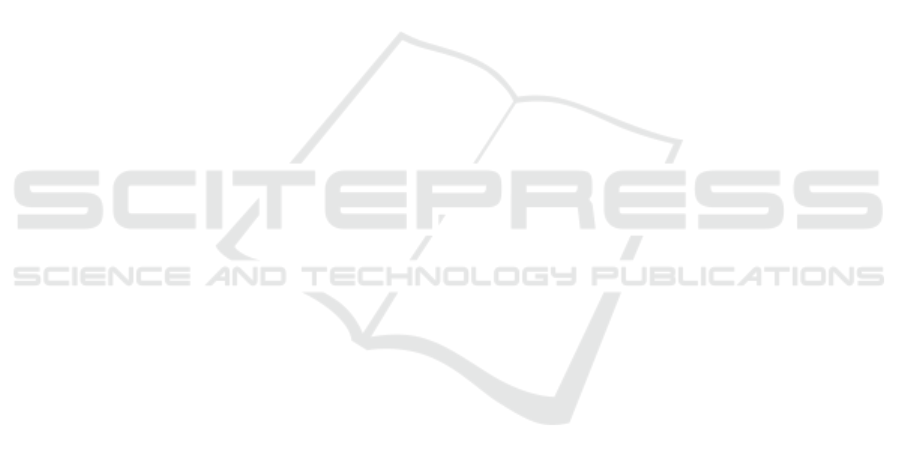
nally, the work measures the severity of the disease
using a k-means clustering algorithm. Further inves-
tigation may be carried out with this system for other
leaf diseases that cause color variations in the lesion
area.
REFERENCES
Azadbakht, M., Ashourloo, D., Aghighi, H., Radiom, S.,
and Alimohammadi, A. (2019). Wheat leaf rust detec-
tion at canopy scale under different LAI levels using
machine learning techniques. Computers and Elec-
tronics in Agriculture, 156:119–128.
Caubel, J., Launay, M., Ripoche, D., Gouache, D., Buis,
S., Huard, F., Huber, L., Brun, F., and Bancal,
M. O. (2017). Climate change effects on leaf rust of
wheat: Implementing a coupled crop-disease model
in a french regional application. European Journal of
Agronomy, 90:53–66.
Deutsch, D. (1985). Quantum theory, the church–turing
principle and the universal quantum computer. Pro-
ceedings of the Royal Society of London. A. Mathe-
matical and Physical Sciences, 400(1818):97–117.
Erenstein, O., Jaleta, M., Mottaleb, K. A., Sonder, K.,
Donovan, J., and Braun, H.-J. (2022). Global trends
in wheat production, consumption and trade. In
Wheat Improvement, pages 47–66. Springer Interna-
tional Publishing.
Figueroa, M., Hammond-Kosack, K. E., and Solomon, P. S.
(2017). A review of wheat diseases-a field perspec-
tive. Molecular Plant Pathology, 19(6):1523–1536.
Goldberg, D. E. (1989). Genetic Algorithms in Search, Op-
timization and Machine Learning. Addison-Wesley
Longman Publishing Co., Inc., USA, 1st edition.
Gozali, A. A., Kurniawan, B., Weng, W., and Fujimura, S.
(2019). Solving university course timetabling problem
using localized island model genetic algorithm with
dual dynamic migration policy. IEEJ Transactions
on Electrical and Electronic Engineering, 15(3):389–
400.
Han, K.-H. and Kim, J.-H. (2002). Quantum-inspired evo-
lutionary algorithm for a class of combinatorial opti-
mization. IEEE Transactions on Evolutionary Com-
putation, 6(6):580–593.
Haralick, R. M., Shanmugam, K., and Dinstein, I. (1973).
Textural features for image classification. IEEE
Transactions on Systems, Man and Cybernetics,
3(6):610–621.
Khan, H., Haq, I. U., Munsif, M., Mustaqeem, Khan,
S. U., and Lee, M. Y. (2022). Automated wheat
diseases classification framework using advanced ma-
chine learning technique. Agriculture, 12(8):1226.
Kukreja, V. and Kumar, D. (2021). Automatic classification
of wheat rust diseases using deep convolutional neu-
ral networks. In 2021 9th International Conference
on Reliability, Infocom Technologies and Optimiza-
tion (Trends and Future Directions) (ICRITO). IEEE.
Kumar, S. N., Aggarwal, P., Rani, D. S., Saxena, R.,
Chauhan, N., and Jain, S. (2014). Vulnerability of
wheat production to climate change in india. Climate
Research, 59(3):173–187.
Kurdi, M. (2016). An effective new island model genetic al-
gorithm for job shop scheduling problem. Computers
& Operations Research, 67:132–142.
Lipper, L., McCarthy, N., Zilberman, D., Asfaw, S., and
Branca, G., editors (2018). Climate Smart Agricul-
ture. Springer International Publishing.
Liu, Y., Ghandar, A., and Theodoropoulos, G. (2019). Is-
land model genetic algorithm for feature selection in
non-traditional credit risk evaluation. In 2019 IEEE
Congress on Evolutionary Computation (CEC). IEEE.
Mendeley (2020). Wheat nitrogen defi-
ciency and leaf rust image dataset. url-
https://data.mendeley.com/datasets/th422bg4yd/1.
Accessed: (20/04/2023).
Mottaleb, K. A., Kruseman, G., Frija, A., Sonder, K., and
Lopez-Ridaura, S. (2023). Projecting wheat demand
in china and india for 2030 and 2050: Implications for
food security. Frontiers in Nutrition, 9.
Ortiz, A., G
´
orriz, J. M., Ram
´
ırez, J., Salas-Gonz
´
alez,
D., and Llamas-Elvira, J. M. (2013). Two fully-
unsupervised methods for MR brain segmentation us-
ing SOM-based strategies. Applied Soft Computing,
13(5):2668–2682.
Shafi, U., Mumtaz, R., Qureshi, M. D. M., Mahmood, Z.,
Tanveer, S. K., Haq, I. U., and Zaidi, S. M. H. (2023).
Embedded AI for wheat yellow rust infection type
classification. IEEE Access, 11:23726–23738.
Xu, P., Wu, G., Guo, Y., chen, X., Yang, H., and Zhang, R.
(2017). Automatic wheat leaf rust detection and grad-
ing diagnosis via embedded image processing system.
Procedia Computer Science, 107:836–841.
Zhao, J., Fang, Y., Chu, G., Yan, H., Hu, L., and Huang,
L. (2020). Identification of leaf-scale wheat powdery
mildew (blumeria graminis f. sp. tritici) combining
hyperspectral imaging and an SVM classifier. Plants,
9(8):936.
Zinke-Wehlmann, C. and Charv
´
at, K. (2021). Introduc-
tion of smart agriculture. In Big Data in Bioeconomy,
pages 187–190. Springer International Publishing.
Feature Selection Using Quantum Inspired Island Model Genetic Algorithm for Wheat Rust Disease Detection and Severity Estimation
499