Zhou, F., and Liu, Z.
(2022). 2d human pose estimation: A survey. Multi-
media Systems, pages 1–24.
Chen, T., Saxena, S., Li, L., Fleet, D. J., and Hinton, G.
(2021). Pix2seq: A language modeling framework for
object detection. arXiv preprint arXiv:2109.10852.
Cheng, B., Xiao, B., Wang, J., Shi, H., Huang, T. S., and
Zhang, L. (2020). Higherhrnet: Scale-aware repre-
sentation learning for bottom-up human pose estima-
tion. In Proceedings of the IEEE/CVF conference on
computer vision and pattern recognition, pages 5386–
5395.
He, K., Zhang, X., Ren, S., and Sun, J. (2016). Deep resid-
ual learning for image recognition. In Proceedings of
the IEEE conference on computer vision and pattern
recognition, pages 770–778.
Johnson, S. and Everingham, M. (2010). Clustered pose and
nonlinear appearance models for human pose estima-
tion. In British Machine Vision Conference (BMVC).
Li, J., Bian, S., Zeng, A., Wang, C., Pang, B., Liu, W., and
Lu, C. (2021). Human pose regression with resid-
ual log-likelihood estimation. In Proceedings of the
IEEE/CVF international conference on computer vi-
sion, pages 11 025–11 034.
Li, Y., Yang, S., Liu, P., Zhang, S., Wang, Y., Wang, Z.,
Yang, W., and Xia, S.-T. (2022). Simcc: A simple
coordinate classification perspective for human pose
estimation. In European Conference on Computer Vi-
sion, pages 89–106. Springer.
Lin, T.-Y., Maire, M., Belongie, S., Hays, J., Perona, P.,
Ramanan, D., Dollar, P., and Zitnick, C. L. (2014).
Microsoft coco: Common objects in context. In Euro-
pean Conference on Computer Vision (ECCV).
Mao, W., Ge, Y., Shen, C., Tian, Z., Wang, X., and Wang,
Z. (2021). Tfpose: Direct human pose estimation with
transformers. arXiv preprint arXiv:2103.15320.
Newell, A., Yang, K., and Deng, J. (2016). Stacked
hourglass networks for human pose estimation. In
Computer Vision–ECCV 2016: 14th European Con-
ference, Amsterdam, The Netherlands, October 11-
14, 2016, Proceedings, Part VIII, pages 483–499.
Springer.
Nie, X., Feng, J., Zhang, J., and Yan, S. (2019). Single-
stage multi-person pose machines. In Proceedings of
the IEEE/CVF international conference on computer
vision, pages 6951–6960.
Salman, S. A., Zakir, A., Benitez-Garcia, G., and Taka-
hashi, H. (2023a). Acenet: Attention-driven contex-
tual features-enhanced lightweight efficientnet for 2d
hand pose estimation. In 2023 38th International Con-
ference on Image and Vision Computing New Zealand
(IVCNZ), pages 1–6. IEEE.
Salman, S. A., Zakir, A., and Takahashi, H. (2023b). Cas-
caded deep graphical convolutional neural network for
2d hand pose estimation. In International Workshop
on Advanced Imaging Technology (IWAIT) 2023, vol-
ume 12592, pages 227–232. SPIE.
Salman, S. A., Zakir, A., and Takahashi, H. (2023c).
Sdfposegraphnet: Spatial deep feature pose graph
network for 2d hand pose estimation. Sensors,
23(22):9088.
Sun, K., Xiao, B., Liu, D., and Wang, J. (2019). Deep high-
resolution representation learning for human pose es-
timation. In Proceedings of the IEEE/CVF conference
on computer vision and pattern recognition, pages
5693–5703.
Tian, Z., Chen, H., and Shen, C. (2019). Directpose: Di-
rect end-to-end multi-person pose estimation. arXiv
preprint arXiv:1911.07451.
Tompson, J. J., Jain, A., LeCun, Y., and Bregler, C. (2014).
Joint training of a convolutional network and a graph-
ical model for human pose estimation. Advances in
neural information processing systems, 27.
Xiao, B., Wu, H., and Wei, Y. (2018). Simple baselines
for human pose estimation and tracking. In Proceed-
ings of the European conference on computer vision
(ECCV), pages 466–481.
Yang, S., Quan, Z., Nie, M., and Yang, W. (2021). Trans-
pose: Keypoint localization via transformer. In Pro-
EBA-PRNetCC: An Efficient Bridge Attention-Integration PoseResNet for Coordinate Classification in 2D Human Pose Estimation
143