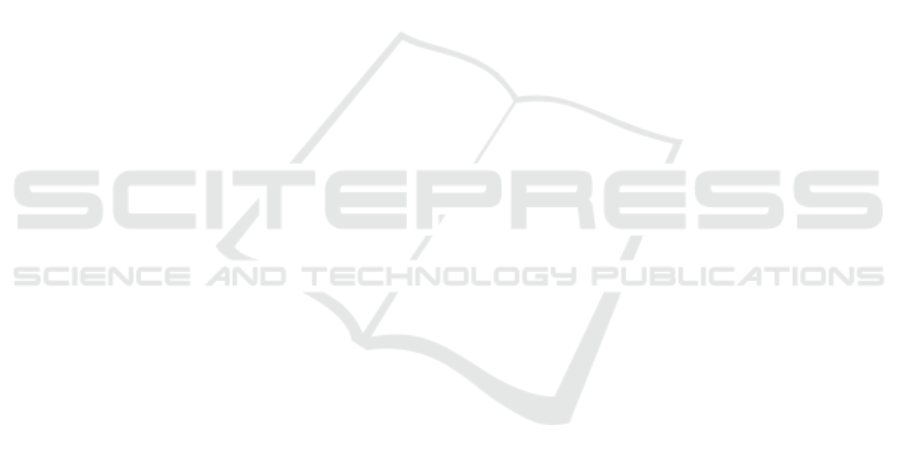
REFERENCES
Agrawal, A. K. and Chakraborty, G. (2023). Neighborhood
component analysis to leverage the class label infor-
mation during feature selection to enhance the damage
classification performance. In Structures, volume 57,
page 105174. Elsevier.
Aksoy, A. and Gunes, M. H. (2016). Operating system clas-
sification performance of tcp/ip protocol headers. In
2016 IEEE 41st Conference on Local Computer Net-
works Workshops (LCN Workshops), pages 112–120.
IEEE.
Aksoy, A. and Gunes, M. H. (2019). Automated iot de-
vice identification using network traffic. In ICC 2019-
2019 IEEE International Conference on Communica-
tions (ICC), pages 1–7. IEEE.
Aksoy, A., Louis, S., and Gunes, M. H. (2017). Operat-
ing system fingerprinting via automated network traf-
fic analysis. In 2017 IEEE Congress on Evolutionary
Computation (CEC), pages 2502–2509. IEEE.
Alghanam, O. A., Almobaideen, W., Saadeh, M., and Ad-
wan, O. (2023). An improved pio feature selection
algorithm for iot network intrusion detection system
based on ensemble learning. Expert Systems with Ap-
plications, 213:118745.
Chhabra, A., Hussien, A. G., and Hashim, F. A. (2023).
Improved bald eagle search algorithm for global opti-
mization and feature selection. Alexandria Engineer-
ing Journal, 68:141–180.
de Oliveira Sementille, L. F. M., Rodrigues, D., de Souuza,
A. N., and Papa, J. P. (2023). Binary flying squirrel
optimizer for feature selection. In Brazilian Confer-
ence on Intelligent Systems, pages 51–64. Springer.
Eskandari, S. and Seifaddini, M. (2023). Online and of-
fline streaming feature selection methods with bat al-
gorithm for redundancy analysis. Pattern Recognition,
133:109007.
Gharehchopogh, F. S., Abdollahzadeh, B., Barshandeh, S.,
and Arasteh, B. (2023). A multi-objective mutation-
based dynamic harris hawks optimization for botnet
detection in iot. Internet of Things, page 100952.
Houssein, E. H., Hosney, M. E., Mohamed, W. M., Ali,
A. A., and Younis, E. M. (2023). Fuzzy-based hunger
games search algorithm for global optimization and
feature selection using medical data. Neural Comput-
ing and Applications, 35(7):5251–5275.
Jin, Y., Xu, H., and Qin, Z. (2023). Intrusion detection
model for software-defined networking based on fea-
ture selection. In Sixth International Conference on
Computer Information Science and Application Tech-
nology (CISAT 2023), volume 12800, pages 428–434.
SPIE.
Keahey, K., Anderson, J., Zhen, Z., Riteau, P., Ruth, P.,
Stanzione, D., Cevik, M., Colleran, J., Gunawi, H. S.,
Hammock, C., Mambretti, J., Barnes, A., Halbach, F.,
Rocha, A., and Stubbs, J. (2020). Lessons learned
from the chameleon testbed. In Proceedings of the
2020 USENIX Annual Technical Conference (USENIX
ATC ’20). USENIX Association.
Maslan, A., Mohamad, K. M. B., Hamid, A., Pangaribuan,
H., and Sitohang, S. (2023). Feature selection to en-
hance ddos detection using hybrid n-gram heuristic
techniques. JOIV: International Journal on Informat-
ics Visualization, 7(3):815–822.
Miettinen, M., Marchal, S., Hafeez, I., Asokan, N., Sadeghi,
A.-R., and Tarkoma, S. (2017). Iot sentinel: Au-
tomated device-type identification for security en-
forcement in iot. In Distributed Computing Systems
(ICDCS), 2017 IEEE 37th International Conference
on, pages 2177–2184. IEEE.
Mishra, D. and Sahu, B. (2011). Feature selection for can-
cer classification: a signal-to-noise ratio approach. In-
ternational Journal of Scientific & Engineering Re-
search, 2(4):1–7.
Nkongolo, M. (2023). Assessing cyclostationary mal-
ware detection via feature selection and classification.
arXiv preprint arXiv:2308.15237.
Patel, R. K. and Giri, V. (2016). Feature selection and classi-
fication of mechanical fault of an induction motor us-
ing random forest classifier. Perspectives in Science,
8:334–337.
Rana, S. and Aksoy, A. (2021). Automated fast-flux detec-
tion using machine learning and genetic algorithms.
In IEEE INFOCOM 2021-IEEE Conference on Com-
puter Communications Workshops (INFOCOM WK-
SHPS), pages 1–6. IEEE.
Sangaiah, A. K., Javadpour, A., Ja’fari, F., Pinto, P., Zhang,
W., and Balasubramanian, S. (2023). A hybrid heuris-
tics artificial intelligence feature selection for intru-
sion detection classifiers in cloud of things. Cluster
Computing, 26(1):599–612.
Sivagaminathan, R. K. and Ramakrishnan, S. (2007). A hy-
brid approach for feature subset selection using neural
networks and ant colony optimization. Expert systems
with applications, 33(1):49–60.
Sun, P., Wang, D., Mok, V. C., and Shi, L. (2019). Compari-
son of feature selection methods and machine learning
classifiers for radiomics analysis in glioma grading.
IEEE Access, 7:102010–102020.
Thakkar, A. and Lohiya, R. (2023). Fusion of statistical im-
portance for feature selection in deep neural network-
based intrusion detection system. Information Fusion,
90:353–363.
Wang, X., Dong, X., Zhang, Y., and Chen, H. (2023).
Crisscross harris hawks optimizer for global tasks
and feature selection. Journal of Bionic Engineering,
20(3):1153–1174.
Wu, Y.-L., Tang, C.-Y., Hor, M.-K., and Wu, P.-F. (2011).
Feature selection using genetic algorithm and clus-
ter validation. Expert Systems with Applications,
38(3):2727–2732.
Xie, W., Wang, L., Yu, K., Shi, T., and Li, W. (2023). Im-
proved multi-layer binary firefly algorithm for opti-
mizing feature selection and classification of microar-
ray data. Biomedical Signal Processing and Control,
79:104080.
Zhu, Y., Li, W., and Li, T. (2023). A hybrid artificial im-
mune optimization for high-dimensional feature se-
lection. Knowledge-Based Systems, 260:110111.
Comparative Analysis of Feature Selection Algorithms for Automated IoT Device Fingerprinting
437