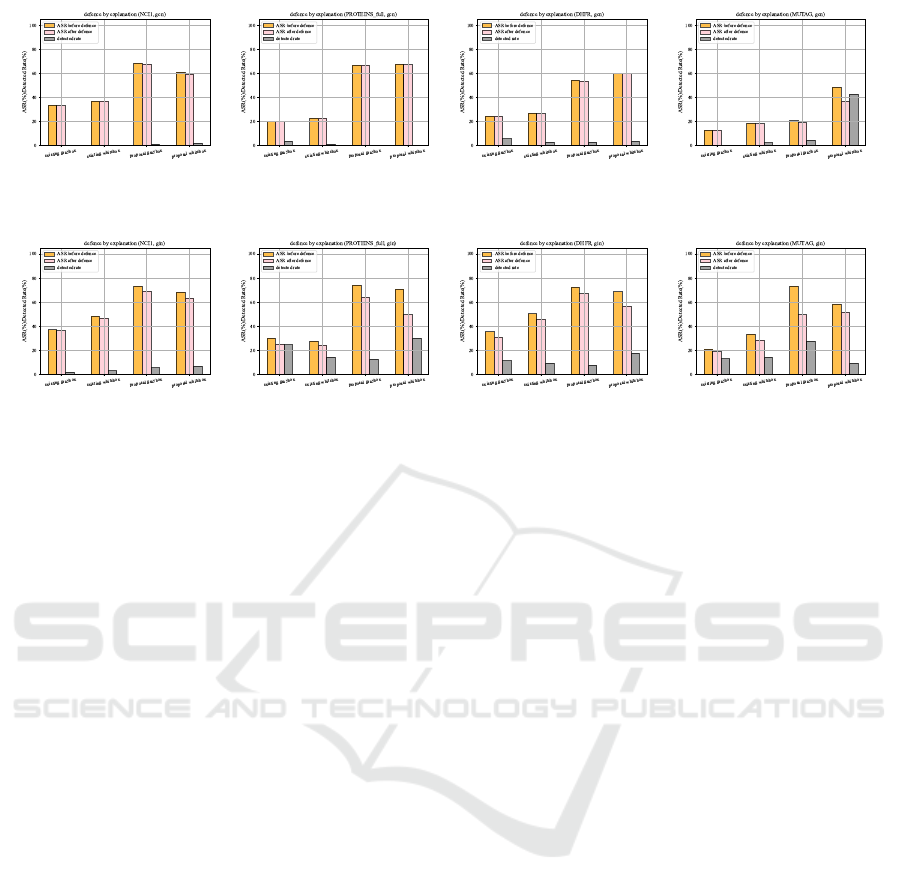
(a) NCI1 (b) PROTEINS (c) DHFR (d) MUTAG
Figure 6: Comparison of Defense results (GCN).
(a) NCI1 (b) PROTEINS (c) DHFR (d) MUTAG
Figure 7: Comparison of Defense results (GIN).
Dobson, P. D. and Doig, A. J. (2003). Distinguishing
enzyme structures from non-enzymes without align-
ments. Journal of molecular biology, 330(4):771–
783.
Erdos, P. (1959). On random graphs. Mathematicae, 6:290–
297.
Feng, P., Ma, J., Li, T., Ma, X., Xi, N., and Lu, D.
(2020). Android malware detection based on call
graph via graph neural network. In 2020 International
Conference on Networking and Network Applications
(NaNA), pages 368–374. IEEE.
Gu, T., Dolan-Gavitt, B., and Garg, S. (2019). Bad-
nets: Identifying vulnerabilities in the machine learn-
ing model supply chain.
Guo, L., Yin, H., Chen, T., Zhang, X., and Zheng, K.
(2021). Hierarchical hyperedge embedding-based
representation learning for group recommendation.
ACM Transactions on Information Systems (TOIS),
40(1):1–27.
Jiang, B. and Li, Z. (2022). Defending against backdoor at-
tack on graph nerual network by explainability. arXiv
preprint arXiv:2209.02902.
Jiang, C., He, Y., Chapman, R., and Wu, H. (2022). Cam-
ouflaged poisoning attack on graph neural networks.
In Proceedings of the 2022 International Conference
on Multimedia Retrieval, pages 451–461.
Kipf, T. N. and Welling, M. (2016). Semi-supervised clas-
sification with graph convolutional networks. arXiv
preprint arXiv:1609.02907.
Kwon, H., Yoon, H., and Park, K.-W. (2019). Selec-
tive poisoning attack on deep neural network to in-
duce fine-grained recognition error. In 2019 IEEE
Second International Conference on Artificial Intel-
ligence and Knowledge Engineering (AIKE), pages
136–139. IEEE.
Li, Y., Li, Y., Wu, B., Li, L., He, R., and Lyu, S. (2021). In-
visible backdoor attack with sample-specific triggers.
In Proceedings of the IEEE/CVF International Con-
ference on Computer Vision (ICCV), pages 16463–
16472.
Liao, C., Zhong, H., Squicciarini, A., Zhu, S., and Miller, D.
(2018). Backdoor embedding in convolutional neural
network models via invisible perturbation.
Liu, Y., Ma, S., Aafer, Y., Lee, W.-C., Zhai, J., Wang, W.,
and Zhang, X. (2018). Trojaning attack on neural net-
works. In 25th Annual Network And Distributed Sys-
tem Security Symposium (NDSS 2018). Internet Soc.
Morris, C., Kriege, N. M., Bause, F., Kersting, K., Mutzel,
P., and Neumann, M. (2020). Tudataset: A collec-
tion of benchmark datasets for learning with graphs.
In ICML 2020 Workshop on Graph Representation
Learning and Beyond (GRL+ 2020).
Qiu, R., Huang, Z., Li, J., and Yin, H. (2020). Exploiting
cross-session information for session-based recom-
mendation with graph neural networks. ACM Trans-
actions on Information Systems (TOIS), 38(3):1–23.
Wale, N., Watson, I. A., and Karypis, G. (2008). Compar-
ison of descriptor spaces for chemical compound re-
trieval and classification. Knowledge and Information
Systems, 14:347–375.
Wang, B., Yao, Y., Shan, S., Li, H., Viswanath, B., Zheng,
H., and Zhao, B. Y. (2019). Neural cleanse: Identi-
fying and mitigating backdoor attacks in neural net-
works. In 2019 IEEE Symposium on Security and Pri-
vacy (SP), pages 707–723.
Xi, Z., Pang, R., Ji, S., and Wang, T. (2021). Graph back-
door. In 30th USENIX Security Symposium (USENIX
Security 21), pages 1523–1540.
Xu, J. and Picek, S. (2022). Poster: Clean-label backdoor
attack on graph neural networks. In Proceedings of
the 2022 ACM SIGSAC Conference on Computer and
Communications Security, pages 3491–3493.
Xu, K., Hu, W., Leskovec, J., and Jegelka, S. (2018). How
powerful are graph neural networks? arXiv preprint
arXiv:1810.00826.
ICISSP 2024 - 10th International Conference on Information Systems Security and Privacy
520