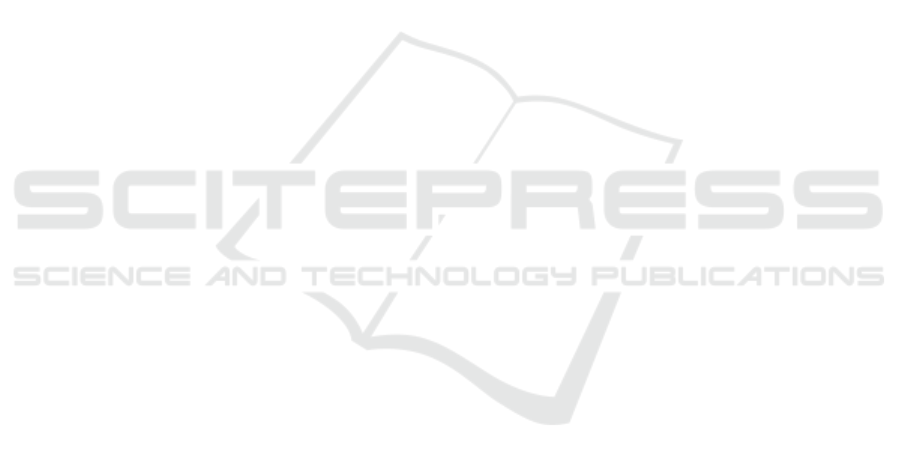
resolution. In European conference on computer vi-
sion, pages 184–199. Springer.
Eismann, M. T. and Hardie, R. C. (2005). Hyperspectral
resolution enhancement using high-resolution multi-
spectral imagery with arbitrary response functions.
IEEE Transactions on Geoscience and Remote Sens-
ing, 43(3):455–465.
Gharbi, M., Chaurasia, G., Paris, S., and Durand, F. (2016).
Deep joint demosaicking and denoising. ACM Trans-
actions on Graphics (TOG), 35(6):1–12.
G
¨
ob, S., G
¨
otz, T. I., and Wittenberg, T. (2021). Multispec-
tral single chip reconstruction using dnns with appli-
cation to open neurosurgery. Current Directions in
Biomedical Engineering, 7(2):37–40.
Habtegebrial, T. A., Reis, G., and Stricker, D. (2019). Deep
convolutional networks for snapshot hypercpectral de-
mosaicking. In 2019 10th Workshop on Hyperspectral
Imaging and Signal Processing: Evolution in Remote
Sensing (WHISPERS), pages 1–5. IEEE.
Hershey, J. and Zhang, Z. (2008). Multispectral digital
camera employing both visible light and non-visible
light sensing on a single image sensor. US Patent
7,460,160.
Hyttinen, J., F
¨
alt, P., J
¨
asberg, H., Kullaa, A., and Hauta-
Kasari, M. (2020). Oral and dental spectral image
database—odsi-db. Applied Sciences, 10(20):7246.
Jung, A., Kardev
´
an, P., and T
˝
okei, L. (2006). Hyperspectral
technology in vegetation analysis. Progress in Agri-
cultural Engineering Sciences, 2(1):95–117.
Khan, H. A., Mihoubi, S., Mathon, B., Thomas, J.-B., and
Hardeberg, J. Y. (2018). Hytexila: High resolution
visible and near infrared hyperspectral texture images.
Sensors, 18(7):2045.
Li, Q., Wang, Q., and Li, X. (2020). Mixed 2d/3d con-
volutional network for hyperspectral image super-
resolution. Remote Sensing, 12(10):1660.
Li, Y., Zhang, L., Dingl, C., Wei, W., and Zhang, Y.
(2018). Single hyperspectral image super-resolution
with grouped deep recursive residual network. In 2018
IEEE Fourth International Conference on Multimedia
Big Data (BigMM), pages 1–4. IEEE.
Lim, B., Son, S., Kim, H., Nah, S., and Mu Lee, K. (2017).
Enhanced deep residual networks for single image
super-resolution. In Proceedings of the IEEE con-
ference on computer vision and pattern recognition
workshops, pages 136–144.
Lu, G. and Fei, B. (2014). Medical hyperspectral imaging:
a review. Journal of Biomedical Optics, 19(1):10901.
Malvar, H. S., He, L.-w., and Cutler, R. (2004). High-
quality linear interpolation for demosaicing of bayer-
patterned color images. In 2004 IEEE International
Conference on Acoustics, Speech, and Signal Process-
ing, volume 3, pages iii–485. IEEE.
Mei, S., Yuan, X., Ji, J., Zhang, Y., Wan, S., and Du, Q.
(2017). Hyperspectral image spatial super-resolution
via 3d full convolutional neural network. Remote
Sensing, 9(11):1139.
Mihoubi, S., Losson, O., Mathon, B., and Macaire, L.
(2015). Multispectral demosaicing using intensity-
based spectral correlation. In International Confer-
ence on Image Processing Theory, Tools and Applica-
tions (IPTA), volume 5, pages 461–466. IEEE.
Mirhashemi, A. (2019). Configuration and registration of
multi-camera spectral image database of icon paint-
ings. Computation, 7(3):47.
Moghadam, P., Ward, D., Goan, E., Jayawardena, S., Sikka,
P., and Hernandez, E. (2017). Plant disease detection
using hyperspectral imaging. In 2017 International
Conference on Digital Image Computing: Techniques
and Applications (DICTA), pages 1–8. IEEE.
Monno, Y., Kikuchi, S., Tanaka, M., and Okutomi, M.
(2015). A practical one-shot multispectral imaging
system using a single image sensor. IEEE Transac-
tions on Image Processing, 24(10):3048–3059.
Monno, Y., Teranaka, H., Yoshizaki, K., Tanaka, M., and
Okutomi, M. (2018). Single-sensor rgb-nir imaging:
High-quality system design and prototype implemen-
tation. IEEE Sensors Journal, 19(2):497–507.
M
¨
uhle, R., Markgraf, W., Hilsmann, A., Malberg, H., Eis-
ert, P., and Wisotzky, E. L. (2021). Comparison
of different spectral cameras for image-guided or-
gan transplantation. Journal of Biomedical Optics,
26(7):076007.
Niu, B., Wen, W., Ren, W., Zhang, X., Yang, L., Wang,
S., Zhang, K., Cao, X., and Shen, H. (2020). Sin-
gle image super-resolution via a holistic attention net-
work. In Computer Vision–ECCV 2020: 16th Euro-
pean Conference, Glasgow, UK, August 23–28, 2020,
Proceedings, Part XII 16, pages 191–207. Springer.
Ronneberger, O., Fischer, P., and Brox, T. (2015). U-
net: Convolutional networks for biomedical image
segmentation. In Medical Image Computing and
Computer-Assisted Intervention–MICCAI 2015: 18th
International Conference, Munich, Germany, October
5-9, 2015, Proceedings, Part III 18, pages 234–241.
Springer.
Shafri, H. Z., Taherzadeh, E., Mansor, S., and Ashurov, R.
(2012). Hyperspectral remote sensing of urban areas:
an overview of techniques and applications. Research
Journal of Applied Sciences, Engineering and Tech-
nology, 4(11):1557–1565.
Shi, Z., Chen, C., Xiong, Z., Liu, D., and Wu, F. (2018).
Hscnn+: Advanced cnn-based hyperspectral recovery
from rgb images. In Proceedings of the IEEE Con-
ference on Computer Vision and Pattern Recognition
Workshops, pages 939–947.
Shinoda, K., Yoshiba, S., and Hasegawa, M. (2018). Deep
demosaicking for multispectral filter arrays. arXiv
preprint arXiv:1808.08021.
Song, B., Ma, S., He, F., and Sun, W. (2022). Hyperspec-
tral reconstruction from rgb images based on res2-unet
deep learning network. Opt Precis Eng, 30(13):1606.
Wang, Y., Cao, R., Guan, Y., Liu, T., and Yu, Z. (2021).
A deep survey in the applications of demosaick-
ing. In 2021 3rd International Academic Exchange
Conference on Science and Technology Innovation
(IAECST), pages 596–602. IEEE.
Wang, Y.-Q. (2014). A multilayer neural network for image
demosaicking. In 2014 IEEE International Confer-
Efficient and Accurate Hyperspectral Image Demosaicing with Neural Network Architectures
549