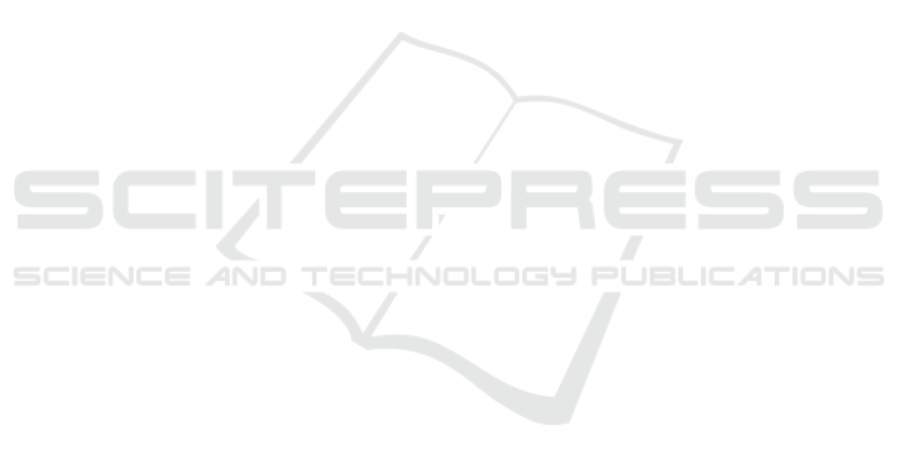
Carion, N., Massa, F., Synnaeve, G., Usunier, N., Kirillov,
A., and Zagoruyko, S. (2020). End-to-end object de-
tection with transformers. In European conference on
computer vision, pages 213–229. Springer.
Chen, A. T.-Y., Kevin, I., and Wang, K. (2016). Computer
vision based chess playing capabilities for the baxter
humanoid robot. In 2016 2nd International Confer-
ence on Control, Automation and Robotics (ICCAR),
pages 11–14. IEEE.
Chen, A. T.-Y. and Wang, K. I.-K. (2019). Robust computer
vision chess analysis and interaction with a humanoid
robot. Computers, 8(1):14.
Community, B. O. (2018). Blender - a 3D modelling and
rendering package. Blender Foundation, Stichting
Blender Foundation, Amsterdam.
Czyzewski, M. A., Laskowski, A., and Wasik, S. (2020).
Chessboard and chess piece recognition with the sup-
port of neural networks. Foundations of Computing
and Decision Sciences, 45(4):257–280.
Danner, C. and Kafafy, M. (2015). Visual chess recognition.
In EE 368, Spring 2015.
Ding, J. (2016). Chessvision: Chess board and piece recog-
nition. In Tech. rep. Stanford University.
Karaoglu, S., Tao, R., van Gemert, J. C., and Gevers, T.
(2017). Con-text: Text detection for fine-grained ob-
ject classification. IEEE transactions on image pro-
cessing, 26(8):3965–3980.
Kingma, D. P. and Ba, J. (2014). Adam: A
method for stochastic optimization. arXiv preprint
arXiv:1412.6980.
Kołosowski, P., Wolniakowski, A., and Miatliuk, K. (2020).
Collaborative robot system for playing chess. In 2020
International Conference Mechatronic Systems and
Materials (MSM), pages 1–6. IEEE.
Koray, C., Sumer, E., Struc, V., et al. (2016). A computer
vision system for chess game tracking. In 21st Com-
puter Vision Winter Workshop, Rimske Toplice, Slove-
nia.
Loshchilov, I. and Hutter, F. (2017). Decoupled weight de-
cay regularization. arXiv preprint arXiv:1711.05101.
Matuszek, C., Mayton, B., Aimi, R., Deisenroth, M. P., Bo,
L., Chu, R., Kung, M., LeGrand, L., Smith, J. R.,
and Fox, D. (2011). Gambit: An autonomous chess-
playing robotic system. In 2011 IEEE International
Conference on Robotics and Automation, pages 4291–
4297. IEEE.
Mehta, A. (2020). Augmented reality chess analyzer
(archessanalyzer): In-device inference of physical
chess game positions through board segmentation and
piece recognition using convolutional neural network.
arXiv preprint arXiv:2009.01649.
Neto, A. d. S. D. and Campello, R. M. (2019). Chess posi-
tion identification using pieces classification based on
synthetic images generation and deep neural network
fine-tuning. In 2019 21st Symposium on Virtual and
Augmented Reality (SVR), pages 152–160. IEEE.
Neufeld, J. E. and Hall, T. S. (2010). Probabilistic location
of a populated chessboard using computer vision. In
2010 53rd IEEE International Midwest Symposium on
Circuits and Systems, pages 616–619. IEEE.
Quintana, D. M., Garc
´
ıa, A. A. d. B., and Mat
´
ıas, M. P.
(2020). Livechess2fen: A framework for classi-
fying chess pieces based on cnns. arXiv preprint
arXiv:2012.06858.
Redmon, J., Divvala, S., Girshick, R., and Farhadi, A.
(2016). You only look once: Unified, real-time object
detection. In Proceedings of the IEEE conference on
computer vision and pattern recognition, pages 779–
788.
Ren, S., He, K., Girshick, R., and Sun, J. (2015). Faster
r-cnn: Towards real-time object detection with region
proposal networks. Advances in neural information
processing systems, 28.
Sokic, E. and Ahic-Djokic, M. (2008). Simple computer vi-
sion system for chess playing robot manipulator as a
project-based learning example. In 2008 IEEE Inter-
national Symposium on Signal Processing and Infor-
mation Technology, pages 75–79. IEEE.
Tam, K., Lay, J., and Levy, D. (2008). Automatic grid seg-
mentation of populated chessboard taken at a lower
angle view. In 2008 Digital Image Computing: Tech-
niques and Applications, pages 294–299.
Urting, D. and Berbers, Y. (2003). Marineblue: A low-cost
chess robot. In Robotics and Applications, pages 76–
81.
Van Gemert, J. C. (2011). Exploiting photographic style for
category-level image classification by generalizing the
spatial pyramid. In Proceedings of the 1st ACM Inter-
national Conference on Multimedia Retrieval, pages
1–8.
Wang, V. and Green, R. (2013). Chess move tracking us-
ing overhead rgb webcam. In 2013 28th Interna-
tional Conference on Image and Vision Computing
New Zealand (IVCNZ 2013), pages 299–304. IEEE.
Wei, Y.-A., Huang, T.-W., Chen, H.-T., and Liu, J. (2017).
Chess recognition from a single depth image. In 2017
IEEE International Conference on Multimedia and
Expo (ICME), pages 931–936. IEEE.
W
¨
olflein, G. and Arandjelovic, O. (2021). Dataset of ren-
dered chess game state images. Open Science Frame-
work.
W
¨
olflein, G. and Arandjelovi
´
c, O. (2021). Determining
chess game state from an image. Journal of Imaging,
7(6):94.
Xie, S., Girshick, R., Doll
´
ar, P., Tu, Z., and He, K. (2017).
Aggregated residual transformations for deep neural
networks. In Proceedings of the IEEE conference on
computer vision and pattern recognition, pages 1492–
1500.
Xie, Y., Tang, G., and Hoff, W. (2018a). Chess piece
recognition using oriented chamfer matching with a
comparison to cnn. In 2018 IEEE Winter Conference
on Applications of Computer Vision (WACV), pages
2001–2009. IEEE.
Xie, Y., Tang, G., and Hoff, W. (2018b). Geometry-based
populated chessboard recognition. In Tenth Interna-
tional Conference on Machine Vision (ICMV 2017),
volume 10696, pages 9–13. SPIE.
Zhu, X., Su, W., Lu, L., Li, B., Wang, X., and Dai, J.
(2020). Deformable detr: Deformable transform-
ers for end-to-end object detection. arXiv preprint
arXiv:2010.04159.
VISAPP 2024 - 19th International Conference on Computer Vision Theory and Applications
402