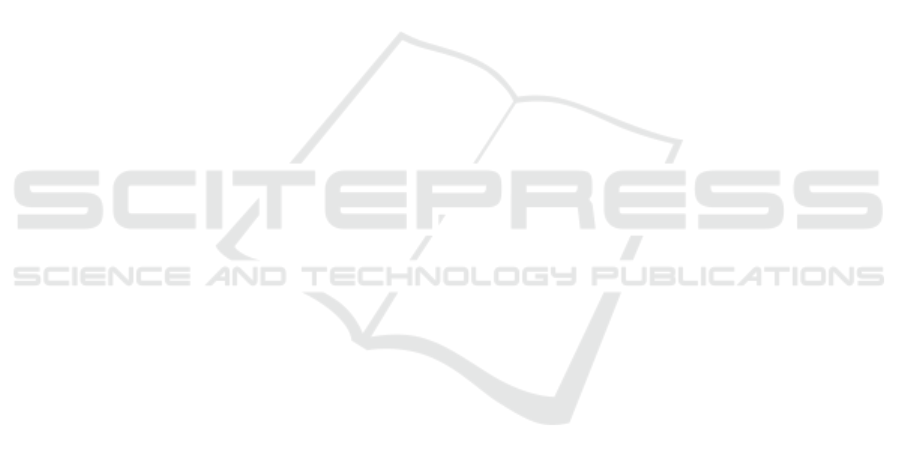
Luo, L., Xu, D., Chen, H., Wong, T.-T., and Heng, P.-
A. (2022). Pseudo Bias-Balanced Learning for Debi-
ased Chest X-Ray Classification. Lecture Notes in Com-
puter Science (including subseries Lecture Notes in Arti-
ficial Intelligence and Lecture Notes in Bioinformatics),
13438 LNCS:621 – 631. ISBN: 978-303116451-4 Pub-
lisher: Springer Science and Business Media Deutsch-
land GmbH Type: Conference paper.
Meng, C., Trinh, L., Xu, N., Enouen, J., and Liu, Y. (2022).
Interpretability and fairness evaluation of deep learn-
ing models on MIMIC-IV dataset. Scientific reports,
12(1):7166. Place: England.
Monlezun, D. J., Sinyavskiy, O., Peters, N., Steigner, L.,
Aksamit, T., Girault, M. I., Garcia, A., Gallagher, C.,
and Iliescu, C. (2022). Artificial Intelligence-Augmented
Propensity Score, Cost Effectiveness and Computational
Ethical Analysis of Cardiac Arrest and Active Cancer
with Novel Mortality Predictive Score. Medicina (Kau-
nas, Lithuania), 58(8). Place: Switzerland.
Morley, J., Machado, C. C., Burr, C., Cowls, J., Joshi, I.,
Taddeo, M., and Floridi, L. (2020). The ethics of ai
in health care: A mapping review. Social Science &
Medicine, 260:113172.
Obermeyer, Z., Powers, B., Vogeli, C., and Mullainathan,
S. (2019). Dissecting racial bias in an algorithm
used to manage the health of populations. Science,
366(6464):447–453.
Paviglianiti, A. and Pasero, E. (2020). VITAL-ECG: a de-
bias algorithm embedded in a gender-immune device. In
2020 IEEE International Workshop on Metrology for In-
dustry 4.0 & IoT, pages 314–318.
Perera, A., Aleti, A., Tantithamthavorn, C., Jiarpakdee,
J., Turhan, B., Kuhn, L., and Walker, K. (2022).
Search-based fairness testing for regression-based ma-
chine learning systems. Empirical Software Engineering,
27(3). Publisher: Springer Type: Article.
Perez Alday, E. A., Rad, A. B., Reyna, M. A., Sadr, N., Gu,
A., Li, Q., Dumitru, M., Xue, J., Albert, D., Sameni, R.,
and Clifford, G. D. (2022). Age, sex and race bias in
automated arrhythmia detectors. Journal of electrocardi-
ology, 74:5–9. Place: United States.
Pessach, D. and Shmueli, E. (2022). A Review on Fair-
ness in Machine Learning. ACM Comput. Surv., 55(3).
Place: New York, NY, USA Publisher: Association for
Computing Machinery.
Pham, T.-H., Yin, C., Mehta, L., Zhang, X., and Zhang,
P. (2023). A fair and interpretable network for clini-
cal risk prediction: a regularized multi-view multi-task
learning approach. Knowledge and information systems,
65(4):1487–1521. Place: England.
Puyol-Ant
´
on, E., Ruijsink, B., Piechnik, S. K., Neubauer,
S., Petersen, S. E., Razavi, R., and King, A. P. (2021).
Fairness in Cardiac MR Image Analysis: An Investi-
gation of Bias Due to Data Imbalance in Deep Learn-
ing Based Segmentation. Lecture Notes in Computer
Science (including subseries Lecture Notes in Artifi-
cial Intelligence and Lecture Notes in Bioinformatics),
12903 LNCS:413 – 423. ISBN: 978-303087198-7 Pub-
lisher: Springer Science and Business Media Deutsch-
land GmbH Type: Conference paper.
Reeves, M., Bhat, H. S., and Goldman-Mellor, S. (2022).
Resampling to address inequities in predictive modeling
of suicide deaths. BMJ health & care informatics, 29(1).
Place: England.
Rueda, J., Rodr
´
ıguez, J. D., Jounou, I. P., Hortal-Carmona,
J., Aus
´
ın, T., and Rodr
´
ıguez-Arias, D. (2022). ”Just” ac-
curacy? Procedural fairness demands explainability in
AI-based medical resource allocations. AI & society,
pages 1–12. Place: Germany.
Sahoo, H. S., Ingraham, N. E., Silverman, G. M., and Sar-
tori, J. M. (2022). Towards Fairness and Interpretabil-
ity: Clinical Decision Support for Acute Coronary Syn-
drome. In 2022 21st IEEE International Conference
on Machine Learning and Applications (ICMLA), pages
882–886.
Shanklin, R., Samorani, M., Harris, S., and Santoro, M. A.
(2022). Ethical Redress of Racial Inequities in AI:
Lessons from Decoupling Machine Learning from Op-
timization in Medical Appointment Scheduling. Philos-
ophy & technology, 35(4):96. Place: Netherlands.
Stanley, E. A. M., Wilms, M., and Forkert, N. D. (2022).
Disproportionate Subgroup Impacts and Other Chal-
lenges of Fairness in Artificial Intelligence for Medical
Image Analysis. Lecture Notes in Computer Science (in-
cluding subseries Lecture Notes in Artificial Intelligence
and Lecture Notes in Bioinformatics), 13755 LNCS:14
– 25. ISBN: 978-303123222-0 Publisher: Springer
Science and Business Media Deutschland GmbH Type:
Conference paper.
Suriyakumar, V. M., Papernot, N., Goldenberg, A., and
Ghassemi, M. (2021). Chasing Your Long Tails: Dif-
ferentially Private Prediction in Health Care Settings. In
Proceedings of the 2021 ACM Conference on Fairness,
Accountability, and Transparency, FAccT ’21, pages
723–734, New York, NY, USA. Association for Com-
puting Machinery. event-place: Virtual Event, Canada.
Wang, Z., Liu, C., and Yao, B. (2022). Multi-Branching
Neural Network for Myocardial Infarction Prediction. In
2022 IEEE 18th International Conference on Automa-
tion Science and Engineering (CASE), pages 2118–2123.
ISSN: 2161-8089.
Yang, J., Soltan, A. A. S., Eyre, D. W., Yang, Y., and
Clifton, D. A. (2023). An adversarial training frame-
work for mitigating algorithmic biases in clinical ma-
chine learning. NPJ digital medicine, 6(1):55. Place:
England.
Zhang, H., Dullerud, N., Seyyed-Kalantari, L., Morris,
Q., Joshi, S., and Ghassemi, M. (2021). An Empirical
Framework for Domain Generalization in Clinical Set-
tings. In Proceedings of the Conference on Health, In-
ference, and Learning, CHIL ’21, pages 279–290, New
York, NY, USA. Association for Computing Machinery.
event-place: Virtual Event, USA.
ICAART 2024 - 16th International Conference on Agents and Artificial Intelligence
822