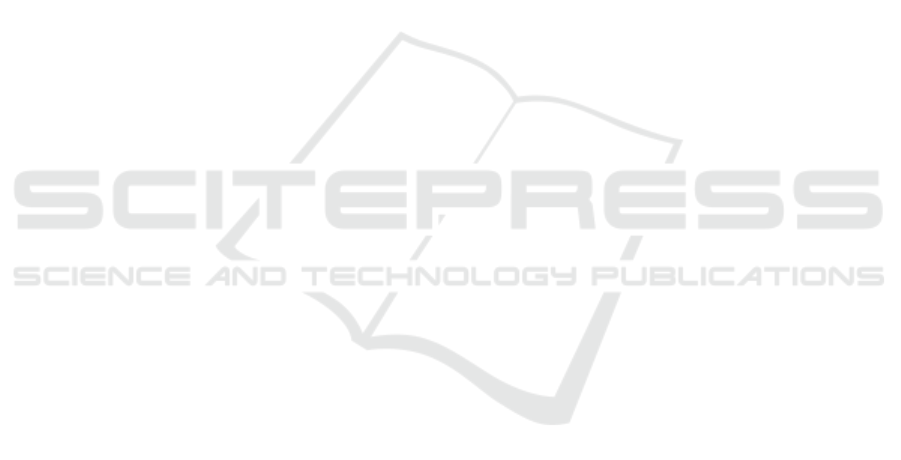
on test splits and unseen datasets. Our technique can
easily be applied to other DNN architectures, appli-
cation domains and tasks (such as, object detection);
more importantly, it can also be adapted to other loss
functions.
REFERENCES
Abdolhoseini, M. et al. (2019). Segmentation of heavily
clustered nuclei from histopathological images. Sci-
entific reports, 9(1):4551.
Abraham, N. and Khan, N. M. (2018). A novel focal tversky
loss function with improved attention u-net for lesion
segmentation.
Chen, L.-C. et al. (2017). Rethinking atrous convolution for
semantic image segmentation.
Doan, T. N. N. et al. (2022). Gradmix for nuclei segmenta-
tion and classification in imbalanced pathology image
datasets.
Dogar, G. M. et al. (2023). Attention augmented distance
regression and classification network for nuclei in-
stance segmentation and type classification in histol-
ogy images. Biomedical Signal Processing and Con-
trol, 79:104199.
Gamper, J. et al. (2020). Pannuke dataset extension, insights
and baselines.
Graham, S. et al. (2019). Hover-net: Simultaneous seg-
mentation and classification of nuclei in multi-tissue
histology images.
Kirillov, A. et al. (2019). Panoptic segmentation. In Pro-
ceedings of the IEEE/CVF conference on computer vi-
sion and pattern recognition, pages 9404–9413.
Kumar, N. et al. (2017). A dataset and a technique for
generalized nuclear segmentation for computational
pathology. IEEE transactions on medical imaging,
36(7):1550–1560.
Li, Z. et al. (2019). Exploiting coarse-to-fine task transfer
for aspect-level sentiment classification. In Proceed-
ings of the AAAI conference on artificial intelligence,
volume 33, pages 4253–4260.
Lin, T.-Y. et al. (2017). Focal loss for dense object detec-
tion. In Proceedings of the IEEE International Con-
ference on Computer Vision (ICCV).
Mahbod, A. et al. (2021). Cryonuseg: A dataset for
nuclei instance segmentation of cryosectioned h&e-
stained histological images. Computers in biology and
medicine, 132:104349.
Oh, H.-J. and Jeong, W.-K. (2023). Diffmix: Diffu-
sion model-based data synthesis for nuclei segmenta-
tion and classification in imbalanced pathology image
datasets.
Pan, X. et al. (2023). Smile: Cost-sensitive multi-task
learning for nuclear segmentation and classification
with imbalanced annotations. Medical Image Anal-
ysis, 88:102867.
Reis, H. C. and Turk, V. (2023). Transfer learning approach
and nucleus segmentation with medclnet colon cancer
database. Journal of Digital Imaging, 36(1):306–325.
Ronneberger, O. et al. (2015). U-net: Convolutional
networks for biomedical image segmentation. In
Medical Image Computing and Computer-Assisted
Intervention–MICCAI 2015: 18th International Con-
ference, Munich, Germany, October 5-9, 2015, Pro-
ceedings, Part III 18, pages 234–241. Springer.
Schmidt, U., Weigert, M., et al. (2018). Cell detection with
star-convex polygons. In Medical Image Computing
and Computer Assisted Intervention – MICCAI 2018,
pages 265–273. Springer International Publishing.
Sudre, C. H. et al. (2017). Generalised dice overlap
as a deep learning loss function for highly unbal-
anced segmentations. In Deep Learning in Medical
Image Analysis and Multimodal Learning for Clini-
cal Decision Support: Third International Workshop,
DLMIA 2017, and 7th International Workshop, ML-
CDS 2017, Held in Conjunction with MICCAI 2017,
Qu
´
ebec City, QC, Canada, September 14, Proceed-
ings 3, pages 240–248. Springer.
Verma, R. et al. (2021). Monusac2020: A multi-organ nu-
clei segmentation and classification challenge. IEEE
Transactions on Medical Imaging, 40(12):3413–3423.
Weigert, M. and Schmidt, U. (2022). Nuclei instance seg-
mentation and classification in histopathology images
with stardist. In 2022 IEEE International Symposium
on Biomedical Imaging Challenges (ISBIC). IEEE.
Ye, Z. et al. (2024). Dsca-net: Double-stage codec attention
network for automatic nuclear segmentation. Biomed-
ical Signal Processing and Control, 88:105569.
Yosinski, J. et al. (2014). How transferable are features in
deep neural networks? Advances in neural informa-
tion processing systems, 27.
Zhang, Z. et al. (2014). Facial landmark detection by
deep multi-task learning. In Computer Vision–ECCV
2014: 13th European Conference, Zurich, Switzer-
land, September 6-12, 2014, Proceedings, Part VI 13,
pages 94–108. Springer.
Zhao, H. et al. (2017). Pyramid scene parsing network. In
Proceedings of the IEEE conference on computer vi-
sion and pattern recognition, pages 2881–2890.
Zhao, T. et al. (2023). Gsn-hvnet: A lightweight, multi-task
deep learning framework for nuclei segmentation and
classification. Bioengineering, 10(3):393.
Zhou, Z. et al. (2018). Unet++: A nested u-net architec-
ture for medical image segmentation. In Deep Learn-
ing in Medical Image Analysis and Multimodal Learn-
ing for Clinical Decision Support: 4th International
Workshop, DLMIA 2018, and 8th International Work-
shop, ML-CDS 2018, Held in Conjunction with MIC-
CAI 2018, Granada, Spain, September 20, 2018, Pro-
ceedings 4, pages 3–11. Springer.
BIOIMAGING 2024 - 11th International Conference on Bioimaging
288