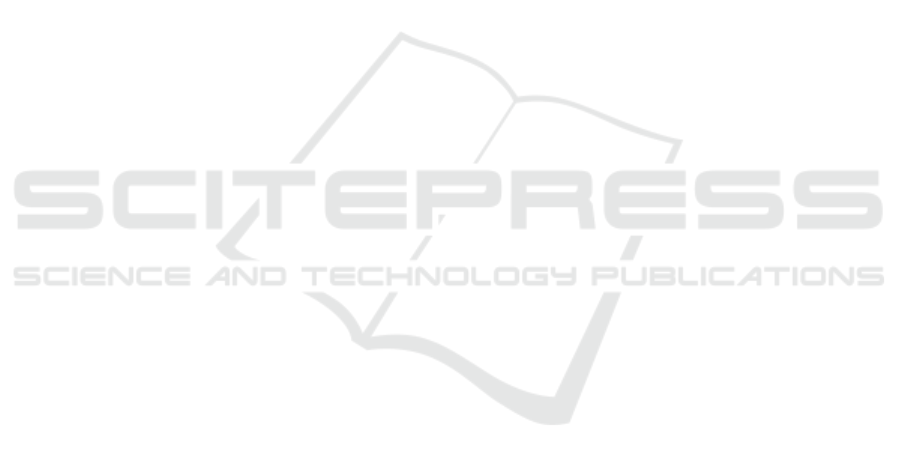
tection on the Cityscapes dataset by applying the re-
optimization model to the baseline models in the do-
main of autonomous driving. These experiments were
conducted employing three distinct methodologies for
the generation of knowledge graphs, including our
novel hybrid knowledge graph generation approach.
The evaluation results suggest that certain ob-
ject detection models may benefit from treating the
knowledge-aware component. It was observed that
more advanced architectures, such as transformers,
possess sufficient sophistication that external knowl-
edge as currently implemented may have a detrimen-
tal impact on their performance. Hence, it is impera-
tive for the community to explore new approaches to
incorporate practical knowledge into the object detec-
tion frameworks.
ACKNOWLEDGEMENTS
This work was supported by the German Ministry
for Economic Affairs and Climate Action (BMWK)
project KI-Wissen under Grant 19A20020G.
REFERENCES
Akiba, T., Sano, S., Yanase, T., Ohta, T., and Koyama, M.
(2019). Optuna: A next-generation hyperparameter
optimization framework. In Proceedings of the 25th
ACM SIGKDD International Conference on Knowl-
edge Discovery and Data Mining.
Altenberger, F. and Lenz, C. (2018). A non-technical survey
on deep convolutional neural network architectures.
Aodha, O. M., Cole, E., and Perona, P. (2019). Presence-
only geographical priors for fine-grained image clas-
sification. 2019 IEEE/CVF International Conference
on Computer Vision (ICCV), pages 9595–9605.
Beemelmanns, T. (2022). cityscapes to coco conversion.
Carion, N., Massa, F., Synnaeve, G., Usunier, N., Kirillov,
A., and Zagoruyko, S. (2020). End-to-end object de-
tection with transformers. In Vedaldi, A., Bischof, H.,
Brox, T., and Frahm, J.-M., editors, Computer Vision
– ECCV 2020, pages 213–229, Cham. Springer Inter-
national Publishing.
Castellano, G., Digeno, V., Sansaro, G., and Vessio, G.
(2022). Leveraging knowledge graphs and deep learn-
ing for automatic art analysis. Knowledge-Based Sys-
tems, 248:108859.
Chen, S., Li, Z., and Tang, Z. (2020). Relation r-cnn: A
graph based relation-aware network for object detec-
tion. IEEE Signal Processing Letters, 27:1680–1684.
Cordts, M., Omran, M., Ramos, S., Rehfeld, T., Enzweiler,
M., Benenson, R., Franke, U., Roth, S., and Schiele,
B. (2016). The cityscapes dataset for semantic urban
scene understanding. CoRR, abs/1604.01685.
Fang, Y., Kuan, K., Lin, J., Tan, C., and Chandrasekhar,
V. (2017). Object detection meets knowledge graphs.
International Joint Conferences on Artificial Intelli-
gence.
LeCun, Y., Bengio, Y., and Hinton, G. (2015). Deep learn-
ing. nature, 521(7553):436–444.
Lemmens, J., Jancura, P., Dubbelman, G., and Elrofai, H.
(2023). [re] object detection meets knowledge graphs.
ReScience C, 9(1).
Liu, Y., Zhang, Z., Niu, L., Chen, J., and Zhang, L.
(2021). Mixed supervised object detection by trans-
ferring mask prior and semantic similarity. Advances
in Neural Information Processing Systems, 34.
Menglong, C., Detao, J., Ting, Z., Dehai, Z., Cheng, X.,
Zhibo, C., and Xiaoqiang, X. (2019). Image clas-
sification based on image knowledge graph and se-
mantics. In 2019 IEEE 23rd International Conference
on Computer Supported Cooperative Work in Design
(CSCWD), pages 81–86. IEEE.
Redmon, J. and Farhadi, A. (2018). Yolov3: An incremental
improvement. arXiv preprint arXiv:1804.02767.
Ren, S., He, K., Girshick, R., and Sun, J. (2016). Faster
r-cnn: towards real-time object detection with region
proposal networks. IEEE transactions on pattern
analysis and machine intelligence, 39(6):1137–1149.
Speer, R., Havasi, C., CHAIDEZ, J., VENEZUELA, J., and
KUO, Y. (2012). Conceptnet 5. Tiny Transactions of
Computer Science.
Tong, H., Faloutsos, C., and Pan, J.-Y. (2006). Fast ran-
dom walk with restart and its applications. In Sixth
international conference on data mining (ICDM’06),
pages 613–622. IEEE.
von Rueden, L., Mayer, S., Beckh, K., Georgiev, B., Gies-
selbach, S., Heese, R., Kirsch, B., Pfrommer, J., Pick,
A., Ramamurthy, R., Walczak, M., Garcke, J., Bauck-
hage, C., and Schuecker, J. (2023). Informed machine
learning – a taxonomy and survey of integrating prior
knowledge into learning systems. IEEE Transactions
on Knowledge and Data Engineering, 35(1):614–633.
Zhou, J., Cui, G., Hu, S., Zhang, Z., Yang, C., Liu, Z.,
Wang, L., Li, C., and Sun, M. (2020). Graph neu-
ral networks: A review of methods and applications.
AI Open, 1:57–81.
Zhu, C., Chen, F., Ahmed, U., Shen, Z., and Savvides, M.
(2021). Semantic relation reasoning for shot-stable
few-shot object detection. 2021 IEEE/CVF Confer-
ence on Computer Vision and Pattern Recognition
(CVPR), pages 8778–8787.
Zhu, X., Li, Z., Wang, X., Jiang, X., Sun, P., Wang, X.,
Xiao, Y., and Yuan, N. J. (2022). Multi-modal knowl-
edge graph construction and application: A survey.
IEEE Transactions on Knowledge and Data Engineer-
ing.
Zou, Z., Chen, K., Shi, Z., Guo, Y., and Ye, J. (2023). Ob-
ject detection in 20 years: A survey. Proceedings of
the IEEE, 111(3):257–276.
ICAART 2024 - 16th International Conference on Agents and Artificial Intelligence
624