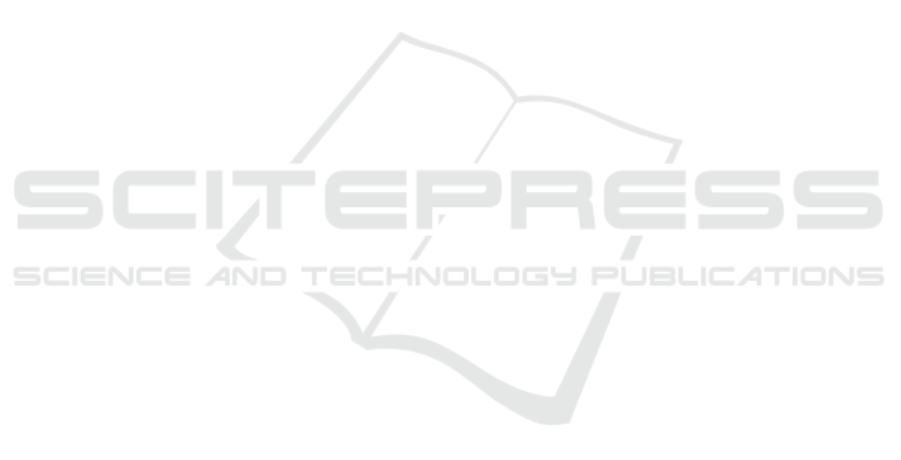
and web news mining to predict covid-19 outbreak.
Asian Pacific journal of tropical medicine, 13(8):378.
Jiang, K., Feng, S., Song, Q., Calix, R. A., Gupta, M., and
Bernard, G. R. (2018). Identifying tweets of personal
health experience through word embedding and lstm
neural network. BMC bioinformatics, 19(8):67–74.
Karisani, P. and Agichtein, E. (2018). Did you really just
have a heart attack? towards robust detection of per-
sonal health mentions in social media. In Proceedings
of the 2018 World Wide Web Conference, pages 137–
146.
Khan, P. I., Razzak, I., Dengel, A., and Ahmed, S.
(2020). Improving personal health mention detection
on twitter using permutation based word representa-
tion learning. In International Conference on Neural
Information Processing, pages 776–785. Springer.
Khan, P. I., Razzak, I., Dengel, A., and Ahmed, S. (2022a).
A novel approach to train diverse types of language
models for health mention classification of tweets. In
Artificial Neural Networks and Machine Learning–
ICANN 2022: 31st International Conference on Ar-
tificial Neural Networks, Bristol, UK, September 6–9,
2022, Proceedings, Part II, pages 136–147. Springer.
Khan, P. I., Siddiqui, S. A., Razzak, I., Dengel, A., and
Ahmed, S. (2022b). Improving health mention classi-
fication of social media content using contrastive ad-
versarial training. IEEE Access, 10:87900–87910.
Li, L., Jiang, Y., and Huang, B. (2021). Long-term pre-
diction for temporal propagation of seasonal influenza
using transformer-based model. Journal of biomedi-
cal informatics, 122:103894.
Liu, Y., Ott, M., Goyal, N., Du, J., Joshi, M., Chen, D.,
Levy, O., Lewis, M., Zettlemoyer, L., and Stoyanov,
V. (2019). Roberta: A robustly optimized bert pre-
training approach. arXiv preprint arXiv:1907.11692.
Loshchilov, I. and Hutter, F. (2018). Fixing weight
decay regularization in adam. arXiv preprint
arXiv:2011.08042v1.
Luo, L., Wang, Y., and Liu, H. (2022). Covid-19 personal
health mention detection from tweets using dual con-
volutional neural network. Expert Systems with Appli-
cations, 200:117139.
Luo, X., Gandhi, P., Storey, S., and Huang, K. (2021). A
deep language model for symptom extraction from
clinical text and its application to extract covid-
19 symptoms from social media. IEEE Journal
of Biomedical and Health Informatics, 26(4):1737–
1748.
Maneriker, P., Stokes, J. W., Lazo, E. G., Carutasu, D., Ta-
jaddodianfar, F., and Gururajan, A. (2021). Urltran:
Improving phishing url detection using transformers.
In MILCOM 2021-2021 IEEE Military Communica-
tions Conference (MILCOM), pages 197–204. IEEE.
Mozafari, M., Farahbakhsh, R., and Crespi, N. (2020). A
bert-based transfer learning approach for hate speech
detection in online social media. In Complex Networks
and Their Applications VIII: Volume 1 Proceedings
of the Eighth International Conference on Complex
Networks and Their Applications COMPLEX NET-
WORKS 2019 8, pages 928–940. Springer.
Naseem, U., Kim, J., Khushi, M., and Dunn, A. G. (2022a).
Identification of disease or symptom terms in reddit to
improve health mention classification. In Proceedings
of the ACM Web Conference 2022, pages 2573–2581.
Naseem, U., Kim, J., Khushi, M., and Dunn, A. G. (2022b).
Robust identification of figurative language in per-
sonal health mentions on twitter. IEEE Transactions
on Artificial Intelligence.
Naseem, U., Lee, B. C., Khushi, M., Kim, J., and Dunn,
A. G. (2022c). Benchmarking for public health
surveillance tasks on social media with a domain-
specific pretrained language model. arXiv preprint
arXiv:2204.04521.
Radford, A., Narasimhan, K., Salimans, T., and Sutskever,
I. (2018). Improving language understanding by gen-
erative pre-training.
Ray, P. and Chakrabarti, A. (2017). Twitter sentiment anal-
ysis for product review using lexicon method. In
2017 International Conference on Data Management,
Analytics and Innovation (ICDMAI), pages 211–216.
IEEE.
Tariq, A., Mehmood, A., Elhadef, M., and Khan, M. U. G.
(2022). Adversarial training for fake news classifica-
tion. IEEE Access, 10:82706–82715.
Wassan, S., Chen, X., Shen, T., Waqar, M., and Jhanjhi,
N. (2021). Amazon product sentiment analysis us-
ing machine learning techniques. Revista Argentina
de Cl
´
ınica Psicol
´
ogica, 30(1):695.
Yang, F., Wang, W., Wang, F., Fang, Y., Tang, D., Huang, J.,
Lu, H., and Yao, J. (2022). scbert as a large-scale pre-
trained deep language model for cell type annotation
of single-cell rna-seq data. Nature Machine Intelli-
gence, 4(10):852–866.
Yang, Z., Dai, Z., Yang, Y., Carbonell, J., Salakhutdinov,
R. R., and Le, Q. V. (2019). Xlnet: Generalized au-
toregressive pretraining for language understanding.
In Advances in neural information processing sys-
tems, pages 5754–5764.
Zbontar, J., Jing, L., Misra, I., LeCun, Y., and Deny, S.
(2021). Barlow twins: Self-supervised learning via
redundancy reduction. In International Conference on
Machine Learning, pages 12310–12320. PMLR.
Zeng, W., Gautam, A., and Huson, D. H. (2023). Mulan-
methyl-multiple transformer-based language models
for accurate dna methylation prediction. bioRxiv,
pages 2023–01.
ICAART 2024 - 16th International Conference on Agents and Artificial Intelligence
680