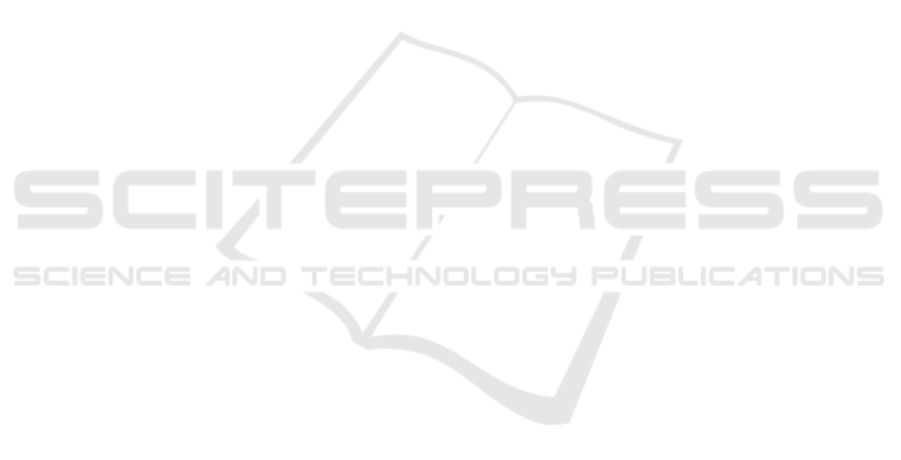
more layers for the VQCs than previous works (Chen
et al., 2022), as they have yielded better results in the
experiments.
In the future, the VQC results could be run not
only on a quantum simulator, but on real quantum
hardware to determine which and if there is a differ-
ence. Also, a comparison of the VQC approach with a
gradient based neural network would be an option for
future work. Another option would be to compare the
VQC approach in terms of the number of parameters
with a data reuploading method and see if this can
solve the coin game similarly well with even fewer
qubits. Additionally, we could work on the hyperpa-
rameters and see if even better results can be achieved
by adapting them.
ACKNOWLEDGEMENTS
This work is part of the Munich Quantum Valley,
which is supported by the Bavarian state government
with funds from the Hightech Agenda Bayern Plus.
REFERENCES
Badia, A. P., Piot, B., Kapturowski, S., Sprechmann, P.,
Vitvitskyi, A., Guo, Z. D., and Blundell, C. (2020).
Agent57: Outperforming the atari human benchmark.
CoRR, abs/2003.13350.
Caldas, L. G. and Norford, L. K. (2002). A design optimiza-
tion tool based on a genetic algorithm. Automation in
construction, 11(2):173–184.
Chen, S. Y. and Goan, H. (2019). Variational quantum
circuits and deep reinforcement learning. CoRR,
abs/1907.00397.
Chen, S. Y.-C. (2022). Quantum deep recurrent reinforce-
ment learning.
Chen, S. Y.-C., Huang, C.-M., Hsing, C.-W., Goan, H.-S.,
and Kao, Y.-J. (2022). Variational quantum reinforce-
ment learning via evolutionary optimization. Machine
Learning: Science and Technology, 3(1):015025.
Deng, S., Xiang, Z., Zhao, P., Taheri, J., Gao, H., Yin, J.,
and Zomaya, A. Y. (2020). Dynamical resource allo-
cation in edge for trustable internet-of-things systems:
A reinforcement learning method. IEEE Transactions
on Industrial Informatics, 16(9):6103–6113.
Dimeas, A. L. and Hatziargyriou, N. D. (2010). Multi-agent
reinforcement learning for microgrids. In IEEE PES
General Meeting, pages 1–8.
Ding, S., Su, C., and Yu, J. (2011). An optimizing bp neural
network algorithm based on genetic algorithm. Artifi-
cial intelligence review, 36:153–162.
Eiben, A. E. and Smith, J. E. (2015). Introduction to evolu-
tionary computing. Springer.
Foerster, J., Chen, R. Y., Al-Shedivat, M., Whiteson, S.,
Abbeel, P., and Mordatch, I. (2018). Learning with
Opponent-Learning Awareness. In Proceedings of the
17th International Conference on Autonomous Agents
and Multiagent Systems, page 122–130, Richland, SC.
International Foundation for Autonomous Agents and
Multiagent Systems.
Franz, M., Wolf, L., Periyasamy, M., Ufrecht, C., Scherer,
D. D., Plinge, A., Mutschler, C., and Mauerer,
W. (2022). Uncovering instabilities in variational-
quantum deep q-networks. Journal of the Franklin
Institute.
Gabor, T. and Altmann, P. (2019). Benchmarking surrogate-
assisted genetic recommender systems. In Proceed-
ings of the Genetic and Evolutionary Computation
Conference Companion, pages 1568–1575.
Harrow, A. W. and Montanaro, A. (2017). Quantum com-
putational supremacy. Nature, 549(7671):203–209.
Hernandez-Leal, P., Kaisers, M., Baarslag, T., and de Cote,
E. M. (2017). A Survey of Learning in Multiagent
Environments: Dealing with Non-Stationarity. arXiv
preprint arXiv:1707.09183.
Holland, J. H. and Miller, J. H. (1991). Artificial adaptive
agents in economic theory. The American economic
review, 81(2):365–370.
Kwak, Y., Yun, W. J., Jung, S., Kim, J.-K., and Kim, J.
(2021). Introduction to quantum reinforcement learn-
ing: Theory and pennylane-based implementation.
Laurent, G. J., Matignon, L., Fort-Piat, L., et al. (2011). The
world of independent learners is not markovian. Inter-
national Journal of Knowledge-based and Intelligent
Engineering Systems, 15(1):55–64.
Leibo, J. Z., Zambaldi, V., Lanctot, M., Marecki, J.,
and Graepel, T. (2017a). Multi-Agent Reinforce-
ment Learning in Sequential Social Dilemmas. In
Proceedings of the 16th Conference on Autonomous
Agents and Multiagent Systems, AAMAS ’17, page
464–473, Richland, SC. International Foundation for
Autonomous Agents and Multiagent Systems.
Leibo, J. Z., Zambaldi, V. F., Lanctot, M., Marecki, J.,
and Graepel, T. (2017b). Multi-agent reinforce-
ment learning in sequential social dilemmas. CoRR,
abs/1702.03037.
Lerer, A. and Peysakhovich, A. (2017). Maintain-
ing Cooperation in Complex Social Dilemmas us-
ing Deep Reinforcement Learning. arXiv preprint
arXiv:1707.01068.
Littman, M. L. (1994). Markov Games as a Framework
for Multi-Agent Reinforcement Learning. In Machine
Learning Proceedings 1994, pages 157–163. Morgan
Kaufmann, San Francisco (CA).
Lukac, M. and Perkowski, M. (2002). Evolving quan-
tum circuits using genetic algorithm. In Proceedings
2002 NASA/DoD Conference on Evolvable Hardware,
pages 177–185. IEEE.
McMahon, D. (2007). Quantum computing explained. John
Wiley & Sons.
Mottonen, M., Vartiainen, J. J., Bergholm, V., and Salomaa,
M. M. (2004). Transformation of quantum states using
Multi-Agent Quantum Reinforcement Learning Using Evolutionary Optimization
81