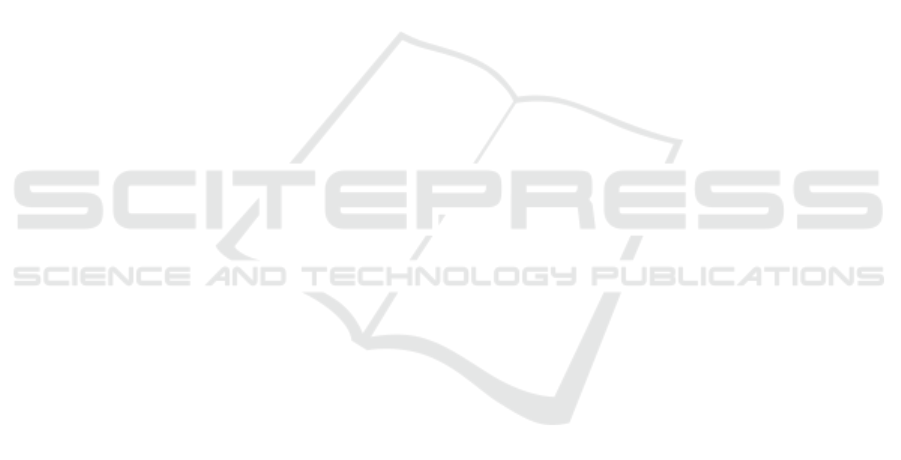
the average results reported by benchmark studies
on indoor or urban scene segmentation. Yet,
there’s still room for improvement of metric values
obtained for railway-specific objects, and results are
mitigated regarding training and inference times.
Finally, the ability of some methods to process
strongly subsampled scans without showing a severe
drop in segmentation performance could meet
industrial expectations in terms of speed for specific
maintenance use cases.
Other than the complexity and size of our dataset,
another possible reason for limited segmentation
performance is that the point clouds captured by
the rotating scanner are relatively sparse. It would
therefore be feasible to test other methods like
SPGraph (Landrieu and Simonovsky, 2018) that is
less affected by distance-dependent sparsity, and
SqueezeSeg (Wu et al., 2018) which exploits the way
the LiDAR captures the data to generate a dense range
image, where each pixel corresponds roughly to a
point in the scan.
Future work also includes completing the dataset
with additional data, compensating for the statistical
class imbalance. Another development to further
evaluate our baselines consists in segmenting more
objects in order to annotate new classes such as
fences, electrical cabinets and traffic lights. Besides,
extending our experiments to classification and object
detection tasks might be a promising direction for
future research.
ACKNOWLEDGEMENTS
This research work contributes to the french
collaborative project TASV (autonomous passenger-
service train), with Railenium, SNCF, Alstom
Crespin, Thales, Bosch and SpirOps. It was carried
out in the framework of IRT Railenium, Valenciennes,
France, and therefore was granted public funds within
the scope of the French Program “Investissements
d’Avenir”.
REFERENCES
Abid, M. and Lefebvre, G. (2021). Improving indoor ge-
omagnetic field fingerprinting using recurrence plot-
based convolutional neural networks. Journal of Lo-
cation Based Services, 15(1):61–87.
Behley, J., Garbade, M., Milioto, A., Quenzel, J., Behnke,
S., Stachniss, C., and Gall, J. (2019). SemanticKITTI:
A dataset for semantic scene understanding of LiDAR
sequences. In Proceedings of the IEEE/CVF inter-
national conference on computer vision, pages 9297–
9307.
Boulch, A. (2020). ConvPoint: Continuous convolutions
for point cloud processing. Computers & Graphics,
88:24–34.
Choy, C., Gwak, J., and Savarese, S. (2019). 4D spatio-
temporal convnets: Minkowski convolutional neural
networks. In Proceedings of the IEEE/CVF con-
ference on computer vision and pattern recognition,
pages 3075–3084.
CloudCompare (2022). (version 2.12.2) [GPL software],
retrieved from http://www.cloudcompare.org/.
Compagnon, P., Lomet, A., Reyboz, M., and Mermillod, M.
(2023). Domestic hot water forecasting for individ-
ual housing with deep learning. In Machine Learning
and Principles and Practice of Knowledge Discovery
in Databases, pages 223–235.
Cordts, M., Omran, M., Ramos, S., Rehfeld, T., Enzweiler,
M., Benenson, R., Franke, U., Roth, S., and Schiele,
B. (2016). The cityscapes dataset for semantic urban
scene understanding. In Proceedings of the IEEE con-
ference on computer vision and pattern recognition,
pages 3213–3223.
Everingham, M., Eslami, S. A., Van Gool, L., Williams,
C. K., Winn, J., and Zisserman, A. (2015). The pascal
visual object classes challenge: A retrospective. In-
ternational journal of computer vision, 111:98–136.
Goyal, P., Doll
´
ar, P., Girshick, R., Noordhuis, P.,
Wesolowski, L., Kyrola, A., Tulloch, A., Jia, Y.,
and He, K. (2017). Accurate, large minibatch
SGD: Training ImageNet in 1 hour. arXiv preprint
arXiv:1706.02677.
Grandio, J., Riveiro, B., Soil
´
an, M., and Arias, P. (2022).
Point cloud semantic segmentation of complex rail-
way environments using deep learning. Automation in
Construction, 141:104425.
Guinard, S. A., Riant, J.-P., Michelin, J.-C., and
Costa D’Aguiar, S. (2021). Fast weakly supervised
detection of railway-related infrastructures in lidar ac-
quisitions. ISPRS Annals of the Photogrammetry, Re-
mote Sensing and Spatial Information Sciences, 2:27–
34.
Guo, Y., Wang, H., Hu, Q., Liu, H., Liu, L., and Ben-
namoun, M. (2021). Deep learning for 3d point
clouds: A survey. IEEE Transactions on Pattern Anal-
ysis and Machine Intelligence, 43(12):4338–4364.
Hackel, T., Savinov, N., Ladicky, L., Wegner, J. D.,
Schindler, K., and Pollefeys, M. (2017). Seman-
tic3d. net: A new large-scale point cloud classification
benchmark. arXiv preprint arXiv:1704.03847.
He, T., Zhang, Z., Zhang, H., Zhang, Z., Xie, J., and Li,
M. (2019). Bag of tricks for image classification with
convolutional neural networks. In Proceedings of the
IEEE/CVF conference on computer vision and pattern
recognition, pages 558–567.
Hu, Q., Yang, B., Xie, L., Rosa, S., Guo, Y., Wang, Z.,
Trigoni, N., and Markham, A. (2020). RandLA-Net:
Efficient semantic segmentation of large-scale point
clouds. In Proceedings of the IEEE/CVF conference
RailCloud-HdF: A Large-Scale Point Cloud Dataset for Railway Scene Semantic Segmentation
169