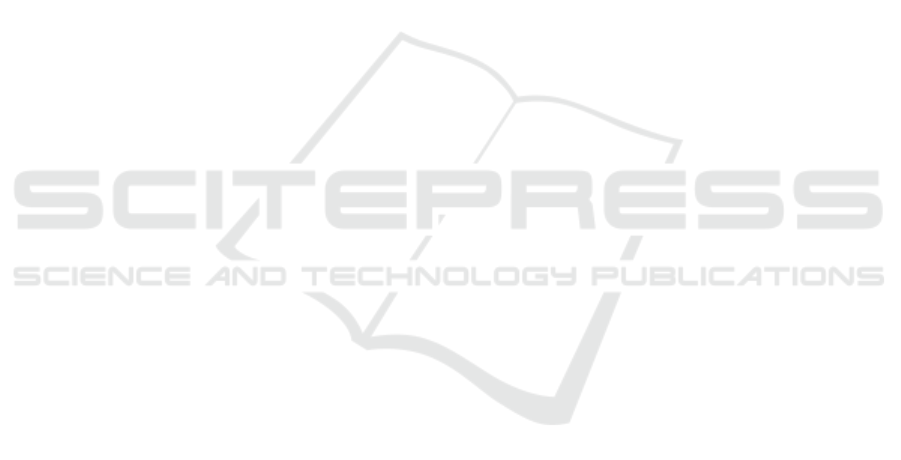
REFERENCES
Ahmad, M., Khan, A. M., Mazzara, M., Distefano, S., Ali,
M., and Sarfraz, M. S. (2022). A fast and compact
3-d cnn for hyperspectral image classification. IEEE
Geoscience and Remote Sensing Letters, 19:1–5.
Ahmed, A. and Reddy, G. (2021). A mobile-based system
for detecting plant leaf diseases using deep learning.
AgriEngineering, 3:478–493.
Bender, F. (2020). Statistical methods for food and agricul-
ture. CRC Press.
Calicioglu, O., Flammini, A., Bracco, S., Bell
`
u, L., and
Sims, R. (2019). The future challenges of food and
agriculture: An integrated analysis of trends and solu-
tions. Sustainability, 11(1):222.
Guo, B.and Gunn, S., Damper, B., and Nelson, J. (2005).
Adaptive band selection for hyperspectral image fu-
sion using mutual information. ” In 2005 7th Interna-
tional Conference on Information Fusion, IEEE, 1.
Harakannanavar, S., Rudagi, J., Puranikmath, V., Siddiqua,
A., and Pramodhini, R. (2022). Plant leaf disease de-
tection using computer vision and machine learning
algorithms. Global Transitions Proceedings, 3:305–
310. International Conference on Intelligent Engi-
neering Approach(ICIEA-2022).
Heble, S., Kumar, A., Prasad, K., Samirana, S.and Rajalak-
shmi, P., and Desai, U. (2018). A low power iot net-
work for smart agriculture. In 2018 IEEE 4th World
Forum on Internet of Things (WF-IoT), pages 609–
614.
Khan, M., Khan, H., Yousaf, A., Khurshid, K., and Ab-
bas, A. (2018). Modern trends in hyperspectral image
analysis: A review. Ieee Access, 6:14118–14129.
Lorena, A., De Carvalho, A., and Gama, J. (2008). A review
on the combination of binary classifiers in multiclass
problems. Artificial Intelligence Review, 30:19–37.
Luo, Y., Zou, J., Yao, C., Zhao, X., Li, T., and Bai, G.
(2018). Hsi-cnn: A novel convolution neural network
for hyperspectral image. In 2018 International Con-
ference on Audio, Language and Image Processing
(ICALIP), pages 464–469. IEEE.
Mc Carthy, U., Uysal, I., Badia-Melis, R., Mercier, S.,
O’Donnell, C., and Ktenioudaki, A. (2018). Global
food security–issues, challenges and technological so-
lutions. Trends in Food Science & Technology, 77:11–
20.
Mogili, U. and Deepak, B. (2018). Review on application
of drone systems in precision agriculture. Procedia
computer science, 113:502–509.
Mohanty, S.P.and Hughes, D. and Salath
´
e, M. (2016). Using
deep learning for image-based plant disease detection.
Frontiers in plant science.
Pereira, L. (2017). Water, agriculture and food: chal-
lenges and issues. Water Resources Management,
31(10):2985–2999.
Ramirez, W., Achanccaray, P.and Mendoza, L., and
Pacheco, M. (2020). Deep convolutional neural net-
works for weed detection in agricultural crops using
optical aerial images. IEEE Latin American GRSS &
ISPRS Remote Sensing Conference (LAGIRS), pages
133–137.
Ravikanth, L., Jayas, D., White, N., Fields, P., and Sun, D.
(2017). Extraction of spectral information from hyper-
spectral data and application of hyperspectral imaging
for food and agricultural products. Food and biopro-
cess technology, 10:1–33.
Rosegrant, M. and Cline, S. (2003). Global food security:
challenges and policies. Science, 302(5652):1917–
1919.
Singh, P., Singh, V., Pandey, M., and Karthikeyan, S.
(2020). One-class classifier ensemble based enhanced
semisupervised classification of hyperspectral remote
sensing images. 2020 International Conference on
Emerging Smart Computing and Informatics (ESCI),
pages 22–27.
Veroustraete, F. (2015). The rise of the drones in agricul-
ture. EC agriculture, 2(2):325–327.
Xu, B., Wang, W., Falzon, G., Kwan, P., Guo, L., Sun, Z.,
and Li, C. (2020). Livestock classification and count-
ing in quadcopter aerial images using mask r-cnn. In-
ternational Journal of Remote Sensing, 41(21):8121–
8142.
Yamamoto, K., Togami, T., and Yamaguchi, N. (2017).
Super-resolution of plant disease images for the ac-
celeration of image-based phenotyping and vigor di-
agnosis in agriculture. Sensors, 17(11):2557.
Zhong, Y., Hu, X., Luo, C., Wang, X., Zhao, J., and Zhang,
L. (2020a). Whu-hi: Uav-borne hyperspectral with
high spatial resolution (h2) benchmark datasets and
classifier for precise crop identification based on deep
convolutional neural network with crf. Remote Sens-
ing of Environment.
Zhong, Y., Hu, X., Luo, C., Wang, X., Zhao, J., and Zhang,
L. (2020b). Whu-hi: Uav-borne hyperspectral with
high spatial resolution (h2) benchmark datasets and
classifier for precise crop identification based on deep
convolutional neural network with crf. Remote Sens-
ing of Environment, 250:112012.
VISAPP 2024 - 19th International Conference on Computer Vision Theory and Applications
566