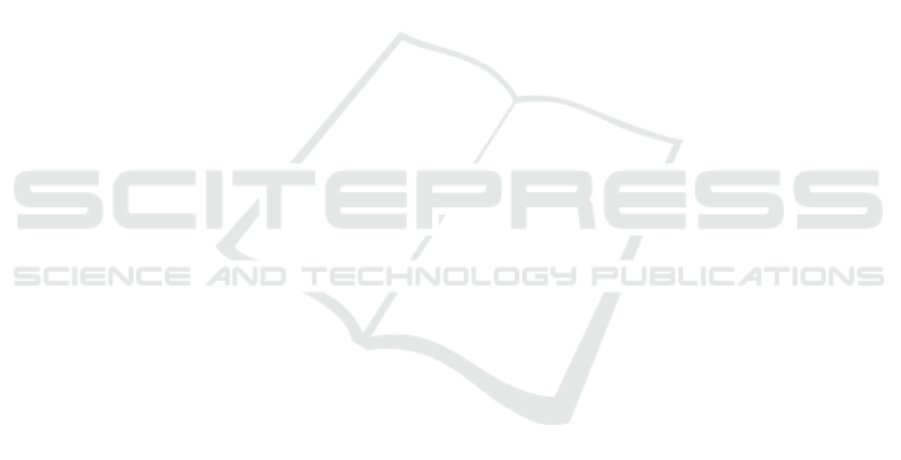
sion probabilistic models. In International Conference
on Neural Information Processing Systems (NeurIPS),
volume 33, pages 6840–6851.
Ho, J. and Salimans, T. (2022). Classifier-free diffusion
guidance. arXiv preprint arXiv:2207.12598.
Huang, G., Liu, Z., Van Der Maaten, L., and Wein-
berger, K. Q. (2017). Densely connected convolu-
tional networks. In Proceedings of the IEEE con-
ference on Computer Vision and Pattern Recognition,
pages 4700–4708.
Jolicoeur-Martineau, A., Li, K., Pich
´
e-Taillefer, R., Kach-
man, T., and Mitliagkas, I. (2021). Gotta go fast
when generating data with score-based models. arXiv
preprint arXiv:2105.14080.
Karras, T., Laine, S., and Aila, T. (2019). A style-based
generator architecture for generative adversarial net-
works. In IEEE/CVF Conference on Computer Vision
and Pattern Recognition (CVPR), pages 4396–4405.
Kim, H., Kim, S., and Yoon, S. (2022a). Guided-tts: A
diffusion model for text-to-speech via classifier guid-
ance. In International Conference on Machine Learn-
ing, pages 11119–11133. PMLR.
Kim, M., Jain, A. K., and Liu, X. (2022b). Adaface: Quality
adaptive margin for face recognition. In Proceedings
of the IEEE/CVF Conference on Computer Vision and
Pattern Recognition.
Kloeden, P. and Platen, E. (2011). The Numerical Solu-
tion of Stochastic Differential Equations, volume 23.
Springer.
Lee, C.-H., Zhang, K., Lee, H.-C., Cheng, C.-W., and Hsu,
W. (2018). Attribute augmented convolutional neu-
ral network for face hallucination. In Proceedings of
the IEEE conference on Computer Vision and Pattern
Recognition workshops, pages 721–729.
Li, H., Yang, Y., Chang, M., Chen, S., Feng, H., Xu, Z.,
Li, Q., and Chen, Y. (2022). SRDiff: Single image
super-resolution with diffusion probabilistic models.
Neurocomputing, 479:47–59.
Li, M., Zhang, Z., Yu, J., and Chen, C. W. (2020). Learn-
ing face image super-resolution through facial seman-
tic attribute transformation and self-attentive struc-
ture enhancement. IEEE Transactions on Multimedia,
23:468–483.
Li, X., Ren, Y., Jin, X., Lan, C., Wang, X., Zeng, W.,
Wang, X., and Chen, Z. (2023). Diffusion models for
image restoration and enhancement–a comprehensive
survey. arXiv preprint arXiv:2308.09388.
Liu, Z., Luo, P., Wang, X., and Tang, X. (2015). Deep learn-
ing face attributes in the wild. In Proceedings of In-
ternational Conference on Computer Vision (ICCV).
Lu, Y., Tai, Y.-W., and Tang, C.-K. (2018). Attribute-guided
face generation using conditional cyclegan. In Pro-
ceedings of the European Conference on Computer
Vision (ECCV), pages 282–297.
Meng, C., Rombach, R., Gao, R., Kingma, D., Ermon,
S., Ho, J., and Salimans, T. (2023). On distillation
of guided diffusion models. In Proceedings of the
IEEE/CVF Conference on Computer Vision and Pat-
tern Recognition, pages 14297–14306.
Neves, J., Moreno, J., and Proenc¸a, H. (2018). QUIS-
CAMPI: an annotated multi-biometrics data feed from
surveillance scenarios. IET Biometrics, 7(4):371–379.
Nichol, A. and Dhariwal, P. (2021). Improved denois-
ing diffusion probabilistic models. arXiv preprint
arXiv:2102.09672.
Niu, C. et al. (2020). Permutation invariant graph genera-
tion via score-based generative modeling. In Interna-
tional Conference on Artificial Intelligence and Statis-
tics (AISTATS), volume 108, pages 4474–4484.
Saharia, C., Chan, W., Saxena, S., Li, L., Whang, J.,
Denton, E. L., Ghasemipour, K., Gontijo Lopes, R.,
Karagol Ayan, B., Salimans, T., et al. (2022). Photo-
realistic text-to-image diffusion models with deep lan-
guage understanding. Advances in Neural Information
Processing Systems, 35:36479–36494.
Saharia, C., Ho, J., Chan, W., Salimans, T., Fleet, D. J., and
Norouzi, M. (2021). Image super-resolution via itera-
tive refinement. arXiv preprint, arXiv:2104.07636:1–
28. Google Research.
Santos, M. D., Laroca, R., Ribeiro, R. O., Neves, J.,
Proenc¸a, H., and Menotti, D. (2022). Face super-
resolution using stochastic differential equations. In
2022 35th SIBGRAPI Conference on Graphics, Pat-
terns and Images (SIBGRAPI), volume 1, pages 216–
221. IEEE.
S
¨
arkk
¨
a, S. and Solin, A. (2019). Applied stochastic differ-
ential equations, volume 10. Cambridge University
Press.
Sohl-Dickstein, J., Weiss, E., Maheswaranathan, N., and
Ganguli, S. (2015). Deep unsupervised learning us-
ing nonequilibrium thermodynamics. In International
Conference on Machine Learning, pages 2256–2265.
Song, J., Meng, C., and Ermon, S. (2020). De-
noising diffusion implicit models. arXiv preprint
arXiv:2010.02502.
Song, Y. and Ermon, S. (2019). Generative modeling by es-
timating gradients of the data distribution. In Interna-
tional Conference on Neural Information Processing
Systems (NeurIPS), pages 1–13.
Song, Y. et al. (2021). Score-based generative model-
ing through stochastic differential equations. In In-
ternational Conference on Learning Representations
(ICLR), pages 1–36.
Vahdat, A., Kreis, K., and Kautz, J. (2021). Score-based
generative modeling in latent space. In International
Conference on Neural Information Processing Sys-
tems (NeurIPS), volume 34, pages 11287–11302.
Vincent, P. (2011). A connection between score match-
ing and denoising autoencoders. Neural computation,
23(7):1661–1674.
Yang, L., Zhang, Z., Song, Y., Hong, S., Xu, R., Zhao,
Y., Shao, Y., Zhang, W., Cui, B., and Yang, M.-H.
(2022). Diffusion models: A comprehensive sur-
vey of methods and applications. arXiv preprint
arXiv:2209.00796.
Yi, D., Lei, Z., Liao, S., and Li, S. Z. (2014). Learn-
ing face representation from scratch. arXiv preprint
arXiv:1411.7923.
Defying Limits: Super-Resolution Refinement with Diffusion Guidance
433