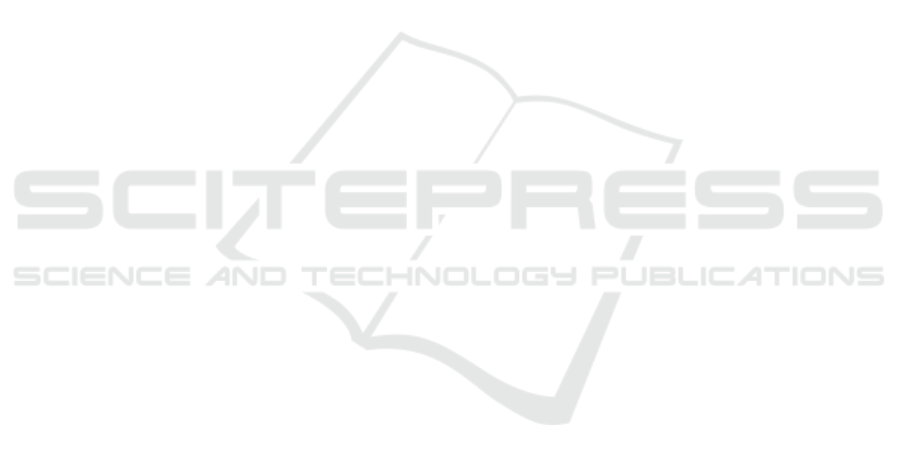
care sector.
For future research, it is recommended to investi-
gate broader healthcare datasets to validate different
hit techniques, varying in size and complexity. These
analyses should include databases with high cardi-
nality to compare supervised and unsupervised ap-
proaches, improving programming techniques in ma-
chine learning projects in healthcare.
ACKNOWLEDGEMENTS
The authors thank the National Council for Scien-
tific and Technological Development of Brazil (CNPq
- Conselho Nacional de Desenvolvimento Cient
´
ıfico
e Tecnol
´
ogico – Code: 311573/2022-3), the Pon-
tif
´
ıcia Universidade Cat
´
olica de Minas Gerais –
PUC-Minas, the Coordination for the Improvement
of Higher Education Personnel - Brazil (CAPES –
Grant PROAP 88887.842889/2023-00 – PUC/MG,
Grant PDPG 88887.708960/2022-00 – PUC/MG -
Inform
´
atica and Finance Code 001), and the Foun-
dation for Research Support of Minas Gerais State
(FAPEMIG – Code: APQ-03076-18).
REFERENCES
Association, A. P. et al. (2014). DSM-5: Manual
diagn
´
ostico e estat
´
ıstico de transtornos mentais.
Artmed Editora.
Bal
´
azs, J., Mikl
´
osi, M., Kereszt
´
eny,
´
A., Hoven, C. W., Carli,
V., Wasserman, C., Apter, A., Bobes, J., Brunner, R.,
Cosman, D., et al. (2013). Adolescent subthreshold-
depression and anxiety: Psychopathology, functional
impairment and increased suicide risk. Journal of
child psychology and psychiatry, 54(6):670–677.
Bernaras, E., Jaureguizar, J., and Garaigordobil, M. (2019).
Child and adolescent depression: A review of theo-
ries, evaluation instruments, prevention programs, and
treatments. Frontiers in psychology, 10:543.
Breskuvien
˙
e, D. and Dzemyda, G. (2023). Categori-
cal feature encoding techniques for improved classi-
fier performance when dealing with imbalanced data
of fraudulent transactions. International Journal of
Computers Communications & Control, 18(3).
Buckman, J., Roy, A., Raffel, C., and Goodfellow, I. (2018).
Thermometer encoding: One hot way to resist adver-
sarial examples. In International conference on learn-
ing representations.
Cerda, P. and Varoquaux, G. (2022). Encoding high-
cardinality string categorical variables. IEEE Transac-
tions on Knowledge and Data Engineering, 34:1164–
1176.
Chu, S.-H., Lenglet, C., Schreiner, M. W., Klimes-Dougan,
B., Cullen, K., and Parhi, K. K. (2018). Classifying
treated vs. untreated mdd adolescents from anatomical
connectivity using nonlinear svm. In 2018 40th An-
nual International Conference of the IEEE Engineer-
ing in Medicine and Biology Society (EMBC), pages
1–4.
Coutinho, M. P. L., Oliveira, M. X., Pereira, D. R., and
Santana, I. O. (2014). Indicadores psicom
´
etricos do
invent
´
ario de depress
˜
ao infantil em amostra infanto-
juvenil. Avaliac¸ao Psicologica: Interamerican Jour-
nal of Psychological Assessment, 13:269–276.
Hankin, B. L. (2006). Adolescent depression: Descrip-
tion, causes, and interventions. Epilepsy & Behavior,
8(1):102–114.
Herrman, H., Kieling, C., McGorry, P., Horton, R., Sargent,
J., and Patel, V. (2019). Reducing the global burden
of depression: a lancet–world psychiatric association
commission. The Lancet, 393(10189):e42–e43.
Kim, D., Kang, P., Kim, J., Kim, C. Y., Lee, J.-H., Suh,
S., and Lee, M.-S. (2019). Machine learning classi-
fication of first-onset drug-naive mdd using structural
mri. IEEE Access, 7:153977–153985.
Kotsiantis, S. B., Kanellopoulos, D., and Pintelas, P. E.
(2006). Data preprocessing for supervised leaning.
International journal of computer science, 1(2):111–
117.
Kovalerchuk, B. and McCoy, E. (2023). Explain-
able machine learning for categorical and mixed
data with lossless visualization. arXiv preprint
arXiv:2305.18437.
Kuhn, M. and Johnson, K. (2019). Feature Engineering
and Selection: A Practical Approach for Predictive
Models. CRC Press.
Lundberg, S. M. and Lee, S.-I. (2017). A unified approach
to interpreting model predictions. In Advances in neu-
ral information processing systems, pages 4765–4774.
PAHO (2022). Depression. https://www.paho.org/en/topics
/depression.
Pargent, F., Pfisterer, F., Thomas, J., and Bischl, B.
(2022). Regularized target encoding outperforms tra-
ditional methods in supervised machine learning with
high cardinality features. Computational Statistics,
37:2671––2692.
Roy, B. (2019). All about categorical variable encoding.
Vellido, A. (2020). The importance of interpretability and
visualization in machine learning for applications in
medicine and health care. Neural computing and ap-
plications, 32(24):18069–18083.
WHO (2021). Depression. https://www.who.int/news-roo
m/fact-sheets/detail/adolescent-mental-health.
Yu, K.-H., Beam, A. L., and Kohane, I. S. (2018). Artifi-
cial intelligence in healthcare. Nature biomedical en-
gineering, 2(10):719–731.
Assessment of the Relationship Between Attribute Coding and the Interpretability of Machine Learning Models: An Analysis in the Context
of Children and Adolescents with Depression
489