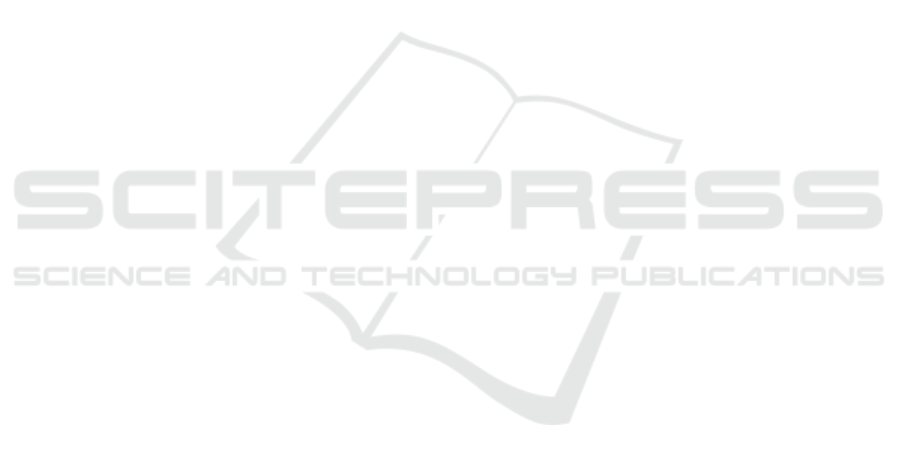
Bull, H., Gouiff
`
es, M., and Braffort, A. (2020b). Automatic
segmentation of sign language into subtitle-units. In
ECCVW, Sign Language Recognition, Translation and
Production (SLRTP).
Camgoz, N. C., Hadfield, S., Koller, O., Ney, H., and Bow-
den, R. (2018a). Neural sign language translation. In
CVPR.
Camgoz, N. C., Hadfield, S., Koller, O., Ney, H., and Bow-
den, R. (2018b). Neural sign language translation. In
Proceedings of the IEEE Conference on Computer Vi-
sion and Pattern Recognition, pages 7784–7793.
Camgoz, N. C., Koller, O., Hadfield, S., and Bowden, R.
(2020). Sign language transformers: Joint end-to-end
sign language recognition and translation. In IEEE
Conference on Computer Vision and Pattern Recogni-
tion (CVPR).
Camgoz, N. C., Saunders, B., Rochette, G., Giovanelli,
M., Inches, G., Nachtrab-Ribback, R., and Bowden,
R. (2021). Content4all open research sign language
translation datasets. arXiv preprint arXiv:2105.02351.
Cao, Z., Hidalgo, G., Simon, T., Wei, S.-E., and Sheikh,
Y. (2019). OpenPose: Realtime multi-person 2D pose
estimation using part affinity fields. IEEE Transac-
tions on Pattern Analysis and Machine Intelligence,
43(1):172–186.
Dayter, D. (2019). Collocations in non-interpreted and si-
multaneously interpreted english: a corpus study. In
New empirical perspectives on translation and inter-
preting, pages 67–91. Routledge.
Duarte, A., Albanie, S., Gir
´
o-i Nieto, X., and Varol, G.
(2022). Sign language video retrieval with free-form
textual queries. In Proceedings of the IEEE/CVF Con-
ference on Computer Vision and Pattern Recognition,
pages 14094–14104.
Erard, M. (2017). Why sign-language gloves
don’t help deaf people. The Atlantic, https:
//www.theatlantic.com/technology/archive/2017/11/
why-sign-language-gloves-dont-help-deaf-people/
545441/.
Fink, J., Fr
´
enay, B., Meurant, L., and Cleve, A. (2021).
Lsfb-cont and lsfb-isol: Two new datasets for vision-
based sign language recognition.
Forster, J., Schmidt, C., Hoyoux, T., Koller, O., Zelle, U.,
Piater, J. H., and Ney, H. (2012). Rwth-phoenix-
weather: A large vocabulary sign language recog-
nition and translation corpus. In Proceedings of
the Eighth International Conference on Language
Resources and Evaluation (LREC’12), pages 3746–
3753, Istanbul, Turkey. European Language Resource
Association (ELRA).
Forster, J., Schmidt, C., Koller, O., Bellgardt, M., and Ney,
H. (2014). Extensions of the sign language recog-
nition and translation corpus rwth-phoenix-weather.
In Proceedings of the Ninth International Conference
on Language Resources and Evaluation (LREC’14),
pages 1911–1916.
Huang, J., Zhou, W., Zhang, Q., Li, H., and Li, W. (2018).
Video-based sign language recognition without tem-
poral segmentation. In Thirty-Second AAAI Confer-
ence on Artificial Intelligence.
Jiang, T., Camgoz, N. C., and Bowden, R. (2021a). Look-
ing for the signs: Identifying isolated sign instances
in continuous video footage. IEEE International Con-
ferene on Automatic Face and Gesture Recognition.
Jiang, T., Camgoz, N. C., and Bowden, R. (2021b). Skele-
tor: Skeletal transformers for robust body-pose esti-
mation. In IEEE/CVF Conference on Computer Vision
and Pattern Recognition (CVPR).
Kudo, T. and Richardson, J. (2018). Sentencepiece: A sim-
ple and language independent subword tokenizer and
detokenizer for neural text processing. arXiv preprint
arXiv:1808.06226.
Leeson, L. (2005). Making the effort in simultaneous inter-
preting. In Topics in Signed Language Interpreting:
Theory and Practice, volume 63, chapter 3, pages 51–
68. John Benjamins Publishing.
Liu, Z., Ning, J., Cao, Y., Wei, Y., Zhang, Z., Lin, S., and
Hu, H. (2022). Video swin transformer. In Proceed-
ings of the IEEE/CVF Conference on Computer Vision
and Pattern Recognition, pages 3202–3211.
Lugaresi, C., Tang, J., Nash, H., McClanahan, C., Uboweja,
E., Hays, M., Zhang, F., Chang, C., Yong, M. G., Lee,
J., Chang, W., Hua, W., Georg, M., and Grundmann,
M. (2019). Mediapipe: A framework for building per-
ception pipelines. CoRR, abs/1906.08172.
Momeni, L., Bull, H., Prajwal, K., Albanie, S., Varol, G.,
and Zisserman, A. (2022). Automatic dense anno-
tation of large-vocabulary sign language videos. In
Computer Vision–ECCV 2022: 17th European Con-
ference, Tel Aviv, Israel, October 23–27, 2022, Pro-
ceedings, Part XXXV, pages 671–690. Springer.
Morford, J. P. and Carlson, M. L. (2011). Sign perception
and recognition in non-native signers of asl. Language
learning and development, 7(2):149–168.
M
¨
uller, M., Ebling, S., Avramidis, E., Battisti, A., Berger,
M., Zurich, H., Bowden, R., Braffort, A., Camg
¨
oz,
N. C., Espa
˜
na-Bonet, C., et al. Findings of the first
wmt shared task on sign language translation.
Post, M. (2018). A call for clarity in reporting BLEU scores.
In Proceedings of the Third Conference on Machine
Translation: Research Papers, pages 186–191, Bel-
gium, Brussels. Association for Computational Lin-
guistics.
Prajwal, K., Bull, H., Momeni, L., Albanie, S., Varol, G.,
and Zisserman, A. (2022). Weakly-supervised fin-
gerspelling recognition in british sign language. In
British Machine Vision Conference (BMVC) 2022.
Renz, K., Stache, N. C., Fox, N., Varol, G., and Al-
banie, S. (2021). Sign segmentation with changepoint-
modulated pseudo-labelling. In Proceedings of the
IEEE/CVF Conference on Computer Vision and Pat-
tern Recognition, pages 3403–3412.
Saunders, B., Camgoz, N. C., and Bowden, R. (2020). Ev-
erybody sign now: Translating spoken language to
photo realistic sign language video. arXiv preprint
arXiv:2011.09846.
Schembri, A., Fenlon, J., Rentelis, R., and Cormier,
K. (2017). British Sign Language corpus project:
A corpus of digital video data and annotations of
Mediapi-RGB: Enabling Technological Breakthroughs in French Sign Language (LSF) Research Through an Extensive Video-Text Corpus
147