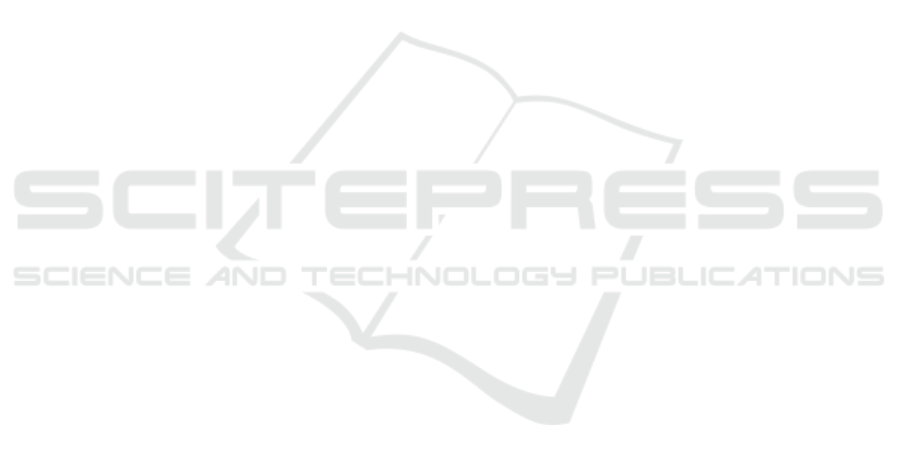
in segmentation might yield more pronounced results
in future investigations. We assume that having a
diverse array of segments for each object category,
without pronounced imbalances, is crucial. As ob-
served in Table 6, segmentation didn’t enhance the
outcomes for tables. This can be linked to the distri-
bution where over 96% of the table points are associ-
ated with two classes, and fewer than 4% with a third
class. This distribution is detailed in Table 5.
5 CONCLUSIONS
This study presented the line of models for point
cloud completion by accurately incorporating a view-
guided approach and segmentation. This method uti-
lizes images and incomplete point clouds to address
the task. The ImgAdaPoinTr performs better for all
classes of 3D objects considered in comparison with
baselines. The best results were received by ImgEnc-
SegDecAPTr, which is enchanced by fusion of im-
age features and segmentation simultaneously. We
also introduce the ImgPCN dataset, generated via our
open-source rendering tool, which provides a new re-
source for evaluating point cloud completion tech-
niques. Due to the revealed limitations of existing
pre-trained segmentation models, we plan to widen
ImgPCN with segmentation markdown and set up a
precise experiment for fusing.
REFERENCES
Abbasi, R., Bashir, A. K., Alyamani, H. J., Amin, F., Doh,
J., and Chen, J. (2022). Lidar point cloud compres-
sion, processing and learning for autonomous driving.
IEEE Transactions on Intelligent Transportation Sys-
tems, 24(1):962–979.
Aiello, E., Valsesia, D., and Magli, E. (2022). Cross-modal
learning for image-guided point cloud shape comple-
tion. Advances in Neural Information Processing Sys-
tems, 35:37349–37362.
Berger, M., Tagliasacchi, A., Seversky, L., Alliez, P., Guen-
nebaud, G., Levine, J., Sharf, A., and Silva, C.
(2016). A Survey of Surface Reconstruction from
Point Clouds. Computer Graphics Forum, page 27.
Bi, S., Yuan, C., Liu, C., Cheng, J., Wang, W., and Cai, Y.
(2021). A survey of low-cost 3d laser scanning tech-
nology. Applied Sciences, 11(9).
Dai, A., Ruizhongtai Qi, C., and Nießner, M. (2017).
Shape completion using 3d-encoder-predictor cnns
and shape synthesis. In Proceedings of the IEEE con-
ference on computer vision and pattern recognition,
pages 5868–5877.
Han, X., Li, Z., Huang, H., Kalogerakis, E., and Yu, Y.
(2017). High-resolution shape completion using deep
neural networks for global structure and local geom-
etry inference. In Proceedings of the IEEE interna-
tional conference on computer vision, pages 85–93.
Johnson, J., Ravi, N., Reizenstein, J., Novotny, D., Tulsiani,
S., Lassner, C., and Branson, S. (2020). Accelerating
3d deep learning with pytorch3d. In SIGGRAPH Asia
2020 Courses, SA ’20, New York, NY, USA. Associ-
ation for Computing Machinery.
Li, J., Zhang, J., Wang, Z., Shen, S., Wen, C., Ma, Y., Xu,
L., Yu, J., and Wang, C. (2022). Lidarcap: Long-
range markerless 3d human motion capture with lidar
point clouds. In 2022 IEEE/CVF Conference on Com-
puter Vision and Pattern Recognition (CVPR), pages
20470–20480, Los Alamitos, CA, USA. IEEE Com-
puter Society.
Li, R., Li, X., Fu, C.-W., Cohen-Or, D., and Heng, P.-A.
(2019). Pu-gan: a point cloud upsampling adversar-
ial network. In Proceedings of the IEEE/CVF inter-
national conference on computer vision, pages 7203–
7212.
Liu, Y., Fan, B., Xiang, S., and Pan, C. (2019a). Relation-
shape convolutional neural network for point cloud
analysis. In Proceedings of the IEEE/CVF conference
on computer vision and pattern recognition, pages
8895–8904.
Liu, Z., Tang, H., Lin, Y., and Han, S. (2019b). Point-voxel
cnn for efficient 3d deep learning. Advances in Neural
Information Processing Systems, 32.
Nichol, A., Jun, H., Dhariwal, P., Mishkin, P., and Chen,
M. (2022). Point-e: A system for generating 3d
point clouds from complex prompts. arXiv preprint
arXiv:2212.08751.
Poole, B., Jain, A., Barron, J. T., and Mildenhall, B. (2022).
Dreamfusion: Text-to-3d using 2d diffusion. arXiv
preprint arXiv:2209.14988.
Qi, C. R., Su, H., Mo, K., and Guibas, L. J. (2017a). Point-
net: Deep learning on point sets for 3d classification
and segmentation. In Proceedings of the IEEE con-
ference on computer vision and pattern recognition,
pages 652–660.
Qi, C. R., Yi, L., Su, H., and Guibas, L. J. (2017b). Point-
net++: Deep hierarchical feature learning on point sets
in a metric space. Advances in neural information pro-
cessing systems, 30.
Radford, A., Kim, J. W., Hallacy, C., Ramesh, A., Goh, G.,
Agarwal, S., Sastry, G., Askell, A., Mishkin, P., Clark,
J., et al. (2021). Learning transferable visual models
from natural language supervision. In International
conference on machine learning, pages 8748–8763.
PMLR.
Raj, T., Hashim, F. H., Huddin, A. B., Ibrahim, M. F., and
Hussain, A. (2020). A survey on lidar scanning mech-
anisms. Electronics, 9(5).
Ramesh, A., Pavlov, M., Goh, G., Gray, S., Voss, C., Rad-
ford, A., Chen, M., and Sutskever, I. (2021). Zero-shot
text-to-image generation. In International Conference
on Machine Learning, pages 8821–8831. PMLR.
Reimers, N. and Gurevych, I. (2019). Sentence-BERT: Sen-
tence embeddings using Siamese BERT-networks. In
VISAPP 2024 - 19th International Conference on Computer Vision Theory and Applications
56