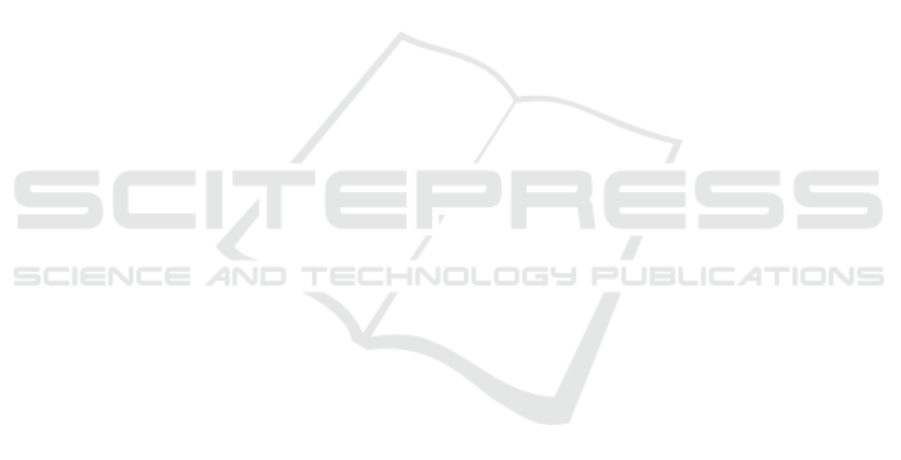
LIME, RetinexNet and our method are able to remove
the effect of the illumination. However, LIME and
RetinexNet are affected by noise and also produce ar-
tifacts, like the halo surrounding the lamp.
6 CONCLUSION
In this paper, we have proposed a low-light image en-
hancement method that estimates illumination and re-
flectance separately using variational models. In par-
ticular, we have introduced a contrast-invariant non-
local regularization term for recovering fine details
in the reflectance component. The experiments have
shown that our method obtains state-of-the-art results
and performs well in terms of noise reduction, color
recovery, geometry and texture preservation.
ACKNOWLEDGEMENTS
This work is part of the MaLiSat project TED2021-
132644B-I00, funded by MCIN/AEI/10.13039/
501100011033/ and by the European Union
NextGenerationEU/PRTR, and also of the Mo-
LaLIP project PID2021-125711OB-I00, financed by
MCIN/AEI/10.13039/501100011033/FEDER, EU.
REFERENCES
Buades, A., Lisani, J.-L., Petro, A. B., and Sbert, C.
(2020). Backlit images enhancement using global tone
mappings and image fusion. IET Image Processing,
14(2):211–219.
Chambolle, A. and Pock, T. (2011). A first-order primal-
dual algorithm for convex problems with applications
to imaging. Journal of Mathematical Imaging and Vi-
sion, 40:120–145.
Chambolle, A. and Pock, T. (2016). An introduction to
continuous optimization for imaging. Acta Numerica,
25:161–319.
Duran, J., Buades, A., Coll, B., and Sbert, C. (2014). A
nonlocal variational model for pansharpening image
fusion. SIAM Journal on Imaging Sciences, 7(2):761–
796.
Fu, X., Zeng, D., Huang, Y., Liao, Y., Ding, X., and Pais-
ley, J. (2016a). A fusion-based enhancing method
for weakly illuminated images. Signal Processing,
129:82–96.
Fu, X., Zeng, D., Huang, Y., Zhang, X., and Ding, X.
(2016b). A weighted variational model for simulta-
neous reflectance and illumination estimation. CVPR,
pages 2782–2790.
Gilboa, G. and Osher, S. (2009). Nonlocal operators with
applications to image processing. Multiscale Model-
ing & Simulation, 7(3):1005–1028.
Gu, Z., Li, F., and Lv, X.-G. (2019). A detail preserving
variational model for image retinex. Applied Mathe-
matical Modelling, 68:643–661.
Guo, X., Yu, L., and Ling, H. (2017). Lime: Low-light
image enhancement via illumination map estimation.
IEEE Transactions on Image Processing, 26(2):982–
993.
Jobson, D., Rahman, Z., and Woodell, G. (1996). Properties
and performance of a center/surround retinex. TIP,
6(3):451–462.
Jobson, D., Rahman, Z., and Woodell, G. (1997). A multi-
scale retinex for bridging the gap between color im-
ages and the human observation of scenes. IEEE
Transactions on Image Processing, 6(7):965–976.
Kimmel, R., Elad, M., Shaked, D., Keshet, R., and Sobel,
I. (2001). A variational framework for retinex. Int. J.
Comput. Vis., 52(1):7–23.
Land, E. H. and McCann, J. J. (1971). Lightness and retinex
theory. Journal of the Optical Society of America,
61(1):1–11.
Li, M., Liu, J., Yang, W., Sun, X., and Guo, Z. (2018).
Structure-revealing low-light image enhancement via
robust retinex model. IEEE Transactions on Image
Processing, pages 2828–2841.
Lv, F., Yu, L., and Lu, F. (2021). Attention guided low-
light image enhancement with a large scale low-light
simulation dataset. International Journal of Computer
Vision, pages 2175—-2193.
Ma, W. and Osher, S. (2012). A tv bregman iterative model
of retinex theory. Inverse Problems and Imaging,
6(4):697–708.
Morel, J. M., Petro, A. B., and Sbert, C. (2010). A pde
formalization of retinex theory. IEEE Transactions on
Image Processing, 19(11):2825–2837.
Ng, M. K. and Wang, W. (2011). A total variation model
for retinex. SIAM J. Imag. Sci., 4(1):345–365.
Paul, A., Bhattacharya, P., and Maity, S. P. (2022). His-
togram modification in adaptive bi-histogram equal-
ization for contrast enhancement on digital images.
Optik, 259:168899.
Petro, A. B., Sbert, C., and Morel, J. M. (2014). Multiscale
retinex. Image Processing On Line, pages 71–88.
Rudin, L. I., Osher, S., and Fatemi, E. (1992). Nonlinear to-
tal variation based noise removal algorithms. Physica
D: Nonlinear Phenomena, 60(1):259–268.
Thepade, S. D., Ople, M., Mahindra, V., Kulye, V., and
Jamdar, S. (2021). Low light image contrast enhance-
ment using blending of histogram equalization based
methods bbhe and bpheme. In 2021 International
Conference on Disruptive Technologies for Multi-
Disciplinary Research and Applications (CENTCON),
volume 1, pages 259–264.
Wang, W., Wu, X., and snd Z. Guo, X. Y. (2020). An
experiment-based review of low-light image enhance-
ment methods. IEEE Access, 8:87884–87917.
Wei, C., Wang, W., Yang, W., and Liu, J. (2018). Deep
retinex decomposition for low-light enhancement.
BMVC.
Ying, Z., Li, G., and Gao, W. (2017). A bio-inspired multi-
exposure fusion framework for low-light image en-
hancement.
Combining Total Variation and Nonlocal Variational Models for Low-Light Image Enhancement
515