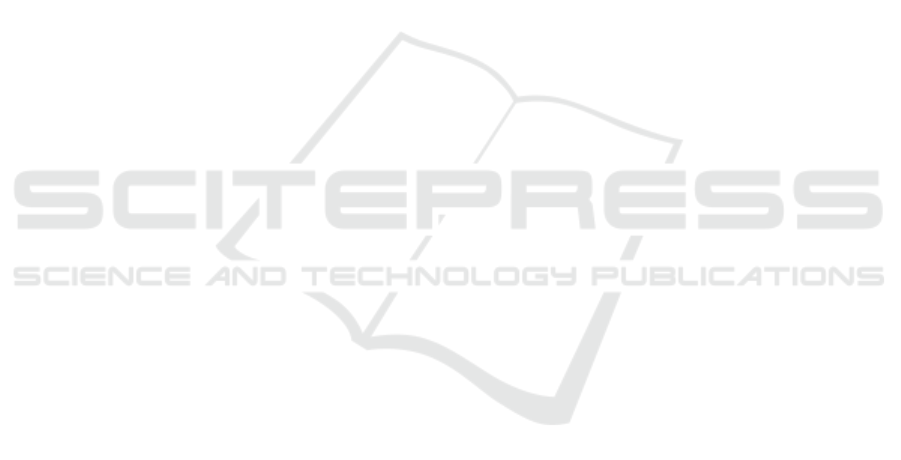
A. This is thought to be one of the reasons for A's
superiority. As shown in the additional questionnaire,
personal preference is also considered to be an issue.
In addition, in questions 1-3, the number of
respondents who answered that B was easier to
understand/slightly easier to understand than 1-1 and
1-2 was considerably larger, suggesting that the
content of the displayed reviews may also be a factor.
We would like to continue to examine the comparison
with the existing method by changing the conditions,
etc.
5.2 Comparison of Score and Text
Displays
This part, which compared the score display only with
the display in the review text, showed that the score
display was easier to understand. However, in all
questions, some respondents answered that the review
text by several people B was easier to convey.
Therefore, believe that the information may be more
easily conveyed to users if the obtained scores and the
predicted review sentences are listed together.
5.3 Weighting of Features
This part, which compares weighted and unweighted
reviews, shows that weighting makes it easier to
convey information about specific items. This
weighting is thought to make it easier to convey
information that is not often mentioned in ordinary
reviews, but that the user cares about.
6 CONCLUSION
Based on the experimental results, the efficacy of
generating and presenting a single review that appears
as if written by the user being recommended to was
confirmed. However, when compared to a vast
number of reviews written by other users, its utility
was found to be relatively lower. We believe that it
can contribute to users' easy understanding of
information when deciding whether to purchase a
product by combining the scoring given when a
product is purchased with review text tailored to the
user's preferences.
As future prospects for this research, we would
like to improve the usefulness of this method in
comparison with existing methods, the accuracy of
product recommendations, and the accuracy of
predicted review sentences, which were the issues in
this experiment.
ACKNOWLEDGEMENTS
This research was supported by JSPS Grants-in-Aid
for Scientific Research JP21H03496, JP22K12157,
JP23H03688 and Sumitomo Electric Industries
Group Social Contribution Fund.
In this paper, we used "Rakuten Dataset"
(https://rit.rakuten.com/data_release/) provided by
Rakuten Group, Inc. via IDR Dataset Service of
National Institute of Informatics.
REFERENCES
Darius Afchar, Romain Hennequin. (2020). Making neural
networks interpretable with attribution: application to
implicit signals prediction. In 14th ACM Conference on
Recommender Systems, pp.220-229.
Riya Ambolkar, Arpita Bhagat, Bhakti Buga, and Swapnil
Gharat. (2022). Hotel Recommendation System using
advanced efficiency and accuracy with modified BERT
technique. In 2022 Second International Conference on
Artificial Intelligence and Smart Energy (ICAIS)
Dimple Chehal, Parul Gupta, and Payal Gulati. (2022). An
Approach to Utilize E-commerce Product Reviews to
Remove Irrelevant Recommendations. In 2022 IEEE
Delhi Section Conference (DELCON)
Taluya Hara, Jun Baba, and Takuya Iwamoto. (2021). Item-
Driven Recommrndation: A Recommendation System
for Other Items Based on the Users. In The 35th Annual
Conference of the Japanese Socie 2021
Taichi Imafuku, Tatsuya Kawakami, Tianxiang Yang, and
Masayuki Goto. (2021). A Study on Item
Recommendation Model by Evaluating the Effect of
Individual Intervention. In The 35th Annual
Conference of the Japanese Society for Artificial
Intelligence 2021.
Rio Iwabuchi, Yoko Nakajima, Hirotoshi Honma, Haruka
Aoshima, Akio Kobayashi, Tomoyoshi Akiba, and
Shigeru Masuyama. (2017). Proposal of recommender
system based on user evaluation and cosmetic
ingredients. In 2019 4th International Conference on
Information Technology (InCIT)
P.Kirubanantham, A.Saranya, and D.Senthil Kumar.
(2022). Convolutional Recommended Neural Network
system based on user reviews for movies.In 2021 4th
International Conference on Computing and
Communications Technologies (ICCCT) .
Yuki Matsunami, Mayumi Ueda, Shinsuke Nakajima,
Takeru Hashikami, Sunao Iwasaki, John O'Donovan,
and Byungkyu kang. (2016). An automatic scoring
method for review by evaluation item using a cosmetic
item evaluation expression dictionary. In 8th Forum on
Data Engineering and Information Management
(DEIM Forum 2016) B1-1.
Hiromu Matsushima, Shun Morisawa, Takumi Ishikawa,
and hayato Yamana. (2021). A Survey of Explainable
Proposal of a Cosmetic Product Recommendation Method with Review Text that is Predicted to Be Write by Users
615