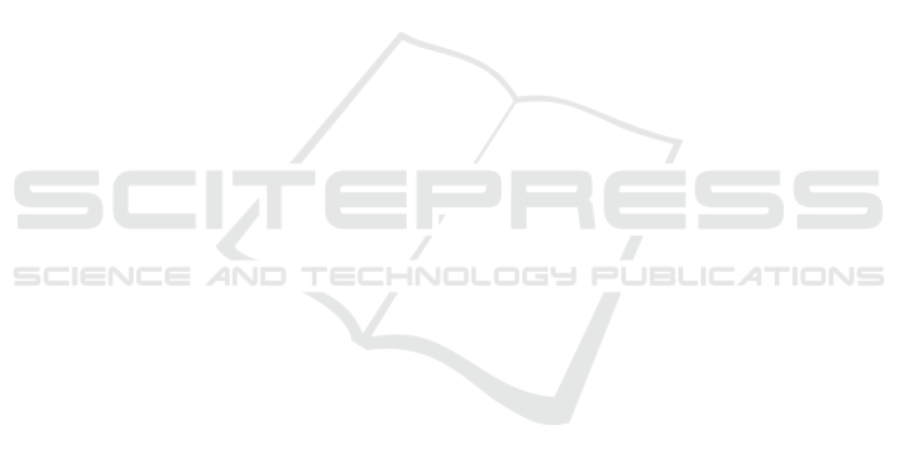
Figure 6 shows the 2D and 3D target reconstruc-
tions errors for the different noise levels. We can see
our method outperforming (El Natour et al., 2015a)
in for all levels of noise in 3D reconstruction. For 2D
reconstruction, all three methods show similar recon-
struction error for level −0 noise, however, (El Natour
et al., 2015a) quickly diverges after level − 4 noise.
This experiment was repeated 250 times for all noise
levels, and show our methods’ robustness to noise.
5.4.4 Ablation Study of the Elevation Constraint
To highlight the importance of our elevation con-
straint, defined in Equation (13), we ran an ablation
study on both of our range calculation methods. This
was done using the Best initialization parameters as
described in Equation (17) and the results can be seen
in Table 2. The mean errors achieved without using
the elevation constraint are considerably higher than
the results achieved when including it. The errors
without the constraint in Equation (13) are also close
to the results of (El Natour et al., 2015a) as seen in Ta-
ble 1. This is expected because the main difference
between them is the distance calculation method. We
observe that before adding the constraint, our method
using the radar range for distance measurement per-
formed better than the method using the camera cor-
respondences, this result was reversed after adding the
constraint.
6 CONCLUSION
In this work, we introduced a new method for extrin-
sic calibration of a camera-radar system. The method
was tested against a high-accuracy motion capture
system, which served as the ground truth. Our setup
is not only simpler, as it operates independently with-
out external sensing, but it also delivers superior re-
sults. Even with less accurate initial parameters and
fewer measurement points, the additional optimiza-
tion constraints we introduced allow the calibration
to converge effectively. We also utilized the calibra-
tion output to reconstruct the 3D targets from the data
matched by the camera-radar system. Instead of more
complicated target designs, our streamlined setup re-
quires fewer calibration targets and merely uses a
single standard retroreflector. While our current ap-
proach only focuses on static targets, calibrating on
a moving target would likely yield better radar target
detection. However, this would come at the cost of
complicating the process, including the setup and tar-
get detection phases of our work.
ACKNOWLEDGMENT
This work has been partially funded by the Ger-
man Ministry of Education and Research (BMBF) of
the Federal Republic of Germany under the research
project RACKET (Grant number 01IW20009). Spe-
cial thanks to Stephan Krauß, Narek Minaskan, and
Alain Pagani for their insightful remarks and discus-
sions.
REFERENCES
Bian, J., Lin, W.-Y., Matsushita, Y., Yeung, S.-K., Nguyen,
T.-D., and Cheng, M.-M. (2017). Gms: Grid-based
motion statistics for fast, ultra-robust feature cor-
respondence. In Conference on Computer Vision
and Pattern Recognition (CVPR), pages 4181–4190.
IEEE.
Chavez-Garcia, R. O. and Aycard, O. (2015). Multiple sen-
sor fusion and classification for moving object detec-
tion and tracking. IEEE Transactions on Intelligent
Transportation Systems, 17(2):525–534.
Cho, H., Seo, Y.-W., Kumar, B. V., and Rajkumar, R. R.
(2014). A multi-sensor fusion system for moving ob-
ject detection and tracking in urban driving environ-
ments. In International Conference on Robotics and
Automation (ICRA), pages 1836–1843. IEEE.
Domhof, J., Kooij, J. F., and Gavrila, D. M. (2019). An
extrinsic calibration tool for radar, camera and lidar. In
International Conference on Robotics and Automation
(ICRA), pages 8107–8113. IEEE.
El Natour, G., Aider, O. A., Rouveure, R., Berry, F., and
Faure, P. (2015a). Radar and vision sensors calibration
for outdoor 3d reconstruction. In International Con-
ference on Robotics and Automation (ICRA), pages
2084–2089. IEEE.
El Natour, G., Ait-Aider, O., Rouveure, R., Berry, F., and
Faure, P. (2015b). Toward 3d reconstruction of out-
door scenes using an mmw radar and a monocular vi-
sion sensor. Sensors, 15(10):25937–25967.
Kim, D. Y. and Jeon, M. (2014). Data fusion of radar
and image measurements for multi-object tracking via
kalman filtering. Information Sciences, 278:641–652.
Lepetit, V., Moreno-Noguer, F., and Fua, P. (2009). Epnp:
An accurate o (n) solution to the pnp problem. Inter-
national journal of computer vision, 81(2):155–166.
Lucas, B. D. and Kanade, T. (1981). An iterative image
registration technique with an application to stereo vi-
sion. In Proceedings of the 7th international joint con-
ference on Artificial intelligence (IJCAI), volume 2,
pages 674–679.
Mor
´
e, J. J. (1978). The levenberg-marquardt algorithm: im-
plementation and theory. In Numerical analysis, pages
105–116. Springer.
Oh, J., Kim, K.-S., Park, M., and Kim, S. (2018). A com-
parative study on camera-radar calibration methods.
In International Conference on Control, Automation,
Robotics and Vision (ICARCV), pages 1057–1062.
IEEE.
ICPRAM 2024 - 13th International Conference on Pattern Recognition Applications and Methods
542