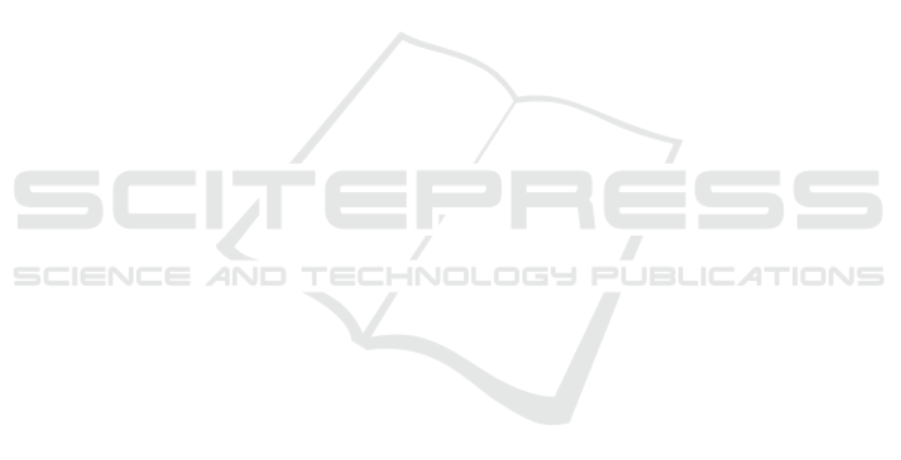
tional Conference on Parallel and Distributed Com-
puting: Applications and Technologies, pages 45–56.
Springer.
Bonettini, N., Cannas, E. D., Mandelli, S., Bondi, L.,
Bestagini, P., and Tubaro, S. (2021). Video face ma-
nipulation detection through ensemble of cnns. In
2020 25th international conference on pattern recog-
nition (ICPR), pages 5012–5019. IEEE.
Brown, T. B., Mann, B., Ryder, N., Subbiah, M., Kaplan, J.,
Dhariwal, P., Neelakantan, A., Shyam, P., Sastry, G.,
Askell, A., Agarwal, S., Herbert-Voss, A., Krueger,
G., Henighan, T., Child, R., Ramesh, A., Ziegler,
D. M., Wu, J., Winter, C., Hesse, C., Chen, M., Sigler,
E., Litwin, M., Gray, S., Chess, B., Clark, J., Berner,
C., McCandlish, S., Radford, A., Sutskever, I., and
Amodei, D. (2020). Language models are few-shot
learners.
Chen, P., Liu, J., Liang, T., Zhou, G., Gao, H., Dai, J.,
and Han, J. (2020). Fsspotter: Spotting face-swapped
video by spatial and temporal clues. In 2020 IEEE
International Conference on Multimedia and Expo
(ICME), pages 1–6.
Chollet, F. (2017). Xception: Deep learning with depthwise
separable convolutions. In Proceedings of the IEEE
conference on computer vision and pattern recogni-
tion, pages 1251–1258.
Ciftci, U. A., Demir, I., and Yin, L. (2020). Fakecatcher:
Detection of synthetic portrait videos using biological
signals. IEEE transactions on pattern analysis and
machine intelligence.
DeepfakeVFX.com (2023). DeepFaceLab - Deep-
fakeVFX.com — deepfakevfx.com. https://www.
deepfakevfx.com/downloads/deepfacelab/. [Accessed
21-Jun-2023].
Deng, Y., Yang, J., Chen, D., Wen, F., and Tong, X. (2020).
Disentangled and controllable face image generation
via 3d imitative-contrastive learning.
Dhariwal, P. and Nichol, A. (2021). Diffusion models beat
gans on image synthesis.
Elhassan, A., Al-Fawa’reh, M., Jafar, M. T., Ababneh, M.,
and Jafar, S. T. (2022). Dft-mf: Enhanced deepfake
detection using mouth movement and transfer learn-
ing. SoftwareX, 19:101115.
FaceApp (2023). FaceApp: Face Editor — faceapp.com.
https://www.faceapp.com/. [Accessed 21-Jun-2023].
Gomes, T. L., Martins, R., Ferreira, J., and Nascimento,
E. R. (2020). Do as i do: Transferring human mo-
tion and appearance between monocular videos with
spatial and temporal constraints.
Haliassos, A., Vougioukas, K., Petridis, S., and Pantic, M.
(2021). Lips don’t lie: A generalisable and robust ap-
proach to face forgery detection. In Proceedings of the
IEEE/CVF conference on computer vision and pattern
recognition, pages 5039–5049.
Hubens, N., Mancas, M., Gosselin, B., Preda, M., and Za-
haria, T. (2021). Fake-buster: A lightweight solution
for deepfake detection. In Applications of Digital Im-
age Processing XLIV, volume 11842, pages 146–154.
SPIE.
Ismail, A., Elpeltagy, M., S. Zaki, M., and Eldahshan,
K. (2021). A new deep learning-based methodology
for video deepfake detection using xgboost. Sensors,
21(16):5413.
Jolicoeur-Martineau, A., Pich
´
e-Taillefer, R., des Combes,
R. T., and Mitliagkas, I. (2020). Adversarial score
matching and improved sampling for image genera-
tion. CoRR, abs/2009.05475.
Karras, T., Laine, S., and Aila, T. (2019). A style-based
generator architecture for generative adversarial net-
works.
Karras, T., Laine, S., Aittala, M., Hellsten, J., Lehtinen, J.,
and Aila, T. (2020). Analyzing and improving the im-
age quality of stylegan.
Lattas, A., Moschoglou, S., Gecer, B., Ploumpis, S., Tri-
antafyllou, V., Ghosh, A., and Zafeiriou, S. (2020).
Avatarme: Realistically renderable 3d facial recon-
struction ”in-the-wild”.
Nirkin, Y., Keller, Y., and Hassner, T. (2019). Fsgan: Sub-
ject agnostic face swapping and reenactment.
Radford, A., Kim, J. W., Hallacy, C., Ramesh, A., Goh, G.,
Agarwal, S., Sastry, G., Askell, A., Mishkin, P., Clark,
J., et al. (2021). Learning transferable visual models
from natural language supervision. In International
conference on machine learning, pages 8748–8763.
PMLR.
Ramesh, A., Dhariwal, P., Nichol, A., Chu, C., and Chen,
M. (2022). Hierarchical text-conditional image gener-
ation with clip latents.
Rana, M. S., Nobi, M. N., Murali, B., and Sung, A. H.
(2022). Deepfake detection: A systematic literature
review. IEEE Access.
Ranjan, P., Patil, S., and Kazi, F. (2020). Improved general-
izability of deep-fakes detection using transfer learn-
ing based cnn framework. In 2020 3rd international
conference on information and computer technologies
(ICICT), pages 86–90. IEEE.
Rombach, R., Blattmann, A., Lorenz, D., Esser, P., and Om-
mer, B. (2022). High-resolution image synthesis with
latent diffusion models.
Ronneberger, O., Fischer, P., and Brox, T. (2015). U-
net: Convolutional networks for biomedical image
segmentation. In Medical Image Computing and
Computer-Assisted Intervention–MICCAI 2015: 18th
International Conference, Munich, Germany, October
5-9, 2015, Proceedings, Part III 18, pages 234–241.
Springer.
Rossler, A., Cozzolino, D., Verdoliva, L., Riess, C., Thies,
J., and Nießner, M. (2019). Faceforensics++: Learn-
ing to detect manipulated facial images. In Proceed-
ings of the IEEE/CVF international conference on
computer vision, pages 1–11.
Tariq, S., Lee, S., Kim, H., Shin, Y., and Woo, S. S. (2018).
Detecting both machine and human created fake face
images in the wild. In Proceedings of the 2nd interna-
tional workshop on multimedia privacy and security,
pages 81–87.
Thies, J., Zollh
¨
ofer, M., and Nießner, M. (2019). Deferred
neural rendering: Image synthesis using neural tex-
Diffusion-Inspired Dynamic Models for Enhanced Fake Face Detection
537