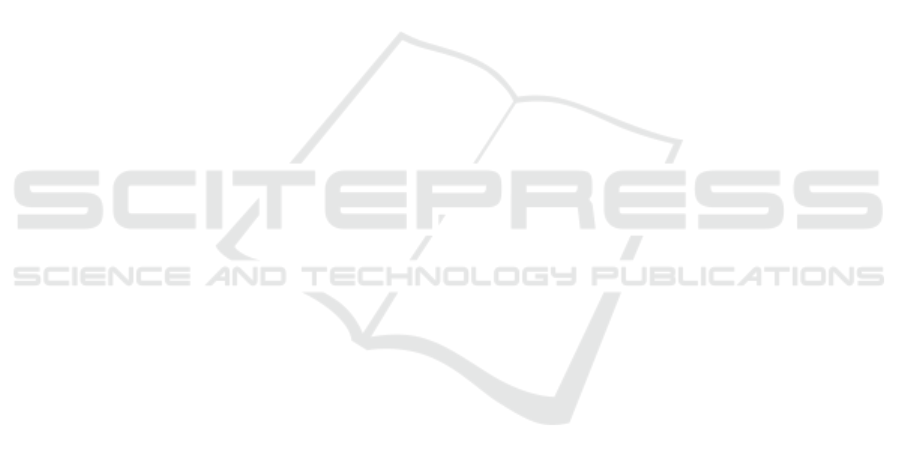
REFERENCES
Acici K, O. g. H. and et al. (2017). A random forest method
to detect parkinson’s disease via gait analysis. In Engi-
neering Applications of Neural Networks.
Archila J., M. A. . M. F. (2022). A multimodal parkinson
quantification by fusing eye and gait motion patterns,
using covariance descriptors , from non-invasive com-
puter vision. In Computer Methods and Programs in
Biomedicine.
Asuroglu T, O. H. and et al. (2018). Parkinson’s disease
monitoring from gait analysis via foot-worn sensors.
In Biocybern Biomed Eng.
Cherubini A, M. M. and et al. (2014). Magnetic resonance
support vector machine discriminates between parkin-
son’s disease and progressive supranuclear palsy. In
International Parkinson and Movement Disorder Soci-
ety.
Du G., Lewis M., K. S. and et al. (2017). Combined diffu-
sion tensor imaging and apparent transverse relaxation
rate differentiate parkinson disease and atypical parkin-
sonism. In In American Journal of Neuroradiology.
Emamzadeh, F. N. and Surguchov, A. (2018). Parkinson’s
disease: Biomarkers, treatment, and risk factors. In
Frontiers in Neuroscience.
Ghaderyan, P. and Fathi, G. (2021). Inter-limb time-varying
singular value: A new gait feature for parkinson’s dis-
ease detection and stage classification. Measurement,
177:109249.
Glaab E, Trezzi JP, D. N., C., E., and et al. (2019). Inte-
grative analysis of blood metabolomics and pet brain
neuroimaging data for parkinson’s disease. In Neuro-
biology of Disease.
Goldberger, A., S. H. E. and et al. (2000). Physiobank,
physiotoolkit, and physionet: Components of a new
research resource for complex physiologic signals. In
In Proc. CVPR.
H. Hirschauer, T. J., Adeli, B., and A., J. (2015). Computer-
aided diagnosis of parkinson’s disease using enhanced
probabilistic neural network. In In Journal of Medical
Systems.
Leger C, Herbert M, D. J. (2020). Non-motor clinical and
biomarker predictors enable high cross-validated accu-
racy detection of early pd but lesser cross-validated ac-
curacy detection of scans without evidence of dopamin-
ergic deficit. In Frontiers in Neurology.
Liu, X., Li, W., Liu, Z., Du, F., and Zou, Q. (2021). A
dual-branch model for diagnosis of parkinson’s disease
based on the independent and joint features of the left
and right gait. Applied Intelligence, 51(10):7221–7232.
Long D., W. J. and et al. (2012). Automatic classification of
early parkinson’s disease with multi-modal mr imaging.
In In PLOS ONE.
Makarious, M. B., Leonard, H. L., Vitale, D., and et al.
(2022). Multi-modality machine learning predicting
parkinson’s disease. npj Parkinson’s Disease, 8(1):35.
MS Hema, M Nageswara Guptha, V. P. and et al. (2023).
Prediction analysis for parkinson disease using mul-
tiple feature selection and classification methods. In
Multimedia Tools and Applications.
Oliveira, F. P. M., Faria, and et al. (2018). Extraction, se-
lection and comparison of features for an effective
automated computer-aided diagnosis of parkinson’s
disease based on [123i]fp-cit spect images. European
Journal of Nuclear Medicine and Molecular Imaging,
45(6):1052–1062.
Pahuja G., P. B. (2022). Deep learning architectures for
parkinson’s disease detection by using multi-modal
features. In Computers in Biology and Medicine.
Prashanth, R., Dutta Roy, S., Mandal, P. K., and Ghosh,
S. (2016). High-accuracy detection of early parkin-
son’s disease through multimodal features and machine
learning. International Journal of Medical Informatics,
90:13–21.
Priya SJ, R. A. and N., U. (2020). Improving the prediction
accuracy of parkinson’s disease based on pattern tech-
niques. In 5th International Conference on Devices,
Circuits and Systems.
Tong J, Zhang J, D. E. and S., D. (2021). Severity classi-
fication of parkinson’s disease based on permutation-
variable importance and persistent entropy. In Multi-
media Tools and Applications.
Veeraragavan S, Gopalai AA, G. D. and SA., A. (2020).
Parkinson’s disease diagnosis and severity assessment
using ground reaction forces and neural networks. In
Front Physiol.
Xia Yand Yao Z, Y. Q. and N., C. (2020). A dual-modal
attention-enhanced deep learning network for quantifi-
cation of parkinson’s disease characteristics. In IEEE
Trans Neural Syst Rehabil Eng.
Zeng W, Liu F, Z. Y. and et al. (2016). Parkinson’s dis-
ease classification using gait analysis via deterministic
learning. In Neurosci Lett.
Zhao, A., Qi, L., Li, J., Dong, J., and Yu, H. (2018). A hybrid
spatio-temporal model for detection and severity rating
of parkinson’s disease from gait data. Neurocomputing,
315:1–8.
Mutually Exclusive Multi-Modal Approach for Parkinson’s Disease Classification
243