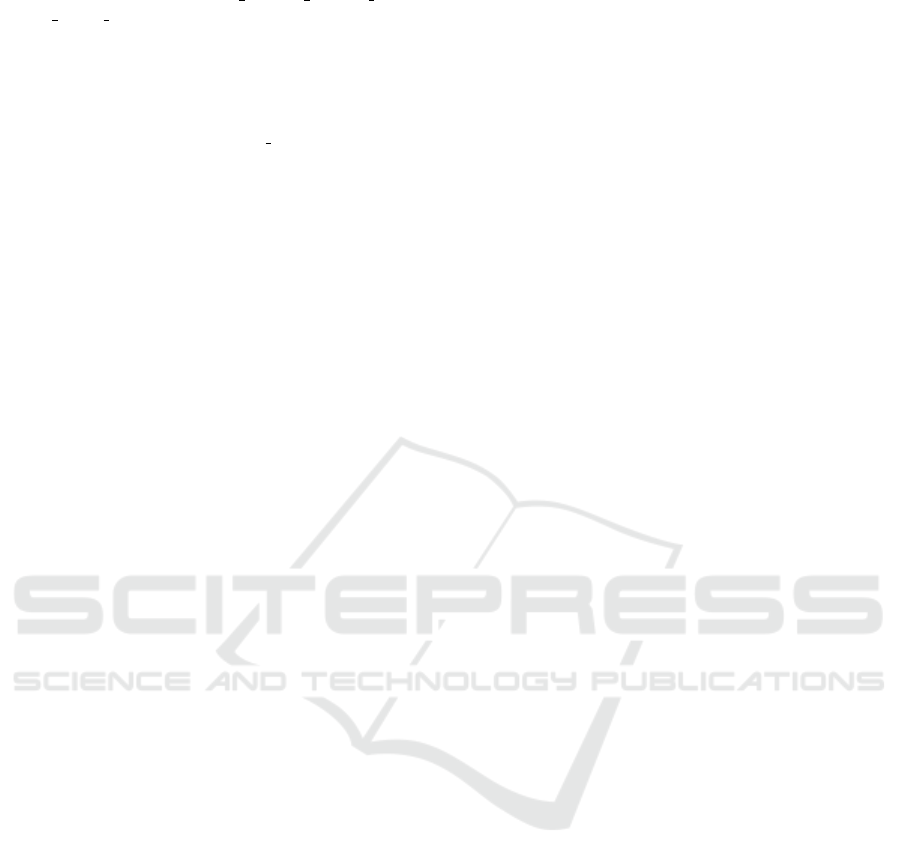
veillance/epi-profiles/Epi\ Profile\ Tables\ Florida
\ 2018\ Locked.xlsx.
Florida Department of Health (2020). Florida’s Uni-
fied Ending the HIV Epidemic Plan [Division
of Disease Control and Health Protection Bu-
reau of Communicable Diseases HIV/AIDS Sec-
tion]. https://www.floridahealth.gov/diseases-and-
conditions/aids/administration/ documents/fl-unified-
ehe-plan.pdf.
Florida Department of Health (2023). HIV, AIDS and
PWH Cases by Year and by County in Florida.
https://www.flhealthcharts.gov/ChartsDashboards/rd
Page.aspx?rdReport=HIVAIDS.DataViewer&cid=986
6.
Hosmer Jr, D. W., Lemeshow, S., and Sturdivant, R. X.
(2013). Applied logistic regression, volume 398. John
Wiley & Sons.
Kapoor, A., Ben, X., Liu, L., Perozzi, B., Barnes, M., Blais,
M., and O’Banion, S. (2020). Examining COVID-
19 forecasting using spatio-temporal graph neural net-
works. arXiv preprint arXiv:2007.03113.
Kipf, T. N. and Welling, M. (2017). Semi-Supervised Clas-
sification with Graph Convolutional Networks. In In-
ternational Conference on Learning Representations
(ICLR), Palais des Congr
`
es Neptune, Toulon, France.
April 24 - 26, 2017.
Lieb, S., White, S., Grigg, B. L., Thompson, D. R., Lib-
erti, T. M., and Fallon, S. J. (2010). Estimated
HIV incidence, prevalence, and mortality rates among
racial/ethnic populations of men who have sex with
men, Florida. JAIDS Journal of Acquired Immune De-
ficiency Syndromes, 54(4):398–405.
Liu, Y., Parisi, C., Fisk-Hoffman, R., Salemi, M., Viteri,
D., Prosperi, M., and Marini, S. (2023). Behavioral
and demographic profiles of HIV contact networks in
Florida. In International Conference on Intelligent Bi-
ology and Medicine (ICIBM 2023), Tampa, Florida,
United States. July 16-19, 2023. Abstract number
6527.
Ramchandani, A., Fan, C., and Mostafavi, A. (2020). Deep-
covidnet: An interpretable deep learning model for
predictive surveillance of COVID-19 using heteroge-
neous features and their interactions. IEEE Access,
8:159915–159930.
Skarding, J., Gabrys, B., and Musial, K. (2021). Founda-
tions and modeling of dynamic networks using dy-
namic graph neural networks: A survey. IEEE Access,
9:79143–79168.
Stekhoven, D. J. and B
¨
uhlmann, P. (2012).
MissForest—non-parametric missing value im-
putation for mixed-type data. Bioinformatics,
28(1):112–118.
Sun, C., Fang, R., Salemi, M., Prosperi, M., and Rife Ma-
galis, B. (2023). DeepDynaForecast: Phylogenetic-
informed graph deep learning for epidemic transmis-
sion dynamic prediction. bioRxiv, pages 2023–07.
Sun, C., Li, Y., Marini, S., Riva, A., Wu, D. O., Salemi,
M., and Magalis, B. R. (2022). Phylogenetic-informed
graph deep learning to classify dynamic transmission
clusters in infectious disease epidemics. bioRxiv,
pages 2022–04.
Tan, R. K. J., Tang, W., and Tucker, J. D. (2023). Pub-
lic health services and intersectional stigma: a social
sciences perspective with implications for HIV ser-
vice design and delivery. Current Opinion in HIV and
AIDS, 18(1):18–26.
Tomy, A., Razzanelli, M., Di Lauro, F., Rus, D., and
Della Santina, C. (2022). Estimating the state of epi-
demics spreading with graph neural networks. Non-
linear Dynamics, 109(1):249–263.
Traag, V. A., Waltman, L., and Van Eck, N. J. (2019). From
Louvain to Leiden: guaranteeing well-connected
communities. Scientific Reports, 9(1):5233.
Trepka, M. J., Niyonsenga, T., Maddox, L., Lieb, S., Lutfi,
K., and Pavlova-McCalla, E. (2013). Community
poverty and trends in racial/ethnic survival dispari-
ties among people diagnosed with AIDS in Florida,
1993–2004. American Journal of Public Health,
103(4):717–726.
Von Winterfeldt, D. and Edwards, W. (1986). Decision
analysis and behavioral research.
Wang, L., Adiga, A., Chen, J., Sadilek, A., Venkatramanan,
S., and Marathe, M. (2022). Causalgnn: Causal-based
graph neural networks for spatio-temporal epidemic
forecasting. In Proceedings of the AAAI Conference
on Artificial Intelligence, volume 36, pages 12191–
12199. February 22 – March 1, 2022.
Wright, I. A., Reid, R., Shahid, N., Ponce, A., Nelson,
C. M., Sanders, J., Gardner, N., Liu, J., Simmons, E.,
Phillips, A., Pan, Y., Alcaide, M. L., Rodriguez, A.,
Ironson, G., Feaster, D. J., Safren, S. A., and Dale,
S. K. (2022). Neighborhood Characteristics, Intersec-
tional Discrimination, Mental Health, and HIV Out-
comes Among Black Women Living With HIV, South-
eastern United States, 2019–2020. American Journal
of Public Health, 112(S4):S433–S443.
Wu, Z., Pan, S., Chen, F., Long, G., Zhang, C., and Philip,
S. Y. (2020). A comprehensive survey on graph neural
networks. IEEE Transactions on Neural Networks and
Learning Systems, 32(1):4–24.
Xu, K., Hu, W., Leskovec, J., and Jegelka, S. (2019).
How powerful are graph neural networks? In-
ternational Conference on Learning Representations
(ICLR). May 6 - 9, 2017.
Zhang, L., Wang, S., and Liu, B. (2018). Deep learning
for sentiment analysis: A survey. Wiley Interdisci-
plinary Reviews: Data Mining and Knowledge Dis-
covery, 8(4):e1253.
Zhou, J., Cui, G., Hu, S., Zhang, Z., Yang, C., Liu, Z.,
Wang, L., Li, C., and Sun, M. (2020). Graph neu-
ral networks: A review of methods and applications.
AI Open, 1:57–81.
Learning on Forecasting HIV Epidemic Based on Individuals’ Contact Networks
111