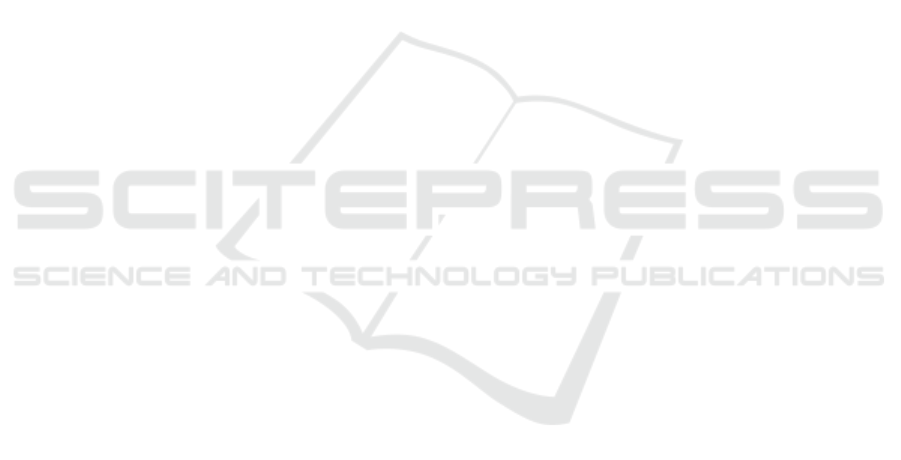
powder and water are put in a pot and rice is put in
a bowl. Therefore, to solve this error, we need a sys-
tem that optimizes the tools while also considering the
content of successive sequences.
In future work, we propose a method for optimiz-
ing the motion sequence for performing the task, and
correcting the tools to be used to take into account the
motion sequence before and after.
ACKNOWLEDGEMENTS
This paper is based on results obtained from a
project, JPNP20006, commissioned by the New En-
ergy and Industrial Technology Development Organi-
zation (NEDO).
REFERENCES
Ardon, P., Pairet, E., Petrick, R., Ramamoorthy, S., and Lo-
han, K. (2020). Self-assessment of grasp affordance
transfer. In Proceedings of IEEE/RSJ International
Conference on Intelligent Robots and Systems (IROS),
pages 9385–9392.
Do, T., Nguyen, A., and Reid, I. (2018). Affordancenet:
An end-to-end deep learning approach for object af-
fordance detection. In Proceedings of IEEE In-
ternational Conference on Robotics and Automation
(ICRA), pages 5882–5889.
Domae, Y., Okuda, H., Taguchi, Y., Sumi, K., and Hi-
rai, T. (2014). Fast graspability evaluation on sin-
gle depth maps for bin picking with general grippers.
In Proceedings of IEEE International Conference on
Robotics and Automation (ICRA), pages 1997–2004.
Inagawa, M., Takei, T., and Imanishi, E. (2020). Japanese
recipe interpretation for motion process generation of
cooking robot. In Proceedings of IEEE/SICE Interna-
tional Symposium on System Integration (SII).
Liang, J. and Boularias, A. (2023). Learning category-level
manipulation tasks from point clouds with dynamic
graph cnns. In Proceedings of IEEE International
Conference on Robotics and Automation (ICRA)),
pages 1807–1813.
Liu, W., Anguelov, D., Erhan, D., Szegedy, C., Reed, S., Fu,
C., and Berg, A. (2016). Ssd: Single shot multibox
detector. In Proceedings of European conference on
computer vision (ECCV), pages 21–37.
Minh, C., Gilani, S., Islam, S., and Suter, D. (2020). Learn-
ing affordance segmentation: An investigative study.
In Proceedings of International Conference on Dig-
ital Image Computing: Techniques and Applications
(DICTA), pages 2870–2877.
Myers, A., Teo, C., Ferm
¨
uller, C., and Aloimonos, Y.
(2015). Affordance detection of tool parts from
geometric features. In Proceedings of IEEE In-
ternational Conference on Robotics and Automation
(ICRA), pages 1374–1381.
Qi, C. R., Yi, L., Su, H., and Guibas, L. J. (2017). Point-
net++: Deep hierarchical feature learning on point sets
in a metric space. In Proceedings of Advances in Neu-
ral Information Processing Systems (NIPS).
Qin, Z., Fang, K., Zhu, Y., Fei-Fei, L., and Savarese,
S. (2020). Keto:learning keypoint representations
for tool manipulation. In Proceedings of IEEE In-
ternational Conference on Robotics and Automation
(ICRA), pages 7278–7285.
Redmon, J., Divvala, S., Girshick, R., and Farhadi, A.
(2016). You only look once:unified, real-time ob-
ject detection. In Proceedings of the IEEE conference
on computer vision and pattern recognition (CVPR),
pages 779–788.
Song, S., Zeng, A., Lee, J., and Funkhouser, T. (2020).
Grasping in the wild: Learning 6dof closed-loop
grasping from low-cost demonstrations. In IEEE
Robotics and Automation Letters, volume 5, pages
4978–4985.
Takata, K., Kiyokawa, T., Ramirez-Alpizar, I. G., Ya-
manobe, N., Wan, W., and Harada, K. (2022). Effi-
cient task/motion planning for a dual-arm robot from
language instructions and cooking images. In Pro-
ceedings of IEEE/RSJ International Conference on In-
telligent Robots and Systems (IROS), pages 12058–
12065.
Tsusaka, Y., Okazaki, Y., komatsu, M., and Yokokohji, Y.
(2012). Development of in-situ robot motion modifi-
cation method by hand-guiding instruction. In Trans-
actions of the JSME (in Japanese), pages 461–467.
Xu, R., Chu, F.-J., Tang, C., Liu, W., and Vela, P. (2021).
An affordance keypoint detection network for robot
manipulation. In IEEE Robotics and Automation Let-
ters, volume 6, pages 2870–2877.
Yamada, T., Murata, S., Arie, H., and Ogata, T. (2020). Rep-
resentation learning of logic words by an rnn: from
word sequences to robot actions. In Frontiers in neu-
rorobotics, volume 11.
Zhang, X., Koyama, K., Domae, Y., Wan, W., and Harada,
K. (2021). A topological solution of entanglement
for complex-shaped parts in robotic bin-picking. In
Proceedings of IEEE International Conference on
Automation Science and Engineering (CASE), pages
461–467.
Automatic Error Correction of GPT-Based Robot Motion Generation by Partial Affordance of Tool
323