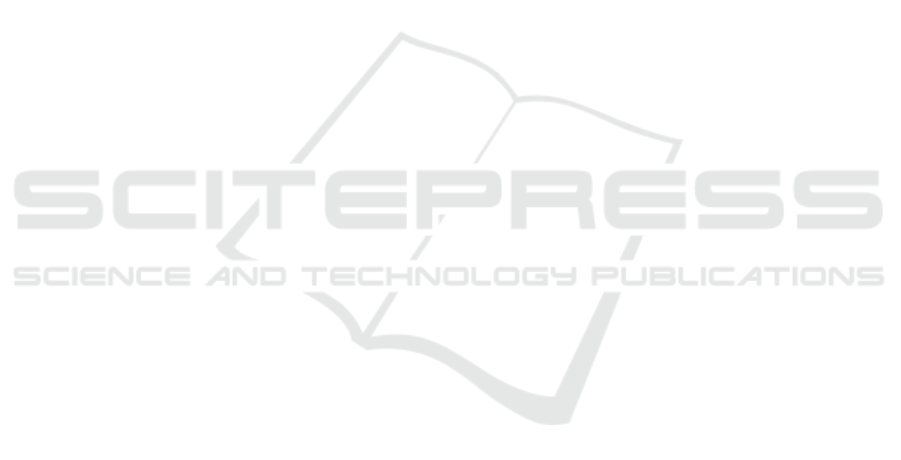
tude embedding as a purely quantum architecture and
a purely classical NN on the compressed and uncom-
pressed input.
Our results suggest that the classification perfor-
mance in hybrid transfer learning is mainly influenced
by the classifying compression layer and that the ac-
tual contribution of the VQC may be doubted. Ad-
ditionally, these approaches yield better results than
models where solely the VQC classifies.
Even though our model performs worse on aver-
age than the hybrid transfer learning models DQC and
SEQUENT, it allows for a more transparent and inter-
pretable analysis of the quantum circuit’s role in the
machine learning task because of the clear distinction
between the components. Additionally, our research
indicates that our approach with angle embedding on
the compressed input is a valid alternative to a VQC
with amplitude embedding on the original input.
ACKNOWLEDGEMENTS
This work is part of the Munich Quantum Valley,
which is supported by the Bavarian state government
with funds from the Hightech Agenda Bayern Plus.
This paper was partially funded by the German Fed-
eral Ministry for Economic Affairs and Climate Ac-
tion through the funding program ”Quantum Comput-
ing – Applications for the industry” based on the al-
lowance ”Development of digital technologies” (con-
tract number: 01MQ22008A).
REFERENCES
Altmann, P., S
¨
unkel, L., Stein, J., M
¨
uller, T., Roch, C.,
and Linnhoff-Popien, C. (2023). SEQUENT: Towards
Traceable Quantum Machine Learning using Sequen-
tial Quantum Enhanced Training. arXiv:2301.02601
[quant-ph].
Becker, S., Ackermann, M., Lapuschkin, S., M
¨
uller, K.-
R., and Samek, W. (2019). Interpreting and Explain-
ing Deep Neural Networks for Classification of Audio
Signals. arXiv:1807.03418 [cs, eess].
Bellman, R. (1957). Dynamic Programming. Princeton
University Press, Princeton, NJ.
Cerezo, M., Arrasmith, A., Babbush, R., Benjamin, S. C.,
Endo, S., Fujii, K., McClean, J. R., Mitarai, K.,
Yuan, X., Cincio, L., and Coles, P. J. (2021). Varia-
tional Quantum Algorithms. Nature Reviews Physics,
3(9):625–644. arXiv:2012.09265 [quant-ph, stat].
Dosovitskiy, A., Beyer, L., Kolesnikov, A., Weissenborn,
D., Zhai, X., Unterthiner, T., Dehghani, M., Minderer,
M., Heigold, G., Gelly, S., Uszkoreit, J., and Houlsby,
N. (2021). An Image is Worth 16x16 Words: Trans-
formers for Image Recognition at Scale. In Interna-
tional Conference on Learning Representations.
Farhi, E. and Neven, H. (2018). Classification with
Quantum Neural Networks on Near Term Processors.
arXiv:1802.06002 [quant-ph].
Goodfellow, I., Bengio, Y., and Courville, A. (2016). Deep
Learning. MIT Press.
Kingma, D. P. and Ba, J. (2015). Adam: A Method for
Stochastic Optimization. In Bengio, Y. and LeCun,
Y., editors, 3rd International Conference on Learn-
ing Representations, ICLR 2015, San Diego, CA, USA,
May 7-9, 2015, Conference Track Proceedings.
Krizhevsky, A., Sutskever, I., and Hinton, G. E. (2017). Im-
ageNet classification with deep convolutional neural
networks. Communications of the ACM, 60(6):84–90.
K
¨
olle, M., Giovagnoli, A., Stein, J., Mansky, M. B., Hager,
J., and Linnhoff-Popien, C. (2023a). Improving Con-
vergence for Quantum Variational Classifiers using
Weight Re-Mapping. arXiv:2212.14807 [quant-ph].
K
¨
olle, M., Giovagnoli, A., Stein, J., Mansky, M. B., Hager,
J., Rohe, T., M
¨
uller, R., and Linnhoff-Popien, C.
(2023b). Weight Re-Mapping for Variational Quan-
tum Algorithms. arXiv:2306.05776 [quant-ph].
LeCun, Y., Bottou, L., Bengio, Y., and Haffner, P. (1998).
Gradient-based learning applied to document recogni-
tion. Proceedings of the IEEE, 86(11):2278–2324.
Lohweg, V. (2013). banknote authentication. Published:
UCI Machine Learning Repository.
Mari, A., Bromley, T. R., Izaac, J., Schuld, M., and Killo-
ran, N. (2020). Transfer learning in hybrid classical-
quantum neural networks. Quantum, 4:340.
Nair, V. and Hinton, G. E. (2010). Rectified Linear Units
Improve Restricted Boltzmann Machines. In Proceed-
ings of the 27th International Conference on Interna-
tional Conference on Machine Learning, ICML’10,
pages 807–814, Madison, WI, USA. Omnipress.
Preskill, J. (2018). Quantum Computing in the NISQ
era and beyond. Quantum, 2:79. arXiv:1801.00862
[cond-mat, physics:quant-ph].
Ramachandran, P., Zoph, B., and Le, Q. V. (2017). Search-
ing for Activation Functions. arXiv:1710.05941 [cs].
Schneider, S., Baevski, A., Collobert, R., and Auli, M.
(2019). wav2vec: Unsupervised Pre-Training for
Speech Recognition. In Interspeech 2019, pages
3465–3469. ISCA.
Schuld, M., Bocharov, A., Svore, K., and Wiebe, N. (2020).
Circuit-centric quantum classifiers. Physical Review
A, 101(3):032308. arXiv:1804.00633 [quant-ph].
Schuld, M. and Petruccione, F. (2018). Supervised Learn-
ing with Quantum Computers. Quantum Science and
Technology. Springer Cham, 1 edition.
Wolberg, W. H., Mangasarian, O. L., and W. Nick Street
(1995). Breast Cancer Wisconsin (Diagnostic). Pub-
lished: UCI Machine Learning Repository.
ICAART 2024 - 16th International Conference on Agents and Artificial Intelligence
656