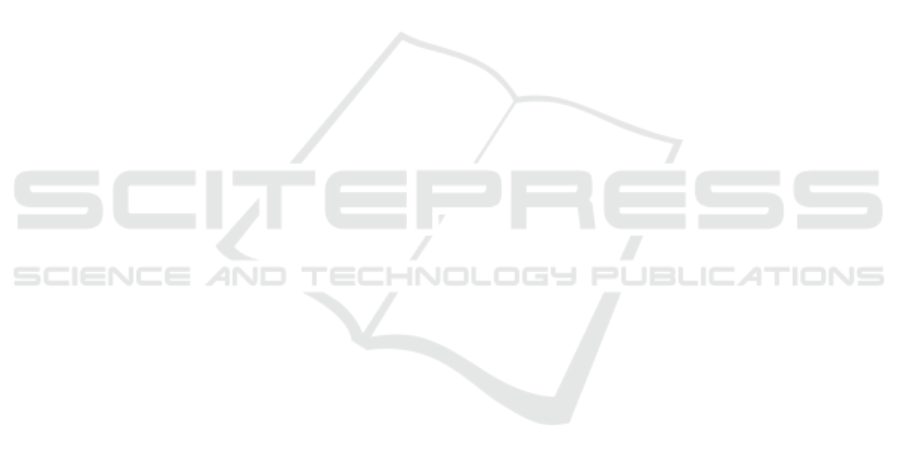
3. The remaining two persons, ∼ 8%, had almost
equally split probabilities to each of the four clus-
ters.
It is however hard to tell which one was correct
since even HR professionals could also have biases,
and be error-prone sometimes.
RQ2: Is anchor and bias removal helpful? When us-
ing the bias and anchoring removal methods proposed
in Section 4.2.2, we observed that results classified
one more person in the same cluster as professionals,
but on the other hand for the rest of 6 persons the av-
erage error difference increased to 0.31.
RQ3: Respondents’ post-evaluation feedback After
finishing the surveys, the 25 persons were asked to
compare it with the ones done face-to-face. Results
show that 21 out of 25 liked more this because they
had more time to think, felt less pressure to give an-
swers, and were satisfied that they had the opportunity
to complete it without prior scheduling from their own
comfort.
6 CONCLUSIONS
After iterating several prototype versions and apply-
ing the framework to our mentioned use case, we
conclude that the proposed method of providing AI
agents to conduct surveys can help organizations in
two ways: a) improve the quality of the results ob-
tained after surveys without having to invest in more
staff to conduct face-to-face surveys, and b) help hu-
man professionals improve alongside AI agents by us-
ing them as assistants during a real-time survey.
ACKNOWLEDGEMENTS
This research was supported by European Union’s
Horizon Europe research and innovation programme
under grant agreement no. 101070455, project DYN-
ABIC.
REFERENCES
Aning, S. and Przybyła-Kasperek, M. (2022). Comparative
study of twoing and entropy criterion for decision tree
classification of dispersed data. Procedia Computer
Science, 207:2434–2443. Proc. of KES2022.
Bajpai, R., Hazarika, D., Singh, K., Gorantla, S., Cam-
bria, E., and Zimmermann, R. (2023). Aspect-
sentiment embeddings for company profiling and em-
ployee opinion mining. In Gelbukh, A., editor, Com-
putational Linguistics and Intelligent Text Processing,
pages 142–160. Springer.
Dee, D. (2006). Bias and data assimilation. PhD thesis,
Shinfield Park, Reading.
Iliescu, D., Ilie, A., and Ispas, D. (2011). Examin-
ing the criterion-related validity of the employee
screening questionnaire: A three-sample investiga-
tion. International Journal of Selection and Assess-
ment, 19(2):222–228.
Khaled, S., El-Tazi, N., and Mokhtar, H. M. O. (2018). De-
tecting fake accounts on social media. In 2018 IEEE
International Conference on Big Data (Big Data),
pages 3672–3681.
Lietz, P. (2010). Research into questionnaire design: A
summary of the literature. International Journal of
Market Research, 52(2):249–272.
Mannens, E., Coppens, S., De Pessemier, T., Dacquin,
H., Deursen, D., Sutter, R., and Van de Walle, R.
(2013). Automatic news recommendations via aggre-
gated profiling. Multimedia Tools and Applications -
MTA, 63.
Ni, X., Zeng, S., Qin, R., Li, J., Yuan, Y., and Wang, F.-Y.
(2017). Behavioral profiling for employees using so-
cial media: A case study based on wechat. In 2017
Chinese Automation Congress (CAC), pages 7725–
7730.
Park, H. and Faradonbeh, M. K. S. (2022). Efficient algo-
rithms for learning to control bandits with unobserved
contexts. IFAC-PapersOnLine, 55(12):383–388. 14th
IFAC Workshop on Adaptive and Learning Control
Systems ALCOS 2022.
Rafae, A. and Erritali, M. (2023). Using a profiling sys-
tem to recommend employees to carry out a project.
Electronics, 12(16).
Samuel Farley, Daniella Mokhtar, K. N. and Niven, K.
(2023). What influences the relationship between
workplace bullying and employee well-being? a
systematic review of moderators. Work & Stress,
37(3):345–372.
Schermer, B. W. (2011). The limits of privacy in automated
profiling and data mining. Computer Law and Secu-
rity Review, 27(1):45–52.
Staale Einarsen, H. H. and Notelaers, G. (2009). Measuring
exposure to bullying and harassment at work: Valid-
ity, factor structure and psychometric properties of the
negative acts questionnaire-revised. Work & Stress,
23(1):24–44.
Wibawa, A. D., Amri, A. M., Mas, A., and Iman, S. (2022).
Text mining for employee candidates automatic profil-
ing based on application documents. EMITTER Inter-
national Journal of Engineering Technology, 10:47–
62.
Xia, P., Zhang, L., and Li, F. (2015). Learning similar-
ity with cosine similarity ensemble. Information sci-
ences, 307:39–52.
Yan, T., Keusch, F., and He, L. (2018). The impact of
question and scale characteristics on scale direction
effects. Survey Practice, 11(2).
ICAART 2024 - 16th International Conference on Agents and Artificial Intelligence
640