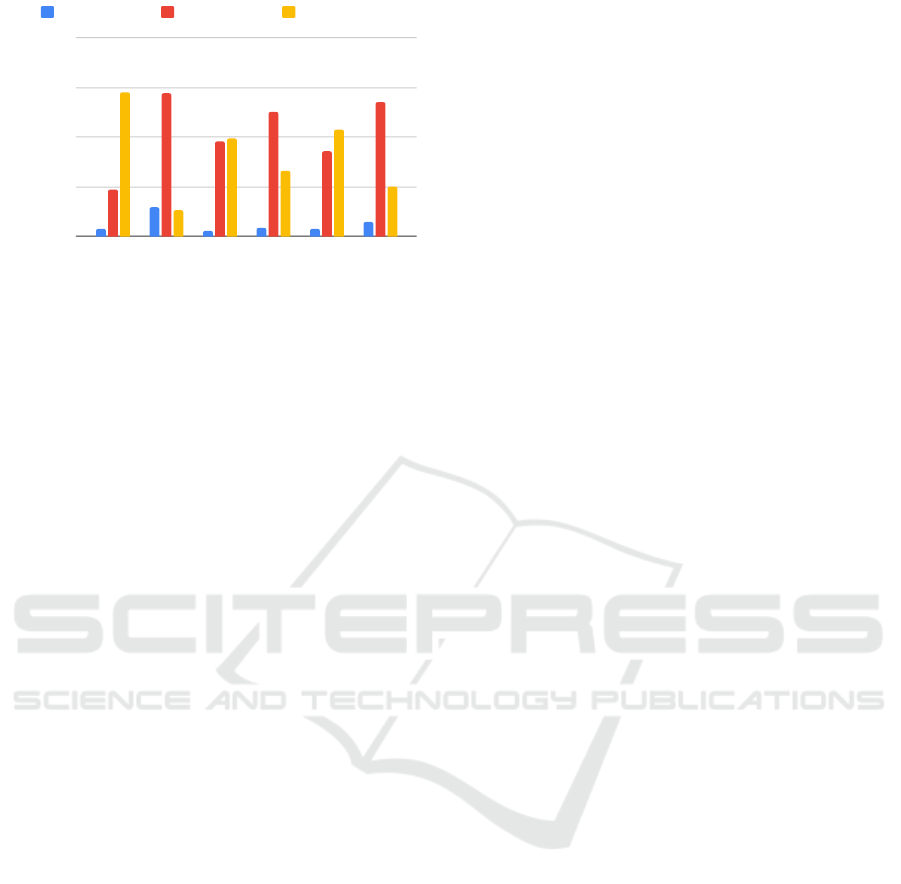
Constructs of Trust
Users Percentage
0
25
50
75
100
Competence
Integrity
Benevolence
Transparency
Re-use
Overall
Explanation A Explanation B Explanation C
Figure 12: Experiment Results.
Re-use, implying that utilizing the DeepAI API en-
riched explanations with additional movie-related in-
formation, making them more adaptable and likely to
be chosen. These findings support our hypothesis that
diverse users prefer different explanations, underscor-
ing the need to provide users with control over their
choice to accommodate varied informational needs.
6 CONCLUDING REMARKS
In this paper, we have introduced a comprehensive
taxonomy of context that finds relevance across di-
verse domains and systems. To practically real-
ize context-sensitive explanations, we have presented
ConEX, a general framework founded on our con-
text conceptualization, along with the incorporation
of a post-hoc explainer. We presented an application
of ConEX that leverages context-sensitive explana-
tions to enhance the personalization of movie recom-
mendations. Additionally, we conducted a user study
to demonstrate that context-sensitive explanations en-
hance user trust and satisfaction empirically. Future
work in this domain can include research into au-
tomated situation recognition, reducing users’ input,
and tracking their current state. Moreover, address-
ing the challenge of temporal changes in user prefer-
ences and maintaining the context model’s accuracy
over time is a promising avenue for future research.
REFERENCES
Apicella, A., Giugliano, S., Isgr
`
o, F., and Prevete, R.
(2022). Exploiting auto-encoders and segmenta-
tion methods for middle-level explanations of image
classification systems. Knowledge-Based Systems,
255:109725.
Berkovsky, S., Taib, R., and Conway, D. (2017). How to
recommend? user trust factors in movie recommender
systems. In Proceedings of the 22nd International
Conference on Intelligent User Interfaces, IUI ’17,
page 287–300, New York, NY, USA. Association for
Computing Machinery.
Brams, A. H., Jakobsen, A. L., Jendal, T. E., Lissandrini,
M., Dolog, P., and Hose, K. (2020). Mindreader: Rec-
ommendation over knowledge graph entities with ex-
plicit user ratings. In Proceedings of the 29th ACM In-
ternational Conference on Information & Knowledge
Management, page 2975–2982, New York, NY, USA.
Association for Computing Machinery.
Br
´
ezillon, P. (2012). Context in artificial intelligence: I. a
survey of the literature. COMPUTING AND INFOR-
MATICS, 18(4):321–340.
Dey, A. (2009). Explanations in context-aware systems.
pages 84–93.
Gunning, D. and Aha, D. (2019). Darpa’s explainable
artificial intelligence (xai) program. AI Magazine,
40(2):44–58.
Han, J., Pei, J., Yin, Y., and Mao, R. (2004). Min-
ing frequent patterns without candidate generation:
A frequent-pattern tree approach. Data Mining and
Knowledge Discovery, 8(1):53–87.
Harper, F. M. and Konstan, J. A. (2015). The movielens
datasets: History and context. ACM Trans. Interact.
Intell. Syst., 5(4).
Liao, Q. V., Pribic, M., Han, J., Miller, S., and Sow, D.
(2021). Question-driven design process for explain-
able AI user experiences. CoRR, abs/2104.03483.
Malandri, L., Mercorio, F., Mezzanzanica, M., and Nobani,
N. (2023). Convxai: a system for multimodal inter-
action with any black-box explainer. Cogn. Comput.,
15(2):613–644.
N
´
obrega, C. and Marinho, L. (2019). Towards explaining
recommendations through local surrogate models. In
Proceedings of the 34th ACM/SIGAPP Symposium on
Applied Computing, SAC ’19, page 1671–1678, New
York, NY, USA. Association for Computing Machin-
ery.
Peake, G. and Wang, J. (2018). Explanation mining: Post
hoc interpretability of latent factor models for rec-
ommendation systems. In Proceedings of the 24th
ACM SIGKDD International Conference on Knowl-
edge Discovery & Data Mining, KDD ’18, page
2060–2069, New York, NY, USA. Association for
Computing Machinery.
Rendle, S. (2010). Factorization machines. In 2010 IEEE
International Conference on Data Mining, pages 995–
1000.
Ribeiro, M. T., Singh, S., and Guestrin, C. (2016). ”why
should i trust you?”: Explaining the predictions of any
classifier.
Robinson, D. and Nyrup, R. (2022). Explanatory pragma-
tism: A context-sensitive framework for explainable
medical ai. Ethics and Information Technology, 24(1).
Schneider, J. and Handali, J. (2019). Personalized explana-
tion in machine learning. CoRR, abs/1901.00770.
Srinivasan, R. and Chander, A. (2021). Explanation per-
spectives from the cognitive sciences—a survey. In
Proceedings of the Twenty-Ninth International Joint
Conference on Artificial Intelligence, IJCAI’20.
ICAART 2024 - 16th International Conference on Agents and Artificial Intelligence
706