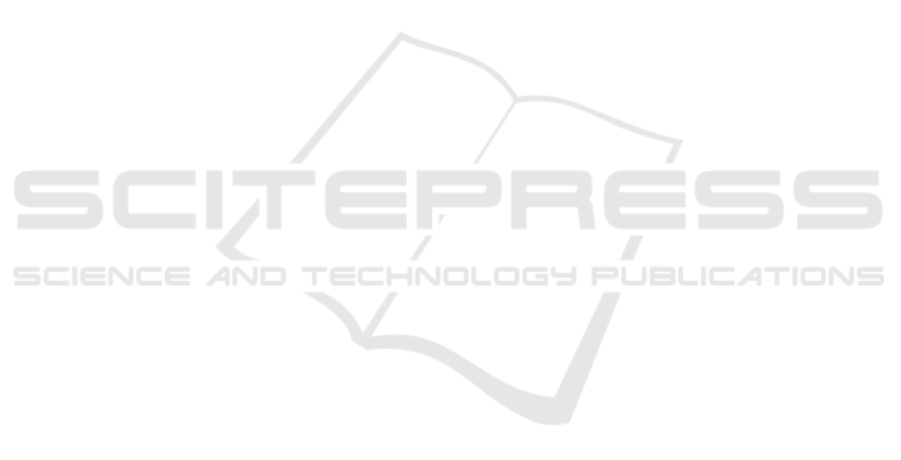
ACKNOWLEDGEMENTS
This research has been supported by the Euro-
pean Commission funded program RESCUER, under
H2020 Grant Agreement 101021836.
REFERENCES
A Handa, V Patraucean, V. B. S. S. R. C. (2016). Under-
standing real world indoor scenes with synthetic data.
In CVPR 2016.
Ancuti, C., Ancuti, C. O., Timofte, R., and
De Vleeschouwer, C. (2018a). I-haze: a dehaz-
ing benchmark with real hazy and haze-free indoor
images. In ACIVS 2018, Proceedings 19. Springer.
Ancuti, C. O., Ancuti, C., Timofte, R., and
De Vleeschouwer, C. (2018b). O-haze: A de-
hazing benchmark with real hazy and haze-free
outdoor images. In CVPR 2018, NTIRE Workshop,
NTIRE CVPR’18, Salt Lake City, Utah, USA.
Ba, Y., Zhang, H., Yang, E., Suzuki, A., Pfahnl, A., Chan-
drappa, C. C., de Melo, C. M., You, S., Soatto, S.,
Wong, A., et al. (2022). Not just streaks: Towards
ground truth for single image deraining. In ECCV.
Butler, D. J., Wulff, J., Stanley, G. B., and Black, M. J.
(2012). A naturalistic open source movie for optical
flow evaluation. In ECCV Proceedings, Part VI 12.
Chen, W.-T., Fang, H.-Y., Ding, J.-J., Tsai, C.-C., and Kuo,
S.-Y. (2020). Jstasr: Joint size and transparency-
aware snow removal algorithm based on modified par-
tial convolution and veiling effect removal. In ECCV
2020 Proceedings, Part XXI 16. Springer.
Chen, W.-T., Fang, H.-Y., Hsieh, C.-L., Tsai, C.-C., Chen,
I., Ding, J.-J., Kuo, S.-Y., et al. (2021). All snow re-
moved: Single image desnowing algorithm using hier-
archical dual-tree complex wavelet representation and
contradict channel loss. In CVPR 2021.
Chen, W.-T., Huang, Z.-K., Tsai, C.-C., Yang, H.-H., Ding,
J.-J., and Kuo, S.-Y. (2022). Learning multiple ad-
verse weather removal via two-stage knowledge learn-
ing and multi-contrastive regularization: Toward a
unified model. In CVPR 2022.
Fu, X., Huang, J., Zeng, D., Huang, Y., Ding, X., and Pais-
ley, J. (2017). Removing rain from single images via
a deep detail network. In CVPR 2017.
Karavarsamis, S., Doumanoglou, A., Konstantoudakis, K.,
and Zarpalas, D. (2022a). Cross-stitched multi-task
dual recursive networks for unified single image de-
raining and desnowing. In WF-IoT 2022.
Karavarsamis, S., Gkika, I., Gkitsas, V., Konstantoudakis,
K., and Zarpalas, D. (2022b). A survey of deep
learning-based image restoration methods for enhanc-
ing situational awareness at disaster sites: the cases of
rain, snow and haze. Sensors, 22(13).
Li, B., Liu, X., Hu, P., Wu, Z., Lv, J., and Peng, X. (2022).
All-in-one image restoration for unknown corruption.
In CVPR 2022.
Li, B., Ren, W., Fu, D., Tao, D., Feng, D., Zeng, W., and
Wang, Z. (2018). Benchmarking single-image dehaz-
ing and beyond. IEEE Transactions on Image Pro-
cessing, 28(1).
Li, R., Cheong, L.-F., and Tan, R. T. (2019). Heavy rain
image restoration: Integrating physics model and con-
ditional adversarial learning. In CVPR 2019.
Li, R., Tan, R. T., and Cheong, L.-F. (2020). All in one bad
weather removal using architectural search. In CVPR.
Liu, P., Zhou, X., Yang, J., El Basha Mohammad, D., and
Fang, R. (2019). Image restoration using deep regu-
lated convolutional networks.
Liu, Y.-F., Jaw, D.-W., Huang, S.-C., and Hwang, J.-N.
(2018). Desnownet: Context-aware deep network for
snow removal. IEEE Trans. Image Processing, 27(6).
Mayer, N., Ilg, E., Hausser, P., Fischer, P., Cremers, D.,
Dosovitskiy, A., and Brox, T. (2016). A large dataset
to train convolutional networks for disparity, optical
flow, and scene flow estimation. In CVPR 2016.
Paszke, A., Gross, S., Massa, F., Lerer, A., Bradbury, J.,
Chanan, G., Killeen, T., Lin, Z., Gimelshein, N.,
Antiga, L., Desmaison, A., Kopf, A., Yang, E., De-
Vito, Z., Raison, M., Tejani, A., Chilamkurthy, S.,
Steiner, B., Fang, L., Bai, J., and Chintala, S. (2019).
PyTorch: An Imperative Style, High-Performance
Deep Learning Library. In Advances in Neural Infor-
mation Processing Systems 32.
Qian, R., Tan, R. T., Yang, W., Su, J., and Liu, J. (2018).
Attentive generative adversarial network for raindrop
removal from a single image. In CVPR 2018.
Ren, Y., Li, S., Nie, M., and Chuankun, L. (2020). Single
image de-raining via improved generative adversarial
nets. Sensors, 20.
Richter, S. R., Vineet, V., Roth, S., and Koltun, V. (2016).
Playing for data: Ground truth from computer games.
In ECCV 2016 Proceedings, Part II 14. Springer.
Valanarasu, J. M. J., Yasarla, R., and Patel, V. M. (2022).
Transweather: Transformer-based restoration of im-
ages degraded by adverse weather conditions. In
CVPR 2022.
Wang, T., Zhang, K., Shao, Z., Luo, W., Stenger, B., Lu, T.,
Kim, T.-K., Liu, W., and Li, H. (2023). Gridformer:
Residual dense transformer with grid structure for im-
age restoration in adverse weather conditions. arXiv
preprint arXiv:2305.17863.
Yang, W., Tan, R. T., Wang, S., Fang, Y., and Liu, J. (2019).
Single image deraining: From model-based to data-
driven and beyond.
Yang, W., Tan, R. T., Wang, S., Fang, Y., and Liu, J. (2020).
Single image deraining: From model-based to data-
driven and beyond. PAMI 2020, 43(11).
Yao, M., Xu, R., Guan, Y., Huang, J., and Xiong, Z. (2023).
Neural degradation representation learning for all-in-
one image restoration.
Zhang, H. and Patel, V. M. (2018). Density-aware single
image de-raining using a multi-stream dense network.
In CVPR 2018.
¨
Ozdenizci, O. and Legenstein, R. (2022). Restoring vi-
sion in adverse weather conditions with patch-based
denoising diffusion models.
VISAPP 2024 - 19th International Conference on Computer Vision Theory and Applications
574