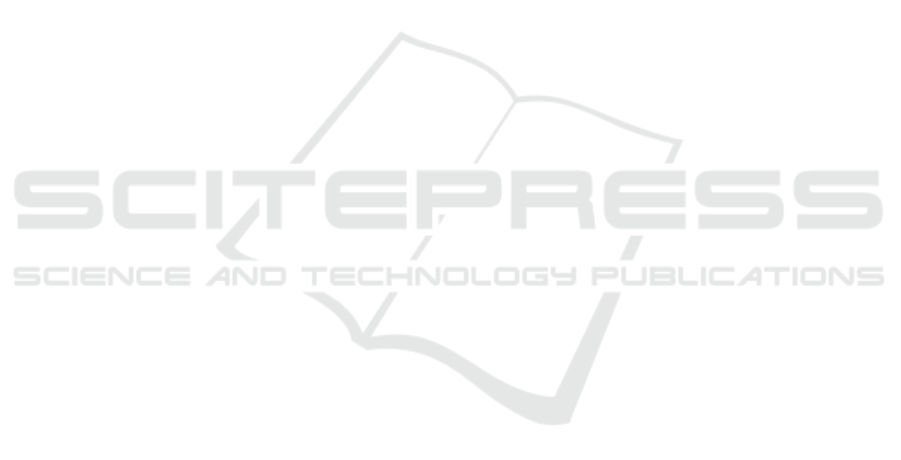
ods for autonomous driving. IEEE Transactions on
Intelligent Vehicles, 7(3):652–674.
Huang, Z., Mo, X., and Lv, C. (2022b). Multi-modal mo-
tion prediction with transformer-based neural network
for autonomous driving. In 2022 International Con-
ference on Robotics and Automation (ICRA), pages
2605–2611. IEEE.
Ivanovic, B. and Pavone, M. (2019). The trajectron:
Probabilistic multi-agent trajectory modeling with dy-
namic spatiotemporal graphs. In Proceedings of the
IEEE/CVF International Conference on Computer Vi-
sion, pages 2375–2384.
Kalman, R. E. (1960). A New Approach to Linear Filtering
and Prediction Problems. Journal of Basic Engineer-
ing, 82(1):35–45.
Kingma, D. P. and Ba, J. (2014). Adam: A
method for stochastic optimization. arXiv preprint
arXiv:1412.6980.
Kosaraju, V., Sadeghian, A., Mart
´
ın-Mart
´
ın, R., Reid, I.,
Rezatofighi, H., and Savarese, S. (2019). Social-bigat:
Multimodal trajectory forecasting using bicycle-gan
and graph attention networks. Advances in Neural In-
formation Processing Systems, 32.
Liu, D., Li, Q., Li, S., Kong, J., and Qi, M. (2023).
Non-autoregressive sparse transformer networks for
pedestrian trajectory prediction. Applied Sciences,
13(5):3296.
Liu, Y., Zhang, J., Fang, L., Jiang, Q., and Zhou, B.
(2021). Multimodal motion prediction with stacked
transformers. In Proceedings of the IEEE/CVF Con-
ference on Computer Vision and Pattern Recognition,
pages 7577–7586.
Lombardo, E., Rabe, M., Xiong, Y., Nierer, L., Cusumano,
D., Placidi, L., Boldrini, L., Corradini, S., Niyazi,
M., Belka, C., et al. (2022). Offline and online
lstm networks for respiratory motion prediction in mr-
guided radiotherapy. Physics in Medicine & Biology,
67(9):095006.
Manjah, D., Cacciarelli, D., Standaert, B., Benkedadra,
M., de Hertaing, G. R., Macq, B., Galland, S., and
De Vleeschouwer, C. (2023). Stream-based active dis-
tillation for scalable model deployment. In Proceed-
ings of the IEEE/CVF Conference on Computer Vision
and Pattern Recognition, pages 4998–5006.
Naphade, M., Wang, S., Anastasiu, D. C., Tang, Z., Chang,
M.-C., Yang, X., Yao, Y., Zheng, L., Chakraborty, P.,
Lopez, C. E., Sharma, A., Feng, Q., Ablavsky, V., and
Sclaroff, S. (2021). The 5th ai city challenge. In
The IEEE Conference on Computer Vision and Pat-
tern Recognition (CVPR) Workshops.
Norbert, W. (1949). Extrapolation, interpolation, and
smoothing of stationary time series: with engineer-
ing applications, volume 113. MIT press Cambridge,
MA.
Quintanar, A., Fern
´
andez-Llorca, D., Parra, I., Izquierdo,
R., and Sotelo, M. (2021). Predicting vehicles trajec-
tories in urban scenarios with transformer networks
and augmented information. In 2021 IEEE Intelligent
Vehicles Symposium (IV), pages 1051–1056. IEEE.
Redmon, J., Divvala, S., Girshick, R., and Farhadi, A.
(2016). You only look once: Unified, real-time object
detection. In Proceedings of the IEEE conference on
computer vision and pattern recognition, pages 779–
788.
Robicquet, A., Sadeghian, A., Alahi, A., and Savarese,
S. (2016). Learning social etiquette: Human trajec-
tory understanding in crowded scenes. In Computer
Vision–ECCV 2016: 14th European Conference, Am-
sterdam, The Netherlands, October 11-14, 2016, Pro-
ceedings, Part VIII 14, pages 549–565. Springer.
Romaguera, L. V., Alley, S., Carrier, J.-F., and Kadoury, S.
(2023). Conditional-based transformer network with
learnable queries for 4d deformation forecasting and
tracking. IEEE Transactions on Medical Imaging.
Sadeghian, A., Kosaraju, V., Gupta, A., Savarese, S., and
Alahi, A. (2018). Trajnet: Towards a benchmark for
human trajectory prediction. arXiv preprint.
Salzmann, T., Ivanovic, B., Chakravarty, P., and Pavone,
M. (2020). Trajectron++: Dynamically-feasible tra-
jectory forecasting with heterogeneous data. In Com-
puter Vision–ECCV 2020: 16th European Confer-
ence, Glasgow, UK, August 23–28, 2020, Proceed-
ings, Part XVIII 16, pages 683–700. Springer.
Shi, S., Jiang, L., Dai, D., and Schiele, B. (2022). Mo-
tion transformer with global intention localization and
local movement refinement. Advances in Neural In-
formation Processing Systems, 35:6531–6543.
Vaswani, A., Shazeer, N., Parmar, N., Uszkoreit, J., Jones,
L., Gomez, A. N., Kaiser, Ł., and Polosukhin, I.
(2017). Attention is all you need. Advances in neural
information processing systems, 30.
Yao, H.-Y., Wan, W.-G., and Li, X. (2022). End-
to-end pedestrian trajectory forecasting with trans-
former network. ISPRS International Journal of Geo-
Information, 11(1):44.
Zhang, P., Bai, L., Wang, Y., Fang, J., Xue, J., Zheng, N.,
and Ouyang, W. (2023). Towards trajectory forecast-
ing from detection. IEEE Transactions on Pattern
Analysis and Machine Intelligence.
ICPRAM 2024 - 13th International Conference on Pattern Recognition Applications and Methods
760