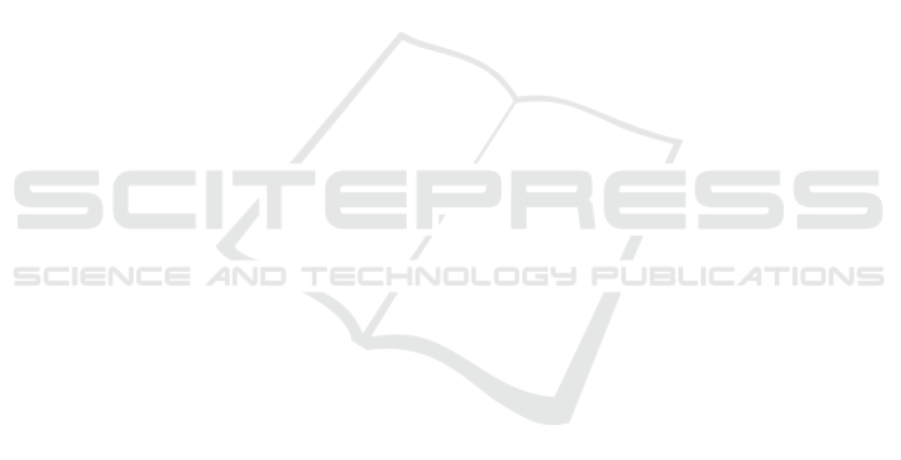
5 CONCLUSIONS
This paper presents a method to perform temporal
modeling effectively and efficiently for video recog-
nition tasks. This architecture, AR-VPT, is an exten-
sion of the original VPT architecture and adapts the
prompt-tuning technique in visual space to perform
temporal feature learning. We demonstrate that our
model is able to effectively learn long-range depen-
dencies in the spatiotemporal dimension via the eval-
uation on both coarse and fine-grained video datasets.
This method shows how effective a simple prompt-
ing mechanism can be when incorporating informa-
tion sharing across frames auto-regressively.
REFERENCES
Arnab, A., Dehghani, M., Heigold, G., Sun, C., Lu
ˇ
ci
´
c, M.,
and Schmid, C. (2021). Vivit: A video vision trans-
former. In ICCV.
Bahng, H., Jahanian, A., Sankaranarayanan, S., and Isola,
P. (2022). Visual prompting: Modifying pixel space
to adapt pre-trained models. arXiv:2203.17274.
Bertasius, G., Wang, H., and Torresani, L. (2021). Is space-
time attention all you need for video understanding?
In ICML.
Brown, T., Mann, B., Ryder, N., Subbiah, M., Kaplan, J. D.,
Dhariwal, P., Neelakantan, A., Shyam, P., Sastry, G.,
Askell, A., et al. (2020). Language models are few-
shot learners. Advances in neural information pro-
cessing systems, 33:1877–1901.
Carreira, J. and Zisserman, A. (2017). Quo vadis, action
recognition? a new model and the kinetics dataset. In
CVPR.
Doll
´
ar, P., Rabaud, V., Cottrell, G., and Belongie, S. (2005).
Behavior recognition via sparse spatio-temporal fea-
tures. In 2005 IEEE international workshop on visual
surveillance and performance evaluation of tracking
and surveillance, pages 65–72. IEEE.
Dosovitskiy, A., Beyer, L., Kolesnikov, A., Weissenborn,
D., Zhai, X., Unterthiner, T., Dehghani, M., Minderer,
M., Heigold, G., Gelly, S., Uszkoreit, J., and Houlsby,
N. (2021). An image is worth 16x16 words: Trans-
formers for image recognition at scale. In ICLR.
Duan, H., Zhao, Y., Xiong, Y., Liu, W., and Lin, D. (2020).
Omni-sourced webly-supervised learning for video
recognition. In ECCV.
Fan, H., Xiong, B., Mangalam, K., Li, Y., Yan, Z., Malik,
J., and Feichtenhofer, C. (2021). Multiscale vision
transformers. In ICCV.
Feichtenhofer, C. (2020). X3d: Expanding architectures for
efficient video recognition. In CVPR.
Feichtenhofer, C., Fan, H., Malik, J., and He, K. (2019).
Slowfast networks for video recognition. In ICCV.
Feichtenhofer, C., Pinz, A., and Wildes, R. (2016). Spa-
tiotemporal residual networks for video action recog-
nition. In NeurIPS.
Gao, T., Fisch, A., and Chen, D. (2020). Making pre-
trained language models better few-shot learners.
arXiv:2012.15723.
Goyal, R., Ebrahimi Kahou, S., Michalski, V., Materzyn-
ska, J., Westphal, S., Kim, H., Haenel, V., Fruend, I.,
Yianilos, P., Mueller-Freitag, M., et al. (2017). The”
something something” video database for learning and
evaluating visual common sense. In ICCV.
He, K., Zhang, X., Ren, S., and Sun, J. (2016). Deep resid-
ual learning for image recognition. In CVPR.
Jia, M., Tang, L., Chen, B.-C., Cardie, C., Belongie, S.,
Hariharan, B., and Lim, S.-N. (2022a). Visual prompt
tuning. In ECCV.
Jia, M., Tang, L., Chen, B.-C., Cardie, C., Belongie, S.,
Hariharan, B., and Lim, S.-N. (2022b). Visual prompt
tuning. arXiv:2203.12119.
Jiang, Z., Xu, F. F., Araki, J., and Neubig, G. (2020). How
can we know what language models know? Trans-
actions of the Association for Computational Linguis-
tics, 8:423–438.
Kay, W., Carreira, J., Simonyan, K., Zhang, B., Hillier, C.,
Vijayanarasimhan, S., Viola, F., Green, T., Back, T.,
Natsev, P., et al. (2017). The kinetics human action
video dataset. In arXiv:1705.06950.
Khattak, M. U., Rasheed, H., Maaz, M., Khan, S., and
Khan, F. S. (2023). Maple: Multi-modal prompt learn-
ing. In The IEEE/CVF Conference on Computer Vi-
sion and Pattern Recognition.
Klaser, A., Marszałek, M., and Schmid, C. (2008). A spatio-
temporal descriptor based on 3d-gradients. In BMVC.
Kondratyuk, D., Yuan, L., Li, Y., Zhang, L., Tan, M.,
Brown, M., and Gong, B. (2021). Movinets: Mo-
bile video networks for efficient video recognition. In
CVPR.
Krizhevsky, A., Sutskever, I., and Hinton, G. E. (2012). Im-
agenet classification with deep convolutional neural
networks. In NeurIPS.
Lester, B., Al-Rfou, R., and Constant, N. (2021). The
power of scale for parameter-efficient prompt tuning.
arXiv:2104.08691.
Li, K., Wang, Y., Gao, P., Song, G., Liu, Y., Li, H., and
Qiao, Y. (2022a). Uniformer: Unified transformer
for efficient spatiotemporal representation learning. In
ICLR.
Li, X. L. and Liang, P. (2021). Prefix-tuning: Op-
timizing continuous prompts for generation.
arXiv:2101.00190.
Li, Y., Ji, B., Shi, X., Zhang, J., Kang, B., and Wang, L.
(2020). Tea: Temporal excitation and aggregation for
action recognition. In CVPR.
Li, Y., Li, Y., and Vasconcelos, N. (2018). Resound: To-
wards action recognition without representation bias.
In ECCV.
Li, Y., Wu, C.-Y., Fan, H., Mangalam, K., Xiong, B., Malik,
J., and Feichtenhofer, C. (2022b). Mvitv2: Improved
multiscale vision transformers for classification and
detection. In CVPR.
Lin, J., Gan, C., and Han, S. (2019). Tsm: Temporal shift
module for efficient video understanding. In ICCV.
AR-VPT: Simple Auto-Regressive Prompts for Adapting Frozen ViTs to Videos
637