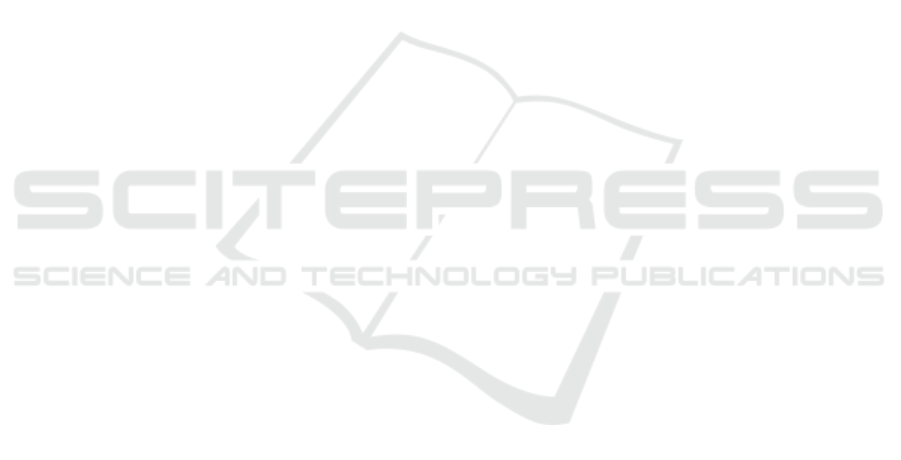
federation of clinical neurophysiology. Clinical Neu-
rophysiology, 132(5):1173–1184.
Bidwell, J., Khuwatsamrit, T., Askew, B., Ehrenberg, J. A.,
and Helmers, S. (2015). Seizure reporting technolo-
gies for epilepsy treatment: A review of clinical infor-
mation needs and supporting technologies. Seizure,
32:109–117.
Blagus, R. and Lusa, L. (2013). Smote for high-dimensional
class-imbalanced data. BMC bioinformatics, 14:1–16.
B
¨
oring, Y. (2021). Implementierung eines convolutional
neural networks zur detektion motorischer epileptis-
cher anf
¨
alle mittels biosignalen eines in-ohr-sensors.
Unpublished master’s project.
Fraunhofer (2023). Mobile, smart neurosensor system for
the detection and documentation of epileptic seizures
in everyday life.
Hasibi, R., Shokri, M., and Dehghan, M. (2019). Aug-
mentation scheme for dealing with imbalanced net-
work traffic classification using deep learning. arXiv
preprint arXiv:1901.00204.
Henze, J., Houta, S., Surges, R., Kreuzer, J., and Bis-
gin, P. (2021). Multimodal detection of tonic–clonic
seizures based on 3d acceleration and heart rate data
from an in-ear sensor. In Del Bimbo, A., Cucchiara,
R., Sclaroff, S., Farinella, G. M., Mei, T., Bertini,
M., Escalante, H. J., and Vezzani, R., editors, Pat-
tern Recognition. ICPR International Workshops and
Challenges, pages 490–502, Cham. Springer Interna-
tional Publishing.
Houta, S., Bisgin, P., and Dulich, P. (2019). Machine
learning methods for detection of epileptic seizures
with long-term wearable devices. In Eleventh Interna-
tional Conference on eHealth, Telemedicine, and So-
cial Medicine.
Iglesias, G., Talavera, E., Gonz
´
alez-Prieto,
´
A., Mozo,
A., and G
´
omez-Canaval, S. (2023). Data augmen-
tation techniques in time series domain: a survey
and taxonomy. Neural Computing and Applications,
35(14):10123–10145.
Ismail Fawaz, H., Forestier, G., Weber, J., Idoumghar, L.,
and Muller, P.-A. (2021). Deep learning for time series
classification: a review. Data Mining and Knowledge
Discovery, 33(4):917–963.
Iwana, B. K. and Uchida, S. (2021). An empirical survey of
data augmentation for time series classification with
neural networks. Plos one, 16(7):e0254841.
Kingma, D. P. and Ba, J. (2014). Adam: A
method for stochastic optimization. arXiv preprint
arXiv:1412.6980.
Lashgari, E., Liang, D., and Maoz, U. (2020). Data aug-
mentation for deep-learning-based electroencephalog-
raphy. Journal of Neuroscience Methods, 346:108885.
McGeehan, B. (2018). Supporting students with epilepsy in
the school setting. Communique, 47(3):8–10.
Meisel, C., El Atrache, R., Jackson, M., Schubach, S.,
Ufongene, C., and Loddenkemper, T. (2020). Ma-
chine learning from wristband sensor data for wear-
able, noninvasive seizure forecasting. Epilepsia,
61(12):2653–2666.
Ramgopal, S., Thome-Souza, S., Jackson, M., Kadish,
N. E., S
´
anchez Fern
´
andez, I., Klehm, J., Bosl, W.,
Reinsberger, C., Schachter, S., and Loddenkemper,
T. (2014). Seizure detection, seizure prediction, and
closed-loop warning sstems in epilepsy. Epilepsy and
Behavior, 37:291–307.
Sazgar, M. and Young, M. (2019). Absolute Epilepsy
and EEG Rotation Review: Essentials for Trainees.
Springer International Publishing.
Schulze-Bonhage, A., Sales, F., Wagner, K., Teotonio, R.,
Carius, A., Schelle, A., and Ihle, M. (2010). Views of
patients with epilepsy on seizure prediction devices.
Epilepsy & behavior, 18(4):388–396.
Shoeb, A. H. and Guttag, J. V. (2010). Application of ma-
chine learning to epileptic seizure detection. In Pro-
ceedings of the 27th international conference on ma-
chine learning (ICML-10), pages 975–982.
Shoeibi, A., Khodatars, M., Ghassemi, N., Jafari, M.,
Moridian, P., Alizadehsani, R., Panahiazar, M.,
Khozeimeh, F., Zare, A., Hosseini-Nejad, H., Khos-
ravi, A., Atiya, A. F., Aminshahidi, D., Hussain,
S., Rouhani, M., Nahavandi, S., and Acharya, U. R.
(2021). Epileptic seizures detection using deep
learning techniques: A review. International Jour-
nal of Environmental Research and Public Health,
18(11):5780.
Shorten, C. and Khoshgoftaar, T. M. (2019). A survey on
image data augmentation for deep learning. Journal
of big data, 6(1):1–48.
Siddiqui, M. K., Huang, X., Morales-Menendez, R., Hus-
sain, N., and Khatoon, K. (2020). Machine learning
based novel cost-sensitive seizure detection classifier
for imbalanced eeg data sets. International Journal
on Interactive Design and Manufacturing (IJIDeM),
14:1491–1509.
Sun, Y., Wong, A. K., and Kamel, M. S. (2009). Classifica-
tion of imbalanced data: A review. International jour-
nal of pattern recognition and artificial intelligence,
23(04):687–719.
Um, T. T., Pfister, F. M., Pichler, D., Endo, S., Lang,
M., Hirche, S., Fietzek, U., and Kuli
´
c, D. (2017).
Data augmentation of wearable sensor data for parkin-
son’s disease monitoring using convolutional neural
networks. In Proceedings of the 19th ACM inter-
national conference on multimodal interaction, pages
216–220.
Wen, Q., Sun, L., Yang, F., Song, X., Gao, J., Wang, X.,
and Xu, H. (2021). Time series data augmentation for
deep learning: A survey. In Proceedings of the Thirti-
eth International Joint Conference on Artificial Intel-
ligence. International Joint Conferences on Artificial
Intelligence Organization.
Comparison of Different Data Augmentation Techniques for Improving Epileptic Seizure Detection Based on 3D Acceleration, Heart Rate
and Temperature Data
153