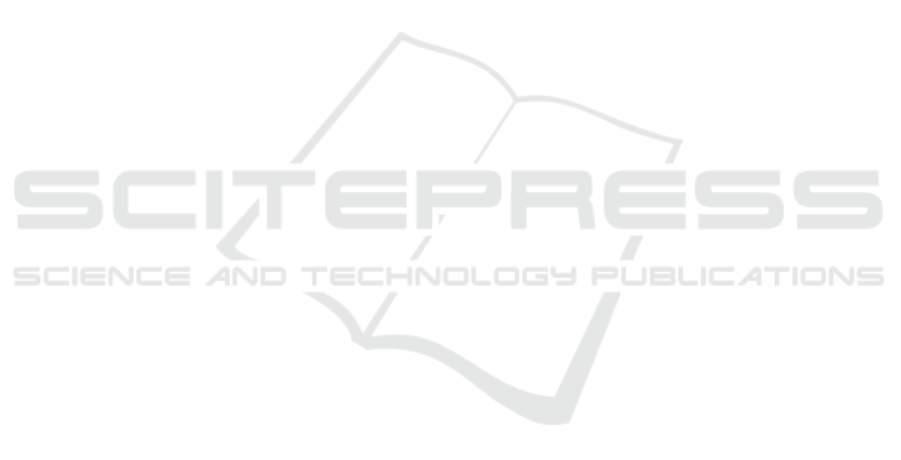
ACKNOWLEDGEMENTS
This work has partly been funded by the Ger-
man Research Foundation (project 3DIL, grant
no. 502864329) and the German Federal Ministry
of Education and Research (project VoluProf, grant
no. 16SV8705).
REFERENCES
Abdal, R., Qin, Y., and Wonka, P. (2019). Im-
age2StyleGAN: How to Embed Images Into the Style-
GAN Latent Space? In 2019 IEEE/CVF International
Conference on Computer Vision (ICCV), pages 4431–
4440, Seoul, Korea (South). IEEE.
Abdal, R., Qin, Y., and Wonka, P. (2020). Im-
age2stylegan++: How to edit the embedded images?
In 2020 IEEE/CVF Conference on Computer Vision
and Pattern Recognition (CVPR), pages 8293–8302.
Abdal, R., Zhu, P., Mitra, N. J., and Wonka, P.
(2021). Styleflow: Attribute-conditioned exploration
of stylegan-generated images using conditional con-
tinuous normalizing flows. ACM Trans. Graph., 40(3).
An, S., Xu, H., Shi, Y., Song, G., Ogras, U. Y., and Luo,
L. (2023). Panohead: Geometry-aware 3d full-head
synthesis in 360deg. In Proceedings of the IEEE/CVF
Conference on Computer Vision and Pattern Recogni-
tion (CVPR), pages 20950–20959.
Barron, J. T., Mildenhall, B., Tancik, M., Hedman, P.,
Martin-Brualla, R., and Srinivasan, P. P. (2021). Mip-
nerf: A multiscale representation for anti-aliasing
neural radiance fields.
Bau, D., Zhu, J.-Y., Wulff, J., Peebles, W., Strobelt, H.,
Zhou, B., and Torralba, A. (2019a). INVERTING
LAYERS OF A LARGE GENERATOR.
Bau, D., Zhu, J.-Y., Wulff, J., Peebles, W., Zhou, B., Stro-
belt, H., and Torralba, A. (2019b). Seeing what a gan
cannot generate. pages 4501–4510.
Bhattarai, A. R., Nießner, M., and Sevastopolsky, A.
(2023). TriPlaneNet: An Encoder for EG3D Inver-
sion. arXiv:2303.13497 [cs].
Brehm, S., Barthel, F., and Lienhart, R. (2022). Control-
ling 3D Objects in 2D Image Synthesis. SN Computer
Science, 4(1):48.
Chan, E., Monteiro, M., Kellnhofer, P., Wu, J., and Wet-
zstein, G. (2020). pi-gan: Periodic implicit generative
adversarial networks for 3d-aware image synthesis. In
arXiv.
Chan, E. R., Lin, C. Z., Chan, M. A., Nagano, K., Pan,
B., Mello, S. D., Gallo, O., Guibas, L., Tremblay, J.,
Khamis, S., Karras, T., and Wetzstein, G. (2021). Ef-
ficient geometry-aware 3D generative adversarial net-
works. In arXiv.
Colloff, M. F., Flowe, H. D., Smith, H. M. J., Seale-Carlisle,
T. M., Meissner, C. A., Rockey, J. C., Pande, B., Ku-
jur, P., Parveen, N., Chandel, P., Singh, M. M., Prad-
han, S., and Parganiha, A. (2022). Active exploration
of faces in police lineups increases discrimination ac-
curacy. American Psychologist, 77(2):196–220.
Deng, J., Guo, J., Xue, N., and Zafeiriou, S. (2019a). Ar-
cface: Additive angular margin loss for deep face
recognition. In 2019 IEEE/CVF Conference on Com-
puter Vision and Pattern Recognition (CVPR), pages
4685–4694.
Deng, Y., Yang, J., Xu, S., Chen, D., Jia, Y., and Tong,
X. (2019b). Accurate 3d face reconstruction with
weakly-supervised learning: From single image to im-
age set. In IEEE Computer Vision and Pattern Recog-
nition Workshops.
Deshmukh, T. and Bhat, M. (2022). GAN inversion of high-
resolution images. Journal of Innovative Image Pro-
cessing, 4(2):103–114.
Gu, J., Liu, L., Wang, P., and Theobalt, C. (2021). Stylenerf:
A style-based 3d-aware generator for high-resolution
image synthesis.
H
¨
ark
¨
onen, E., Hertzmann, A., Lehtinen, J., and Paris, S.
(2020). Ganspace: Discovering interpretable gan con-
trols. In Proc. NeurIPS.
Karras, T., Aila, T., Laine, S., and Lehtinen, J. (2018). Pro-
gressive growing of GANs for improved quality, sta-
bility, and variation. In International Conference on
Learning Representations.
Karras, T., Aittala, M., Laine, S., H
¨
ark
¨
onen, E., Hellsten, J.,
Lehtinen, J., and Aila, T. (2021). Alias-free generative
adversarial networks. In Proc. NeurIPS.
Karras, T., Laine, S., and Aila, T. (2019). A Style-
Based Generator Architecture for Generative Adver-
sarial Networks. arXiv:1812.04948 [cs, stat].
Karras, T., Laine, S., Aittala, M., Hellsten, J., Lehtinen, J.,
and Aila, T. (2020). Analyzing and Improving the Im-
age Quality of StyleGAN. In 2020 IEEE/CVF Con-
ference on Computer Vision and Pattern Recognition
(CVPR), pages 8107–8116, Seattle, WA, USA. IEEE.
Niemeyer, M. and Geiger, A. (2021). GIRAFFE: Represent-
ing Scenes as Compositional Generative Neural Fea-
ture Fields. In 2021 IEEE/CVF Conference on Com-
puter Vision and Pattern Recognition (CVPR), pages
11448–11459, Nashville, TN, USA. IEEE.
Pan, X., Tewari, A., Leimk
¨
uhler, T., Liu, L., Meka, A.,
and Theobalt, C. (2023). Drag Your GAN: Inter-
active Point-based Manipulation on the Generative
Image Manifold. Special Interest Group on Com-
puter Graphics and Interactive Techniques Confer-
ence Conference Proceedings, pages 1–11. Confer-
ence Name: SIGGRAPH ’23: Special Interest Group
on Computer Graphics and Interactive Techniques
Conference ISBN: 9798400701597 Place: Los Ange-
les CA USA Publisher: ACM.
Park, K., Sinha, U., Barron, J. T., Bouaziz, S., Goldman,
D. B., Seitz, S. M., and Martin-Brualla, R. (2021a).
Nerfies: Deformable neural radiance fields. ICCV.
Park, K., Sinha, U., Hedman, P., Barron, J. T., Bouaziz, S.,
Goldman, D. B., Martin-Brualla, R., and Seitz, S. M.
(2021b). Hypernerf: A higher-dimensional represen-
tation for topologically varying neural radiance fields.
ACM Trans. Graph., 40(6).
VISAPP 2024 - 19th International Conference on Computer Vision Theory and Applications
202