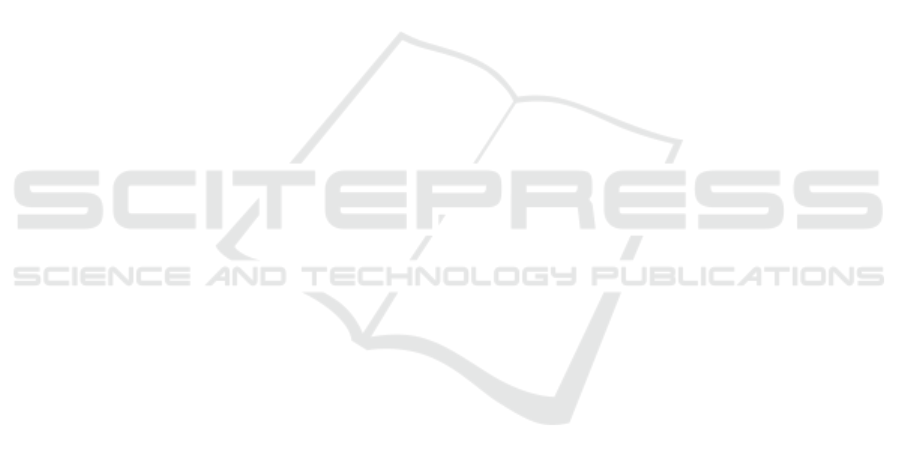
pose’ joints have an effect.
We opted for a 3D joint representation of the data
which gives freedom to the model to minimize the dis-
tance between ground truth and prediction, but a case
can be made to use bone representation in the form of
rotation vectors. This way, the distance between spe-
cific joints is always the same to achieve more consis-
tent motion.
In (Billast et al., 2023), they show that it is pos-
sible to do motion prediction on just two joints, i.e.
the hands. This fits closely with the VR application
as we have the coordinates of the controllers at all
times which would mean that the extra depth sensor
becomes obsolete. Analysing physical ergonomics on
two joints is not feasible but recent VR setups try to
estimate the full body poses based on the headset and
controllers (Jiang et al., 2022).
ACKNOWLEDGEMENTS
This research received funding from the Flemish Gov-
ernment under the “Onderzoeksprogramma Artificile
Intelligentie (AI) Vlaanderen” programme.
REFERENCES
Barquero, G., Escalera, S., and Palmero, C. (2023). Belfu-
sion: Latent diffusion for behavior-driven human mo-
tion prediction.
Billast, M., Mets, K., De Schepper, T., Oramas M, J., and
Latr
´
e, S. (2023). Human motion prediction on the
ikea-asm dataset. pages 906–914.
Chiu, H.-k., Adeli, E., Wang, B., Huang, D.-A., and
Niebles, J. C. (2019). Action-agnostic human pose
forecasting. In 2019 IEEE Winter Conference on Ap-
plications of Computer Vision (WACV), pages 1423–
1432. IEEE.
Cui, Q., Sun, H., and Yang, F. (2020). Learning dynamic
relationships for 3d human motion prediction. In Pro-
ceedings of the IEEE/CVF Conference on Computer
Vision and Pattern Recognition (CVPR).
De Bruyne, J., Joundi, J., Morton, J., Zheleva, A., Van Kets,
N., Van Wallendael, G., Talsma, D., Saldien, J.,
De Marez, L., Durnez, W., et al. (2023). I spy with
my ai: The effects of ai-based visual cueing on hu-
man operators’ performance and cognitive load in cctv
control rooms. International Journal of Industrial Er-
gonomics, 95:103444.
Guan, W., Song, X., Wang, K., Wen, H., Ni, H., Wang, Y.,
and Chang, X. (2023). Egocentric early action pre-
diction via multimodal transformer-based dual action
prediction. IEEE Transactions on Circuits and Sys-
tems for Video Technology, pages 1–1.
Guo, W., Du, Y., Shen, X., Lepetit, V., Alameda-Pineda, X.,
and Moreno-Noguer, F. (2023). Back to mlp: A sim-
ple baseline for human motion prediction. In Proceed-
ings of the IEEE/CVF Winter Conference on Applica-
tions of Computer Vision (WACV), pages 4809–4819.
Hignett, S. and McAtamney, L. (2000). Rapid entire body
assessment (reba). Applied ergonomics, 31(2):201–
205.
Hu, J., Fan, Z., Liao, J., and Liu, L. (2019). Predict-
ing long-term skeletal motions by a spatio-temporal
hierarchical recurrent network. arXiv preprint
arXiv:1911.02404.
Ionescu, C., Papava, D., Olaru, V., and Sminchisescu, C.
(2014). Human3.6m: Large scale datasets and pre-
dictive methods for 3d human sensing in natural envi-
ronments. IEEE Transactions on Pattern Analysis and
Machine Intelligence, 36(7):1325–1339.
Jaffar, N., Abdul-Tharim, A., Mohd-Kamar, I., and Lop, N.
(2011). A literature review of ergonomics risk fac-
tors in construction industry. Procedia engineering,
20:89–97.
Jiang, J., Streli, P., Qiu, H., Fender, A., Laich, L., Snape,
P., and Holz, C. (2022). Avatarposer: Articulated
full-body pose tracking from sparse motion sensing.
In Avidan, S., Brostow, G., Ciss
´
e, M., Farinella,
G. M., and Hassner, T., editors, Computer Vision –
ECCV 2022, pages 443–460, Cham. Springer Nature
Switzerland.
Joundi, J., Bombeke, K., Saldien, J., Durnez, W.,
De Bruyne, J., Vanroelen, C., and Zheleva, A. (2022).
ExperienceDNA : a framework to conduct and analyse
user tests in VR. In Faculty of Engineering and Ar-
chitecture Research Symposium 2022 (FEARS 2022),
Abstracts, page 1.
Kilis, N., Papaioannidis, C., Mademlis, I., and Pitas, I.
(2022). An efficient framework for human action
recognition based on graph convolutional networks.
In 2022 IEEE International Conference on Image Pro-
cessing (ICIP), pages 1441–1445. IEEE.
Lee, M.-L., Liu, W., Behdad, S., Liang, X., and Zheng, M.
(2022). Robot-assisted disassembly sequence plan-
ning with real-time human motion prediction. IEEE
Transactions on Systems, Man, and Cybernetics: Sys-
tems, 53(1):438–450.
Lyu, K., Chen, H., Liu, Z., Zhang, B., and Wang, R. (2022).
3d human motion prediction: A survey. Neurocom-
puting, 489:345–365.
Mahmood, N., Ghorbani, N., Troje, N. F., Pons-Moll, G.,
and Black, M. J. (2019). AMASS: Archive of motion
capture as surface shapes. In International Conference
on Computer Vision, pages 5442–5451.
Martinez, J., Black, M. J., and Romero, J. (2017). On
human motion prediction using recurrent neural net-
works. In Proceedings of the IEEE Conference on
Computer Vision and Pattern Recognition (CVPR).
Mart
´
ınez-Gonz
´
alez, A., Villamizar, M., and Odobez, J.-M.
(2021). Pose transformers (potr): Human motion pre-
diction with non-autoregressive transformers. In Pro-
ceedings of the IEEE/CVF International Conference
on Computer Vision, pages 2276–2284.
Micheletti Cremasco, M., Giustetto, A., Caffaro, F., Colan-
toni, A., Cavallo, E., and Grigolato, S. (2019). Risk
Physical Ergonomics Anticipation with Human Motion Prediction
547